In recent years, algorithmic bias has become a central topic of research across AI disciplines. Interest in the topic ballooned after a 2018 investigation of bias in face recognition software — where bias was defined as differential performance on subjects from different demographic groups.
The natural way to test a face recognition model for bias would be to feed it lots of images featuring subjects from different groups and see how it performs. But that requires data annotated to indicate subjects’ identity across images, and identity annotation is extremely costly — especially at the scale required to conclusively evaluate a face recognition model.
At this year’s European Conference on Computer Vision (ECCV), my colleagues and I presented a new method for assessing bias in face recognition systems that does not require data with identity annotations. Although the method only estimates a model’s performance on data from different demographic groups, our experiments indicate that those estimates are accurate enough to detect the differences in performance indicative of bias.
This result — the ability to predict the relative performance of a face identification model without test data annotated to indicate facial identity — was surprising, and it suggests an evaluation paradigm that should make it much more practical for creators of face recognition software to test their models for bias.
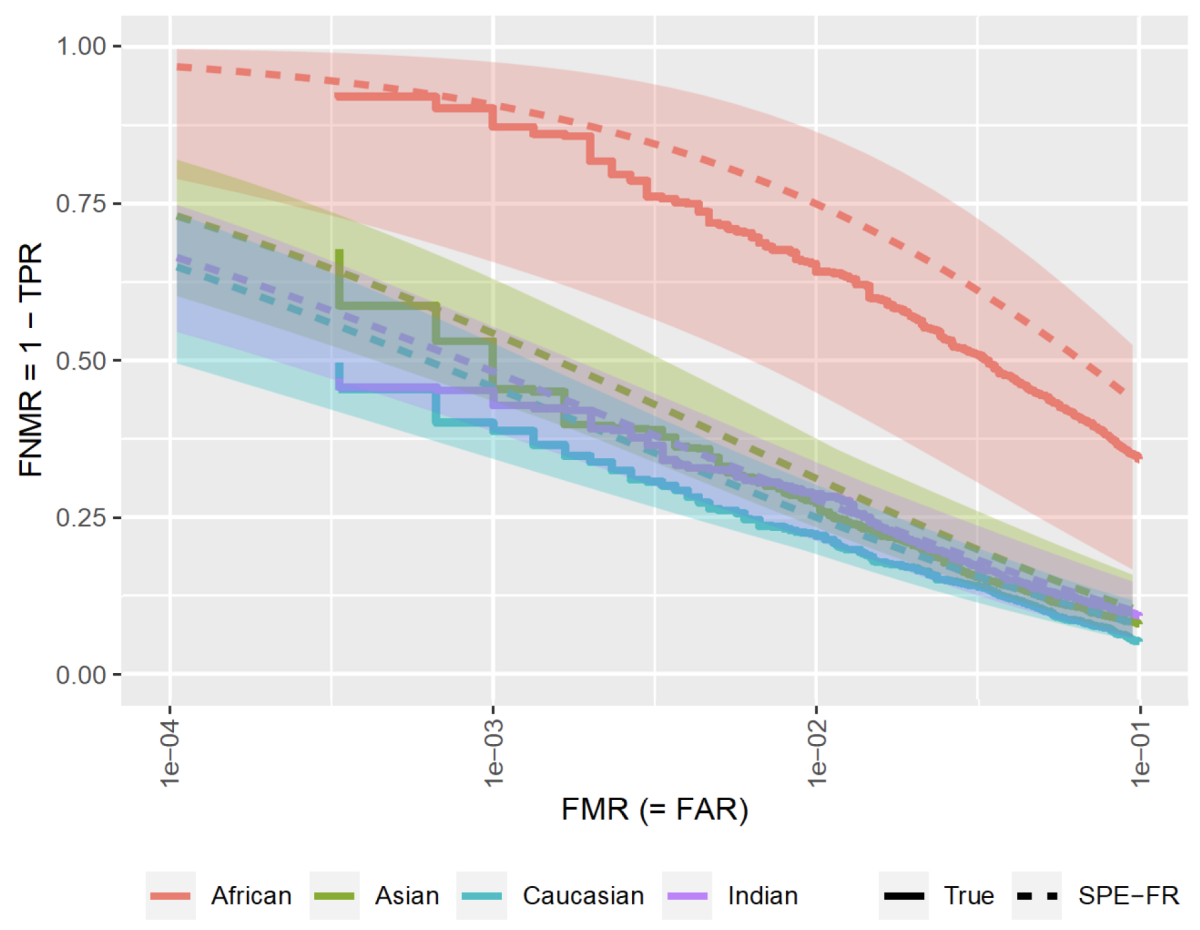
Besides its cost effectiveness, our method also has the advantage that it can be adapted on the fly to new demographic groups. It does require some means of identifying subjects who belong to those groups — such as image metadata from self-reporting — but it doesn’t require identity labels.
