Nafea Bshara, Amazon Web Services vice president and distinguished engineer, and the co-founder of Annapurna Labs, an Israeli-based chipmaker that Amazon acquired in 2015, maintains a low profile, as does his friend and Annapurna co-founder, Hrvoye (Billy) Bilic.
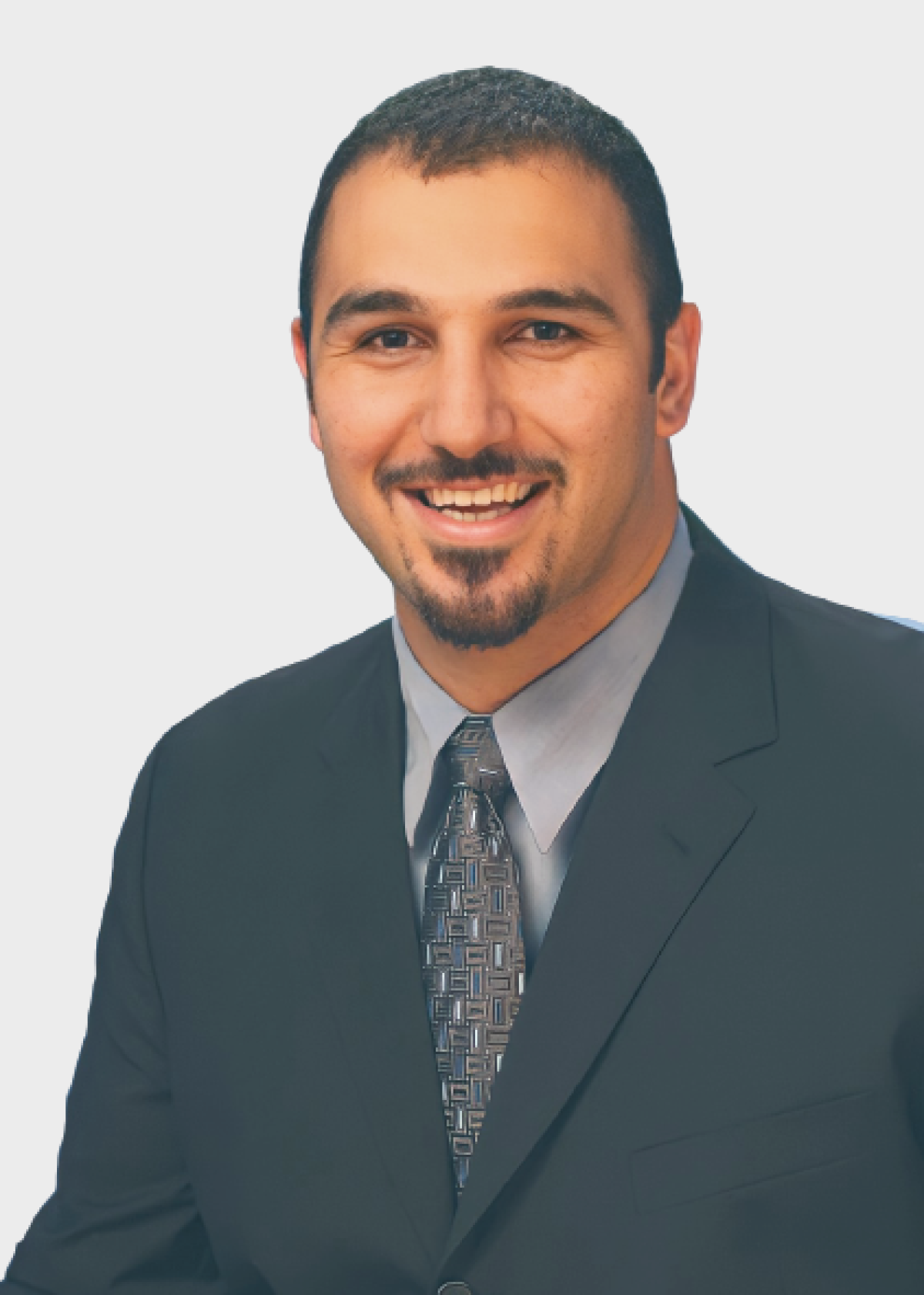
Each executive’s LinkedIn profile is sparse, in fact, Bilic’s is out of date.
