The past year saw an acceleration of the recent trend toward research on fairness and privacy in machine learning. The Alexa Trustworthy AI team was part of that, organizing the Trustworthy Natural Language Processing workshop (TrustNLP 2022) at the meeting of the North American chapter of the Association for Computational Linguistics (NAACL) and a special session at Interspeech 2022 titled Trustworthy Speech Processing. Complementing our own research, our organizational work has the aim of building the community around this important research area.
TrustNLP keynotes
This year was the second iteration of the TrustNLP workshop, with contributed papers, keynote presentations from leading experts, and a panel discussion with a diverse cohort of panelists.
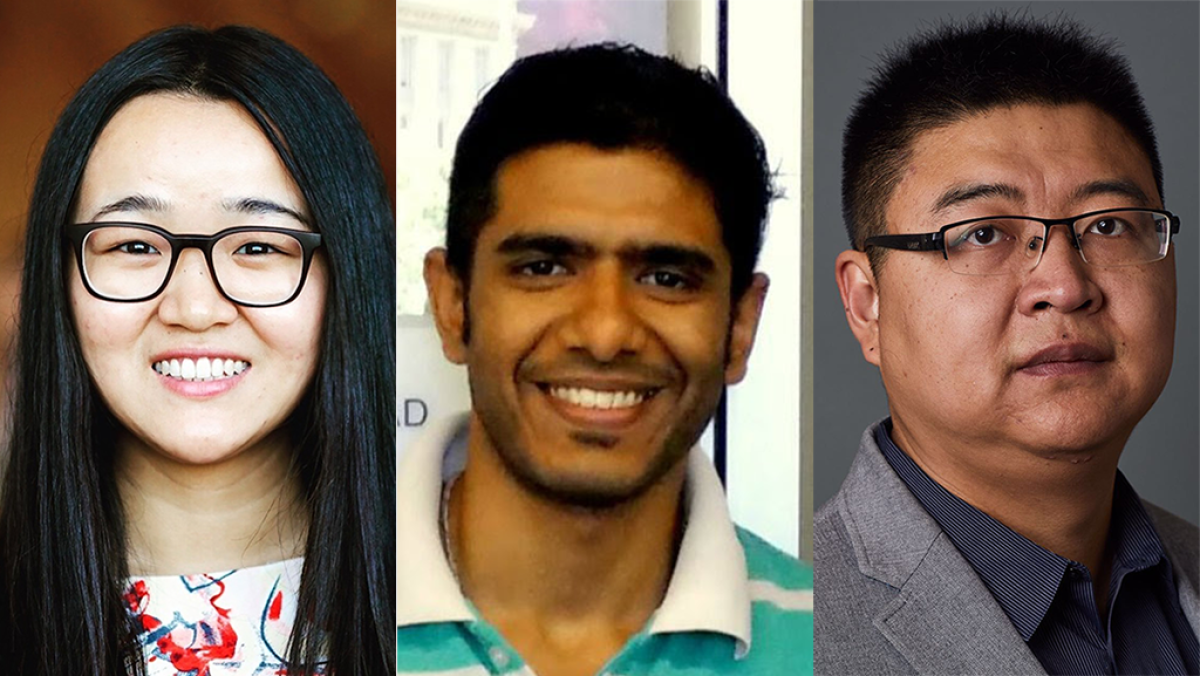
The morning session kicked off with a keynote address by Subho Majumdar of Splunk, on interpretable graph-based mapping of trustworthy machine learning research, which provides a framework for estimating the fairness risks of machine learning (ML) applications in industry. The Splunk researchers scraped papers from previous ML conferences and used the resulting data to build a word co-occurrence matrix to detect interesting communities in this network.
They found that terms related to trustworthy ML separated out into two well-formed communities, one centered on privacy issues and the other on demography and fairness-related problems. Majumdar also suggested that such information could be leveraged to quantitatively assess fairness-related risks for different research projects.
Diyi Yang of Georgia Tech, our second keynote speaker, gave a talk titled Building Positive and Trustworthy Language Technologies, in which she described prior work on conceptualizing and categorizing various kinds of trust.
