The machine learning models that power conversational agents like Alexa are typically trained on labeled data, but data collection and labeling are expensive and complex, creating a bottleneck in the development process.
Large language models (LLMs) such as the 20-billion-parameter Alexa Teacher Model (AlexaTM 20B) might look like a way to break that bottleneck, since they excel in few-shot settings — i.e., when only a handful of labeled examples are available. But their size and computational costs are unsuitable for runtime systems, which require low latency and support high traffic volumes.
To enable models that are lightweight enough for runtime use, even when real training data is scarce, we propose teaching via data (TvD), in which we use an LLM-based “teacher” model to generate synthetic training data for a specific task, then use the generated data to fine-tune a smaller “student” model.
This blog post covers two of our recent papers on TvD. LINGUIST, published at the 2022 International Conference on Computational Linguistics (COLING), generates training data for joint intent classification and slot tagging (IC+ST). CLASP, published at the 2022 Conference of the Asia-Pacific Chapter of the Association for Computational Linguistics (AACL), generates training data for semantic parsing. Both tasks are core components of conversational AI.
We show that LINGUIST data generation improves on popular multilingual IC+ST benchmarks by 2 to 4 points absolute, while CLASP data generation improves multilingual semantic parsing by 5 to 6 points absolute.
The AlexaTM 20B model used in CLASP is now available on AWS JumpStart.
LINGUIST
Conversational-AI agents use intent classification and slot tagging (IC+ST) to understand the intent of a speaker’s request and identify the entities relevant to fulfilling that request. For example, when an agent is asked to “play ‘Wake Me Up’ by Avicii”, it might identify the intent as PlayMusic, with the slot value “wake me up” assigned to the slot Song and “Avicii” assigned to Artist. (Slot tagging in this context is also known as named-entity recognition, or NER.)
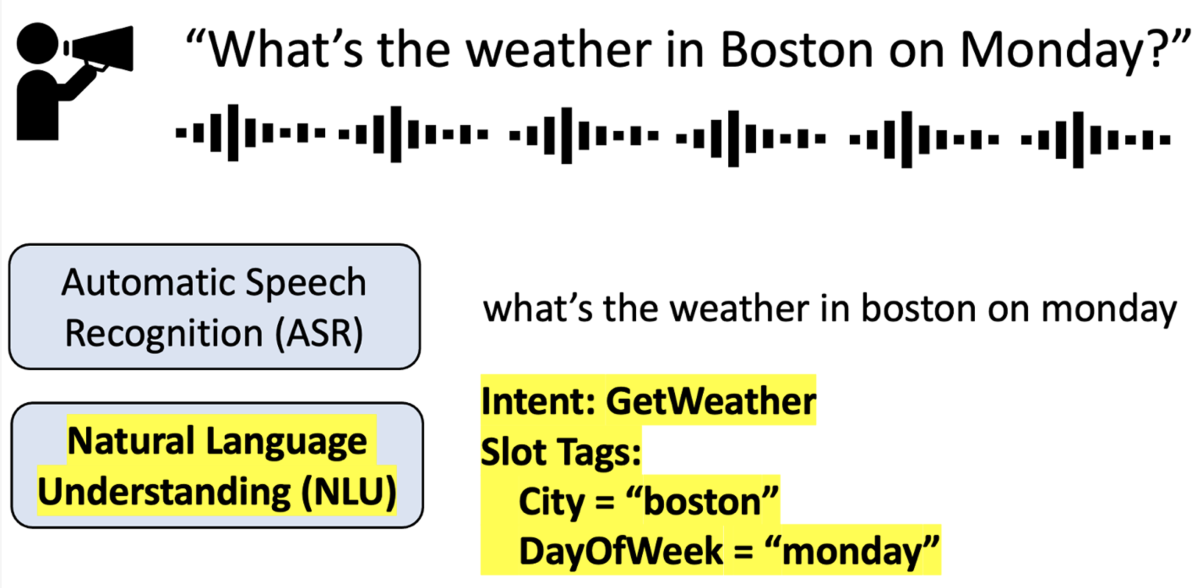
With real-world agents, the set of intents and slots grows over time as developers add support for new use cases. Furthermore, multilingual agents such as Alexa seek to maintain parity across languages when new intents and slots are developed, creating an additional bottleneck during development.