“We hardly do any interviews; our philosophy is to let our products do the talking,” explains Bshara.
Those products, and silicon innovations, have done a lot of talking since 2015, as the acquisition has led to, among other advancements, the development of five generations of the AWS Nitro System, three generations (1, 2, 3) of custom-designed, Arm-based Graviton processors that support data-intensive workloads, as well as AWS Trainium, and AWS Inferentia chips optimized for machine learning training and inference.
Some observers have described the silicon that emerges from Annapurna Labs in the U.S. and Israel as AWS’s “secret sauce”.
Nafea’s silicon journey began at Technion University in Israel, where he earned bachelor’s and master’s degrees in computer engineering, and where he first met Hrvoye. The two then went on to work for Israel-based Galileo, a company that made chips for networking switches, and controllers for networking routers. Galileo was acquired by U.S. semiconductor manufacturer Marvell in 2000, where Bshara and Bilic would work for a decade before deciding to venture out on their own.
“We had developed at least 50 different chips together,” Bshara explained, “so we had a track record and a first-hand understanding of customer needs, and the market dynamics. We could see that some market segments were being underserved, and with the support from our spouses, Lana and Liat, and our funding friends Avigdor [Willenz] and Manuel [Alba], we started Annapurna Labs.”
That was mid-2011, and three and half years later Amazon acquired the company. The two friends have continued their journey at Amazon, where their team’s work has spoken for itself.
Last year, industry analyst David Vellante praised AWS’s “revolution in system architecture.”
“Much in the same way that AWS defined the cloud operating model last decade, we believe it is once again leading in future systems. The secret sauce underpinning these innovations is specialized designs… We believe these moves position AWS to accommodate a diversity of workloads that span cloud, data center as well as the near and far edge.”
Annapurna’s work was highlighted during the AWS Silicon Innovation Day virtual event on August 3. In fact, Nafea was a featured speaker in the event. The Silicon Innovation Day broadcast, which highlighted AWS silicon innovations, included a keynote from David Brown, vice president, Amazon EC2; a talk about the history of AWS silicon innovation from James Hamilton, Amazon senior vice president and distinguished engineer who holds more than 200 patents in 22 countries in server and datacenter infrastructure, database, and cloud computing; and a fireside chat on the Nitro System with Anthony Liguori, AWS vice president and distinguished engineer, and Jeff Barr, AWS vice president and chief evangelist.
In advance of the silicon-innovation event, Amazon Science connected with Bshara to discuss the history of Annapurna, how the company and the industry have evolved in the past decade, and what the future portends.
- Q.
You co-founded Annapurna Labs just over 11 years ago. Why Annapurna?
A.I co-founded the company with my longtime partner, Billy, and with an amazing set of engineers and leaders who believed in the mission. We started Annapurna Labs because we looked at the way the chip industry was investing in infrastructure and data centers; it was minuscule at that time because everybody was going after the gold rush of mobile phones, smartphones, and tablets.
We believed the industry was over indexing on investment for mobile, and under investing in the data center. The data center market was underserved. That, combined with the fact that there was increasing disappointment with the ineffective and non-productive method of developing chips, especially when compared with software development. The productivity of software developers had improved significantly in the past 25 years, while the productivity of chip developers hadn’t improved much since the ‘90s. In assessing the opportunity, we saw a data-center market that was being underserved, and an opportunity to redefine chip development with greater productivity, and with a better business model. Those factors contributed to us starting Annapurna Labs.
- Q.
How has the chip industry evolved in the past 11 years?