In the context of increasing human trust in AI, she talked about some of the published research from her group, ranging from formulating the positive-reframing problem, which aims to neutralize a negative point of view in a sentence and give the author a more positive perspective, to the Moral Integrity Corpus, a large dataset capturing the moral assumptions embedded in roughly 40,000 prompt-reply pairs. Novel benchmarks and tasks like these will prove a useful resource for building trustworthy language technologies.
In our final afternoon keynote session, Fei Wang of Weill Cornell Medicine gave a talk titled Towards Building Trustworthy Machine Learning Models in Medicine: Evaluation vs. Explanation. This keynote provided a comprehensive overview of the evolution of ML techniques as applied to clinical data, ranging from early works on risk prediction and matrix representations of patients using electronic-health-record data to more recent works on sequence representation learning.
Wang cautioned against common pitfalls of using ML methods for applications such as Covid detection, which include risks of bias in public repositories and Frankenstein datasets — hand-massaged datasets to get ideal model performance. He also emphasized the need for more robust explainability methods that can provide insights on model predictions in medicine.
TrustNLP panel
Our most popular session was the panel discussion in the afternoon, with an exciting and eclectic panel from industry and academia. Sara Hooker of Cohere for AI emphasized the need for more-robust tools and frameworks to help practitioners better evaluate various deployment-time design choices, such as compression or distillation. She also discussed the need for more-efficient ways of communicating research that can help policymakers play an active role in shaping developments in the field.
Ethan Perez of Anthropic AI argued the need for red-teaming large language models and how we could use existing language models to identify new types of weaknesses. Pradeep Natarajan of Alexa AI argued the need for communicating risks effectively by drawing on developments from old-school analytic fields such as finance and actuarial analysis.
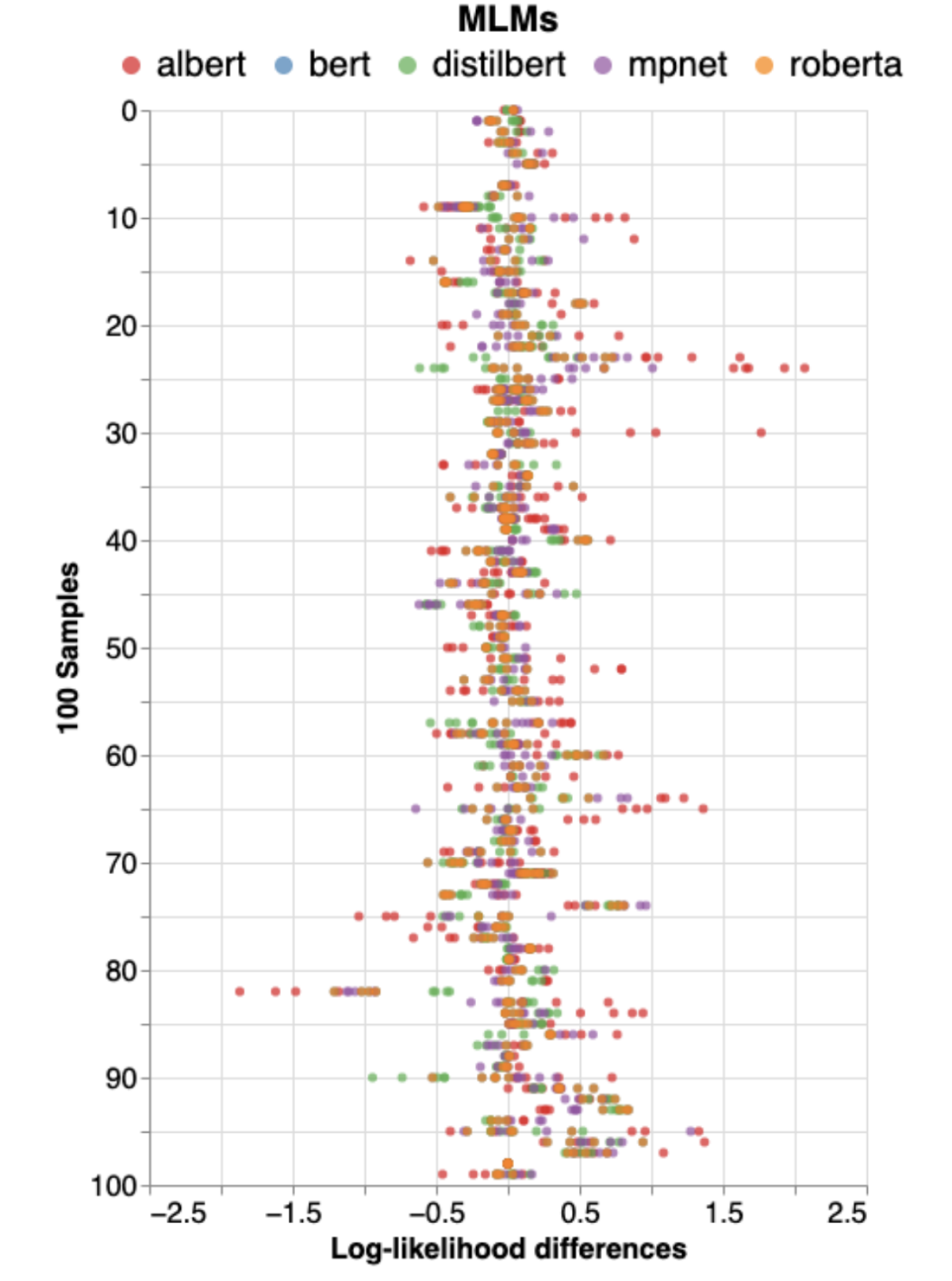
Yulia Tsvetkov from the University of Washington argued that models with good performance on predefined benchmarks still fail to generalize well to real-world applications. Consequently, she argued, there is a need for the community to explore approaches that are adaptive to dynamic data streams. Several panel members also acknowledged the expanding landscape in research, including community research groups producing top-quality research, and there was a healthy discussion around the similarities and differences between research in academia and in industry.
TrustNLP papers