Suppose, for example, that we’re enabling a multilingual agent to understand the new intent GetWeather. To begin with, the intent may have only two associated utterances, in English and no other languages, annotated with the slots City and DayOfWeek. These two utterances alone are not enough to build a strong multilingual IC+ST model, so we need to obtain more training data.

A simple baseline approach to expanding this dataset to a new language is to translate the text. Here is an example using AlexaTM 20B with an in-context one-shot prompt. The text in the yellow box is the input to the model, and we can sample as many outputs from the model as we want, shown in the blue boxes.
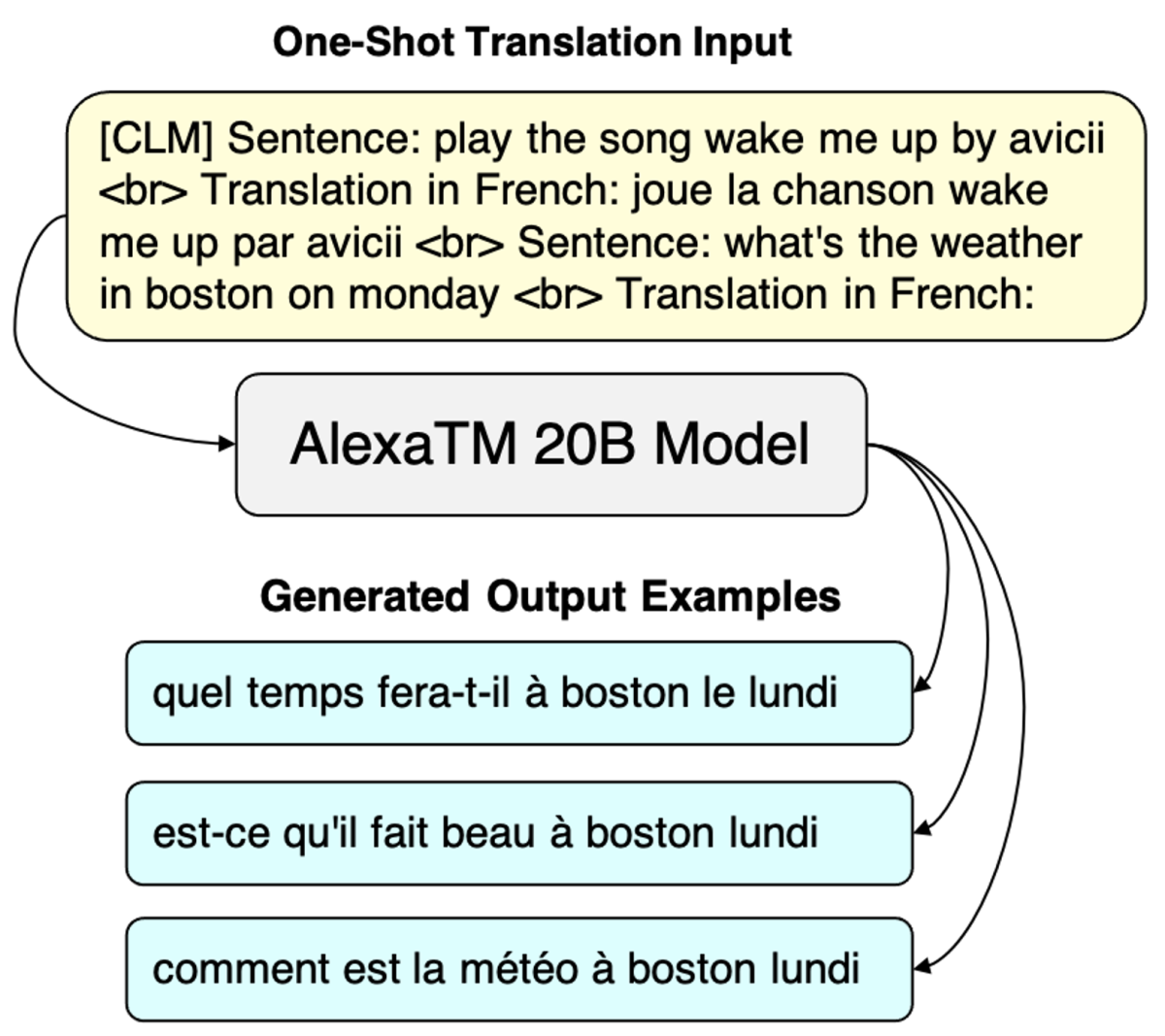
To get more examples in the original English, we can either translate these French outputs back to English (back-translation) or directly use a paraphrasing model, such as, again, AlexaTM 20B with an in-context prompt:
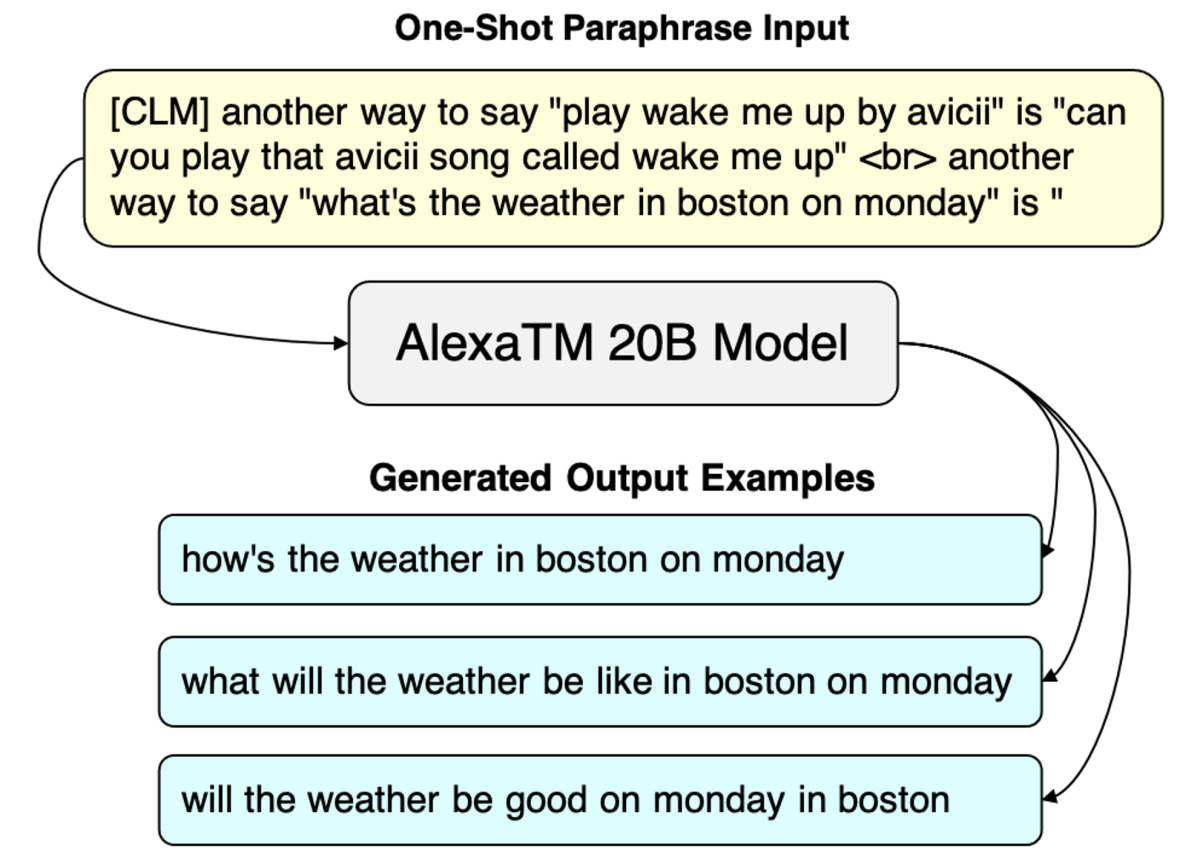
While these approaches go a long way, they have two key limitations: (1) the outputs don’t have the slot tags labeled, so we need to use a separate model (e.g., one that does word alignment) to guess which output words are City and which DayOfWeek, a process that introduces noise; and (2) we cannot control the outputs — say, by restricting them to specific slot types and values.