To evaluate our approach, we trained face recognition models on datasets from which particular demographic data had been withheld, to intentionally introduce bias. In all cases, our method was able to identify differential performance on the withheld demographic groups.
We also compared our approach to Bayesian calibration, a baseline method for predicting a machine learning model’s outputs. Our method outperformed Bayesian calibration across the board, sometimes by a large margin — particularly when you consider that Bayesian calibration requires some annotated data for bootstrapping, whereas our method relied entirely on unannotated data.
The model
From annotated training data, face recognition models typically learn to produce vector representations — embeddings — of input images and measure their distance from each other in the embedding space. Any embeddings whose distance falls below some threshold are classified as representing the same person.
We assume that the distances between true matches fall into some distribution, and the distances between non-identical faces fall into a different distribution. The goal of our method is to learn the parameters of those two distributions.
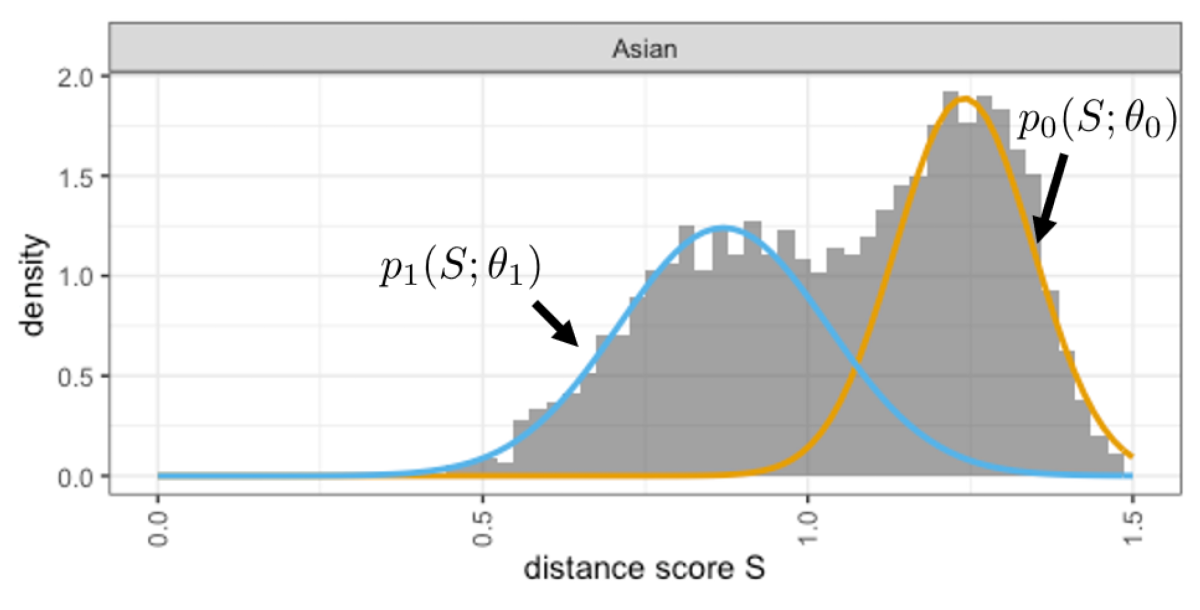
Empirically, we found that the score distributions tend to be slightly skewed, so we modeled them using a two-piece distributions. Two-piece distributions divide the distribution around the mode — the most commonly occurring value — and the distributions on either side of the mode have different parameters.
To evaluate a trained face recognition model, we feed it pairs of images that are annotated with demographic information but not with identity information. The face verification pairings are randomized: some are matches, and some are not, but we don’t know which are which.
From the resulting scores, our model learns a pair of distributions, one for matches and one for non-matches, and on the basis of the separation between the distributions, we can predict the accuracy of the model. We repeat this process for each demographic class in the dataset and compare the results.
Based on hierarchical clustering of the test samples, we can compute error bounds for our accuracy estimates, and our experiments show that even accounting for error, our approach can still provide a clear signal of disparity. We hope that this methodology will help AI practitioners working on face recognition or similar biometric tasks to ensure the fairness of their models.