A.The chip industry realized, a bit late, but nevertheless realized that productivity and time to market needed to be addressed. While Annapurna has been a pioneer in advancing productivity and time to market, many others are following in our footsteps and transitioning to a building-blocks-centric development mindset, similar to how the software industry moved toward object-oriented, and service-oriented software design.
Chip companies have now transitioned to what we refer to as an intellectual property-oriented, or IP-oriented, correct-by-design approach. Secondly, the chip industry has adopted the cloud. Cloud adoption has led to an explosion of compute power for building chips. Using the cloud, we are able to use compute in a ‘bursty’ way and in parallel. We and our chip-industry colleagues couldn’t deliver the silicon we do today without the cloud. This has led to the creation of a healthy market where chip companies have realized they don’t need to build everything in house, in much the same way software companies have realized they can buy libraries from open source or other library providers. The industry has matured to the point where now there is a healthy business model around buying building blocks, or IPs, from providers like Arm, Synopsys, Alphawave, or Cadence.
- Q.
Annapurna Labs was named after one of the tallest peaks in the Himalayas that’s regarded as one of the most dangerous mountains to climb. What's been the tallest peak you've had to climb?
A.I’m up in the cloud, I don’t need to climb anything [laughing]. Yes, Billy and I picked the name Annapurna Labs for a couple of reasons. First, Billy and I originally planned to climb Annapurna before we started the company. But then we got excited about the idea, acquired funding, and suddenly time was of the essence, so we put our climbing plans on hold and started the company. We called it Annapurna because at that time – and it’s true even today – there is a high barrier to entry in starting a chip company. The challenge is steep, and the risk is high, so it’s just like climbing Annapurna. We also believed that we wanted to reach a point above the clouds where you could see things very clearly, and without clutter. That’s always been a mantra for us as a company: Avoid the clutter, and look far into the future to understand what the customer really needs versus getting distracted by the day-to-day noise.
- Q.
What are the unique challenges you face in designing chips for ML training and inference versus more general CPU designs?
A.First, I would want to emphasize what challenge we didn’t have to worry about: with the strong foundation, methodologies, and engineering muscle we built delivering multiple generations of Nitro, we had confidence in our ability to execute on building the chips and manufacturing them at high volume, and high quality. So that was a major thing we didn’t need to worry about. Designing for machine learning is one the most challenging, but also the most rewarding tasks I've had the pleasure to participate in. There is an insatiable demand for machine learning right now, so anyone with a good product won’t have any issues finding customer demand. The demand is there, but there are a couple of challenges.
The first is that customers want ‘just works’ solutions because they have enough challenges to work on the science side. So they are looking for a frictionless migration from the incumbent, let's say GPU-based machine learning, to AWS Trainium or AWS Inferentia. Our biggest challenge is to hide all the complexity so it’s what we refer to internally as boring to migrate. We don’t want our customers, the scientists and researchers, to have to think about moving from one piece of hardware to another. This is a challenge because the incumbent GPUs, specifically NVIDIA, have done a very good job developing broadly adopted technologies. The customer shouldn’t see or experience any of the hard work we’ve done in developing our chips; what the customer should experience is that it’s transparent and frictionless to transition to Inferentia and Trainium. That’s a hefty task and one of our internal challenges as a team.
"The customer shouldn’t see or experience any of the hard work we’ve done in developing our chips; what the customer should experience is that it’s transparent and frictionless to transition to Inferentia and Trainium," says Bshara. The second challenge is more external; it’s the fact that science and machine learning are moving very fast. As an organization that is building hardware, our job is to predict what customers will need three, four, five years down the road because the development cycle for a chip can be two years, and then it gets deployed for three years. The lifecycle is around five years and trying to predict how the needs of scientists and the machine-learning community will evolve over that time span is difficult. Unlike CPU workloads, which aren’t evolving very quickly, machine learning workloads are, and it’s a bit of an art to keep apace. I would give ourselves a high score, not a perfect score, in being efficient in terms of execution and cost, while still being future proof. It’s the art of predicting what customers will need three years from now, while still executing on time and budget. These things only come with experience, and I’m fortunate to be part of a great team that has the experience to strike the right balance between cost, schedule, and future-proofing the product.