To address these two problems, we propose LINGUIST: language model instruction tuning to generate annotated utterances for intent classification and slot tagging. To control outputs, we design an instruction prompt whose syntax resembles that of web markup languages like HTML/XML, which the language model is likely to have encountered during pretraining.
We also introduce an output format with brackets and numbers that enables the model to produce synthetic data with the slots already tagged. In the output “[1 boston ]”, for instance, the numeral “1” indicates the slot tag City. We then fine-tune the teacher model on prompts and targets from existing data — either from other intents or from a separate public dataset like MASSIVE.
When developing a new intent or slot with only a few examples, we can now instruct the LINGUIST model to generate the data we are looking for. For instance, we can generate data for the GetWeather intent that always uses “Dallas” as the City, tagged with the number 1. For the DayOfWeek slot, tagged as number 2, we can use the special wildcard instruction “*”, telling the model to fill in an appropriate value, and it will produce novel values like “Saturday” and “Thursday”, which did not appear in the original examples.
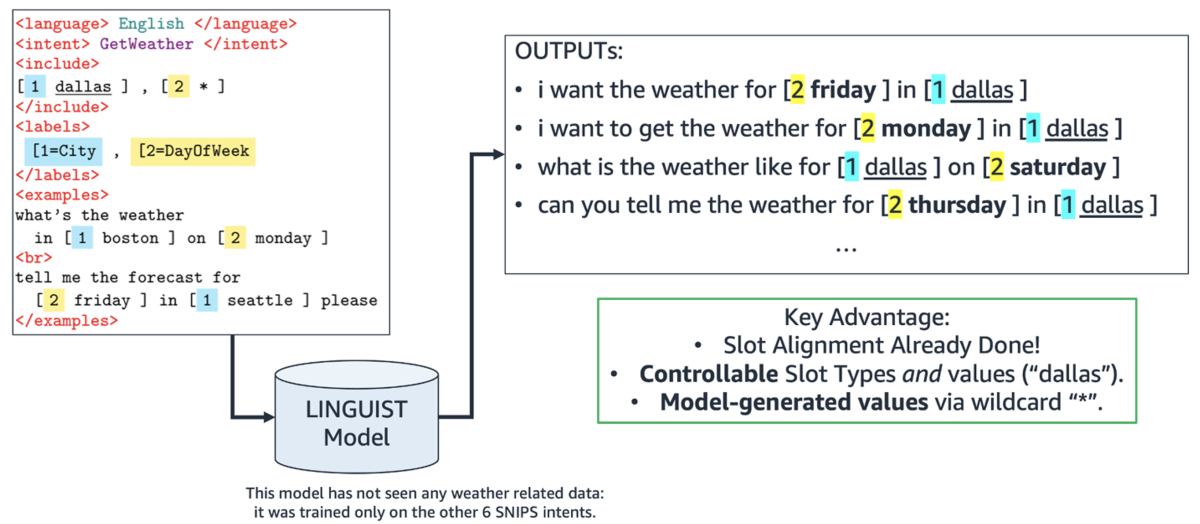
We also built a mechanism to control the output language: by simply changing the prompt to indicate “French” instead of English, we get outputs in French.