Lastly, we had our wonderful list of paper presentations. The workshop website contains the complete list of accepted papers. The best-paper award went to "An empirical study on pseudo-log-likelihood bias measures for masked language models using paraphrased sentences", by Bum Chul Kwon and Nandana Mihindukulasooriya. The researchers study the effect of word choices/paraphrases in log-likelihood-based bias measures, and they suggest improvements, such as thresholding to determine the presence of significant log-likelihood difference between categories of bias attributes.
All the video presentations and live recordings for TrustNLP-2022 are available on underline.
Interspeech session
The special session at Interspeech was our first, and the papers presented there covered a wide array of topics, such as adversarial attacks, attribute and membership inference attacks, and privacy-enhanced strategies for speech-related applications.
We concluded the session with an engaging panel focused on three crucial topics in trustworthy ML: public awareness, policy development, and enforcement. In the discussion, Björn Hoffmeister of the Alexa Speech group stressed the importance of educating people about the risks of all types of data leakage — not just audio recordings and biometric signals — and suggested that this would create a positive feedback cycle with regulatory bodies, academia, and industry, leading to an overall improvement in customer privacy.
Google’s Andrew Hard highlighted the public’s desire to protect personal data and the risks of accidental or malicious data leakage; he stressed the need for continued efforts from the AI community in this space. On a related note, Bhiksha Raj of Carnegie Mellon University (CMU) suggested that increasing public awareness is a bigger catalyst for adoption of trustworthy-ML practices than external regulations, which may get circumvented.
Isabel Trancoso of the University of Lisbon stressed the pivotal role played by academia in raising general awareness, and she called attention to some of the challenges of constructing objective and unambiguous policies that can be easily interpreted in a diverse set of geographic locations and applications. CMU’s Rita Singh expanded on this point and noted that policies developed by a centralized agency would be inherently incomplete. Instead, she recommended a diverse set of — perhaps geographically zoned — regulatory agencies.
Multiple panelists agreed on the need for a concrete and robust measure for trustworthy ML, which can be reported for ML models along with their utility scores. Finally, Shrikanth Narayanan of the University of Southern California (also one of the session cochairs) provided concluding remarks, closing the session with optimism owing to the strong push from all sectors of the AI research community to increase trustworthiness in ML. The full set of papers included in the session are available on the Interspeech site.
We thank all the speakers, authors, and panelists for a memorable and fun learning experience, and we hope to return next year to discuss more exciting developments in the field.