- Q.
At the recent re:MARS conference Rohit Prasad, Amazon senior vice president and Alexa head scientist, said the voice assistant is interacting with customers billions of times each week. Alexa is powered by EC2 Inf1 instances, which use AWS Inferentia chips. Why is it more effective for Alexa workloads to take advantage of this kind of specialized processing versus more general-purpose GPUs?
A.Alexa is one of those Amazon technologies that we want to bring to as many people as possible. It’s also a great example of the Amazon flywheel; the more people use it, the more value it delivers. One of our goals is to provide this service with as low latency as possible, and at the lowest cost possible, and over time improve the machine-learning algorithms behind Alexa. When people say improving Alexa, it really means handling much more complex machine learning, much more sophisticated models while maintaining the performance, and low latency. Using Inferentia, the chip, and Inf1, the EC2 instances that actually hosts all of these chips, Alexa is able to run much more advanced machine learning algorithms at lower costs and with lower latency than a standard general-purpose chip. It's not that the general-purpose chip couldn't do the job, it's that it would do so at higher costs and higher latency. With Inferentia we deliver lower latency and support much more sophisticated algorithms. This results in customers having a better experience with Alexa, and benefitting from a smarter Alexa.
- Q.
AI has been called the new electricity. But as ML models become increasingly large and complex as you just discussed, there also are concerns that energy consumption for AI model training and inference is damaging to the environment. At the chip level, what can be done to reduce the environmental impact of ML model training and Inference?
A.What we can do at the chip level, at the EC2 level, is actually work on three vectors, which we’re doing right now. The first is drive to lower power quickly by using more advanced silicon processes. Every time we build a chip in an advanced silicon process we're utilizing new semiconductor processes with smaller transistors that require less power for the same work. Because of our focus on efficient execution, we can deliver to EC2 customers a new chip based on a more modern, power-efficient silicon process every 18 months or so.
The second vector is building more technologies, trying to accelerate in hardware and in algorithms, to get training and inference done faster. The faster we can handle training and inference, the less power is consumed. For example, one of the technologies we innovated in the last Trainium chip was something called stochastic rounding which, depending upon which measure you're looking at for some neural workloads, could accelerate neural network training by up to 30%. When you say 30% less time that translates into 30% less power.
Another thing we're doing at the algorithmic level is offering different data types. For example, historically machine learning used a 32-bit floating point. Now we’re offering multiple versions of 16-bit and a few versions of 8-bit. When these different data types are used, they not only accelerate machine learning training, they significantly reduce the power for the same amount of workload. For example, doing matrix multiplication on a 16-bit float point is less than one-third the total power if we had done it with 32-bit floating point. The ability to add things like stochastic rounding or new data types at the algorithmic level provides a step-function improvement in power consumption for the same amount of workload.
The third vector is credit to EC2 and the Nitro System, we’re offering more choice for customers. There are different chips optimized for different workloads, and the best way for customers to save energy is to follow the classic Amazon mantra – the everything store. We offer all different types of chips, including multiple generations of Nvidia GPUs, Intel Habana, and Trainium, and share with the customer the power profile and performance of each of the instances hosting these chips, so the customer can choose the right chip for the right workload, and optimize for the lowest possible power consumption at the lowest cost.
- Q.
I’ve focused primarily on machine learning. But let’s turn our attention to more general-purpose workloads running in the cloud, and your work on Graviton processors for Amazon EC2.
A.Yes, in a way Graviton is the opposite of our work on machine learning, in the sense that the focus is on building server processors for general-purpose workloads running in EC2. The market for general-purpose chips has been there for thirty or forty years, and the workloads themselves haven’t evolved as rapidly as machine learning, so when we started designing, the target was clear to us.