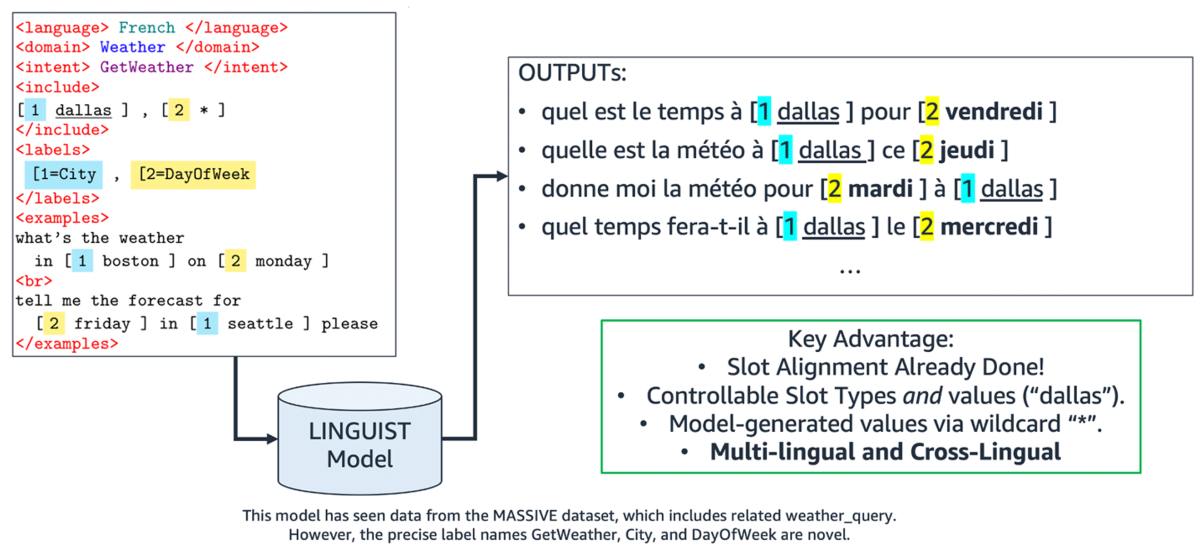
Finally, LINGUIST can generate annotated training data even when we have zero examples to start with, by attending to natural-language label names like “GetWeather”, “City”, and “DayOfWeek”. In this case, there is less information on the input side, so the output contains more noise. However, the generated data is still useful for building a model for new intents and slots.
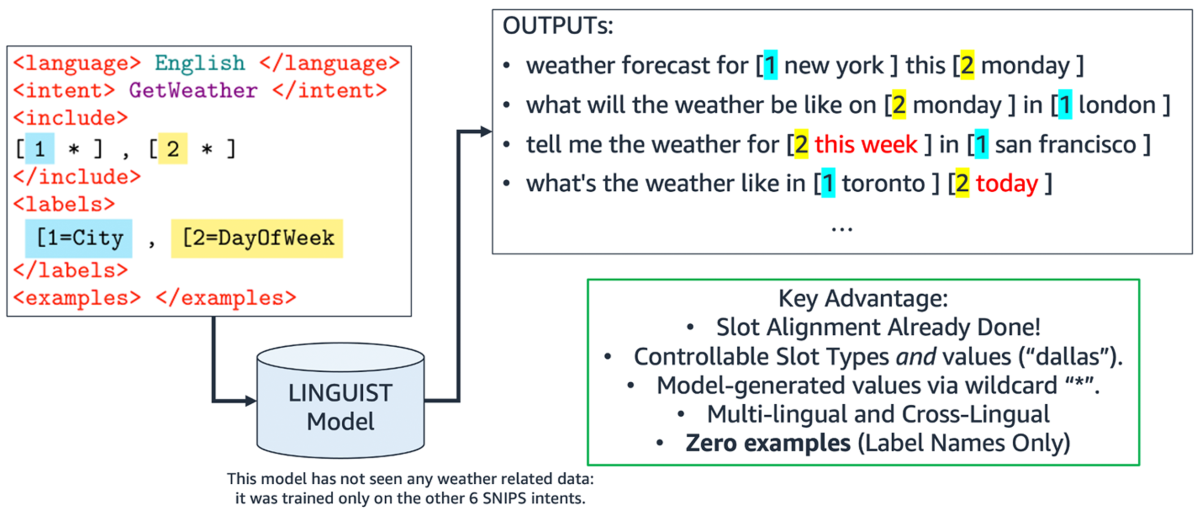
In the paper, we show that LINGUIST outperforms state-of-the-art baselines like translation and paraphrasing by 2-4 points absolute on the public datasets SNIPS and mATIS++ across seven languages.
CLASP
While intent classification and slot tagging cover many interactions with conversational agents, they are limited in scope. For more complex queries, we instead apply semantic parsing (SP). Here is an example from the PIZZA dataset: “large pizza with extra cheese and pineapple hold the ham and two sprites please”. We need SP to recover relevant information like the value of the implicit Number slot, the scope of the modifiers Quantity and Not, and the association between multiple intents and slots.

SP is even more difficult to annotate than IC+ST, so the training datasets tend to be smaller, especially in languages other than English; we don’t have a MASSIVE dataset for semantic parsing. For example, the PIZZA dataset has only 348 real examples to train on (and in our experiments, we also explore the lower-resource setting of 16 examples).
Again adopting the teaching-via-data (TvD) approach, we propose CLASP: few-shot cross-lingual data augmentation for semantic parsing. CLASP consists of four strategies to prompt LLMs like AlexaTM 20B to generate SP training data.
The first two strategies, CLASP-RS (replace slots) and CLASP-TS (translate slots), modify an existing parse by replacing the slots with other values, either from a catalogue of options or via translation to a new language. Then the model generates text to match the new parse.
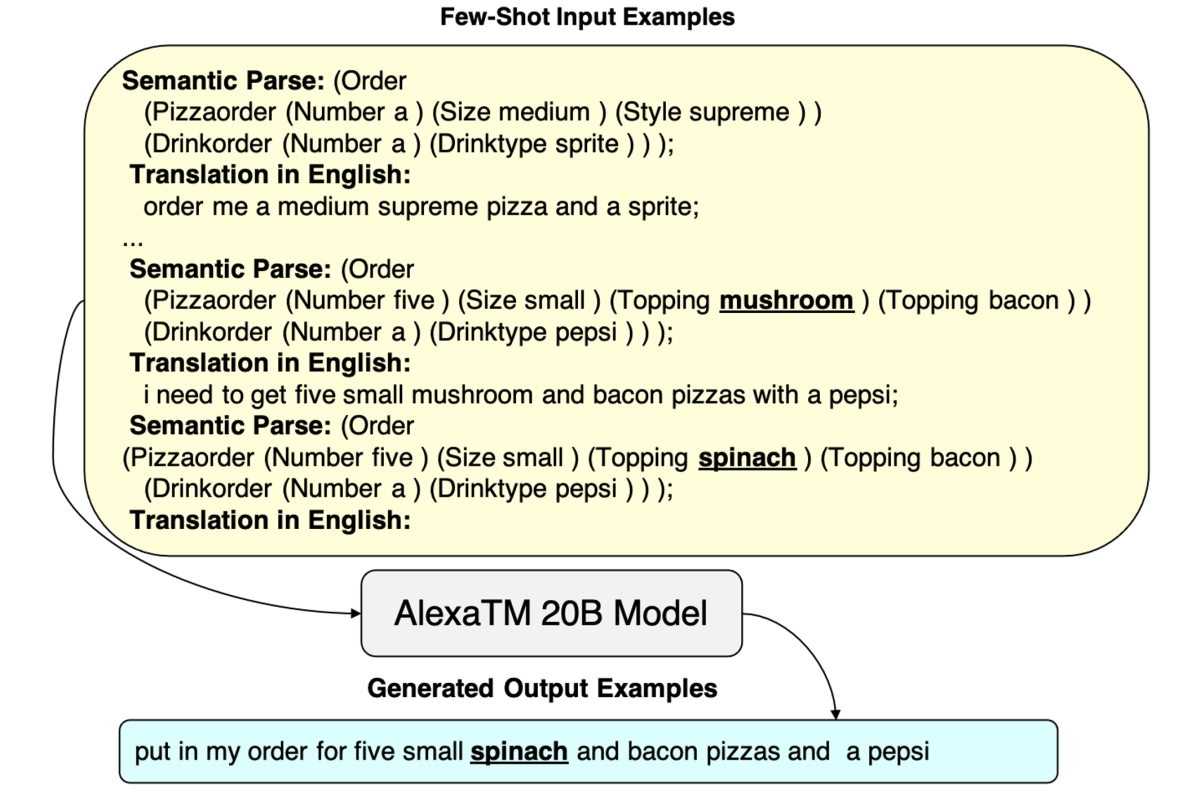
The other two strategies, CLASP-GB (generate both) and CLASP-TB (translate both), give the model more flexibility, instructing it to generate both the parse and the text, in either the same language or a new language.