AWS is three generations into its Graviton chip journey, and Bshara says the company has plans for "many more generations" to come. Because this segment of the industry wasn’t moving that fast, we felt our challenge was to move the industry faster, specifically in offering step function improvement in performance, and reducing costs, and power consumption. There are many times when you build plans, especially for chips, where the original plans are rosy, but as the development progresses you have to make tradeoffs, and the actual product falls short of the original promise. With first-generation Graviton, we experienced the opposite; we were pleasantly surprised that both performance and power efficiency turned out better than our original plan. That’s very rare in our industry.
The same has been true with Graviton2. Because of this there has been a massive movement inside Amazon for general workloads to move to Graviton2, mainly to save on power, but also on costs. For the same workloads, Graviton2 will on average consume 60% less power than same-generation competitive offerings, and we’re passing on those cost-savings to customers. Outside Amazon, at least 48 of AWS’s top 50 customers have not just tested, but have production workloads running on Graviton2.
In May, Graviton3 processors became available, so it’s still Day 1 as we’re only three generations into this journey. We have plans for many more generations, but it’s always very satisfying and rewarding to hear how boring it is for customers to migrate to Graviton, and to hear all the customer success stories. It is incredibly satisfying to come to work every day and hear some of the success stories from the tens of thousands of customers using Graviton.
- Q.
You have more than 100 openings on your jobs page. What kind of talent are you seeking? And what are the characteristics of employees who succeed at Annapurna Labs?
A.We are seeking individuals who like to work on cutting-edge technology, and approach challenges from a principles-first approach because most of the challenges we confront haven’t been dealt with before. While actual experience is important, we place greater value on proper thinking and a principles-first mindset, or reasoning from first principles.
We also value individuals who enjoy working in a dynamic environment where the solution isn’t always the same hammer after the same nail. Given our principles-first approach, many of our challenges get solved at the chip level, the terminal level, and the system level, so we seek individuals who have systems understanding, and are skilled at working across disciplines. It’s difficult for an individual with a single discipline, or single domain knowledge, who isn’t willing to challenge her or himself by learning across other domains, to succeed at Annapurna. Last but not least, we look for individuals who focus on delivering, within a team environment. We recognize ideas are “cheap”, and what makes the difference is delivering on the idea all the way to production. Ideas are a commodity. Executing on those ideas is not.
- Q.
I've read that Billy and you share the belief that if you can dream it, you can do it. So what's your dream about future silicon development?
A.That’s true, and it’s the main reason Billy and I wanted to join AWS, because we had a common vision that there’s so much value we can bring to customers, and AWS leadership and Amazon in general were willing to invest in that vision for the long term. We agreed to be acquired by Amazon not only because of the funding and our common long-term vision, but also because building components for our own data centers would allow us to quickly deliver customer value. We’ve been super happy with the relationship for many reasons, but primarily because of our ability to have customer impact at global scale.
At Amazon, we operate at such a scale and with such a diversity of customers that we are capable of doing application-specific, or domain-specific acceleration. Machine learning is one example of that. What we’ve done with Aqua (advanced query accelerator) for Amazon Redshift is another example where we’ve delivered hardware-based acceleration for analytics. Our biggest challenge these days is deciding what project to prioritize. There’s no shortage of opportunities to deliver value. The only way we’re able to take this approach is because of AWS. Developing silicon requires significant investment, and the only way to gain a good return on that investment is by having a lot of volume and cost-effective development, and we’ve been able to develop a large, and successful customer base with AWS.
I should also add that before joining Amazon we thought we really took a long-term perspective. But once you sit in Amazon meetings, you realize what long-term strategic thinking really means. I continue to learn every day about how to master that. Suffice to say, we have a product roadmap, and a technology and investment strategy that extends to 2032. As much uncertainty as there is in the future, there are a few things we’re highly convicted in, and we’re investing in them, even though they may be ten years out. I obviously can’t disclose future product plans, but we continue to dream big on behalf of our customers.
The AWS Annapurna Labs team has more than 100 job openings for software developers, physical design engineers, design specification engineers, and many other technical roles. The team has development centers in the U.S. and Israel.