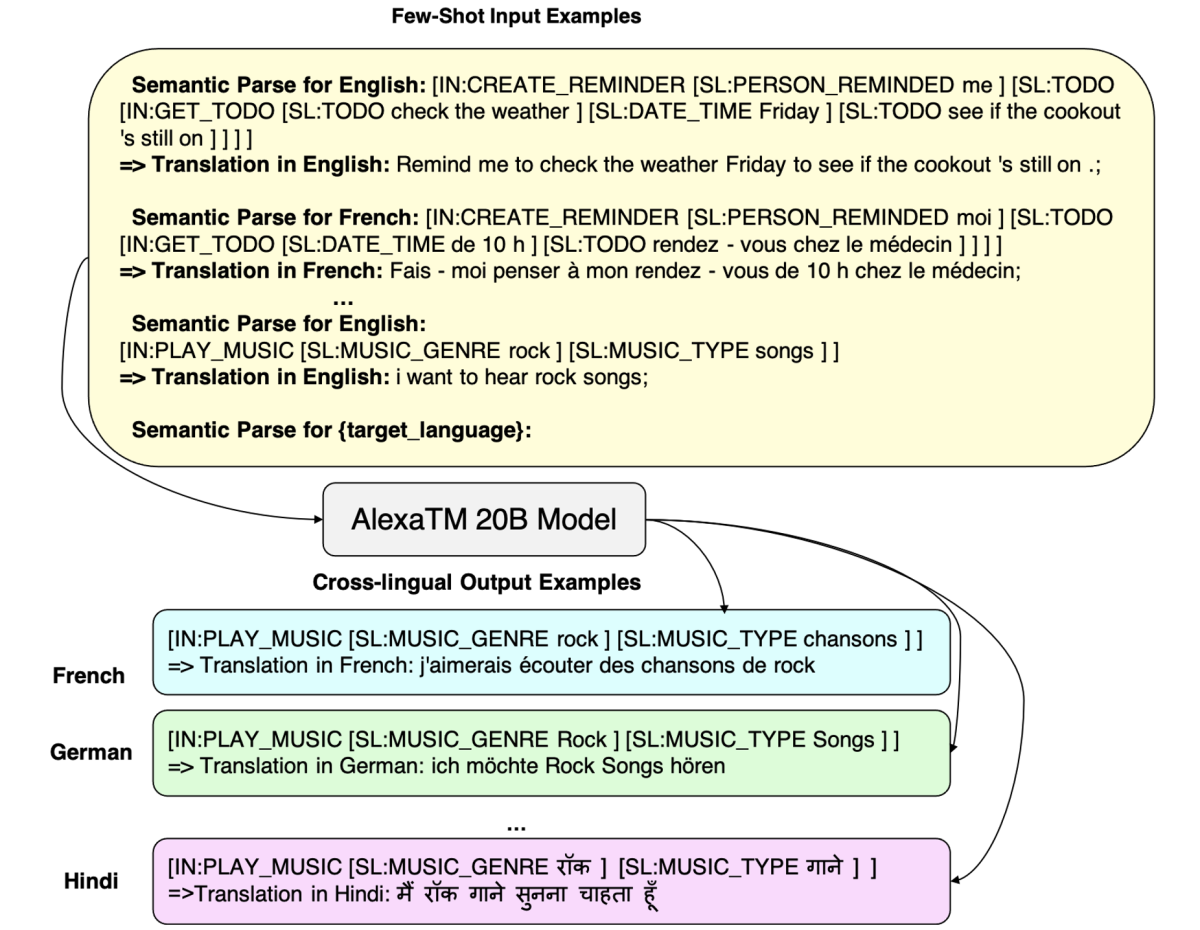
AlexaTM 20B can perform these generation tasks quite reliably from only a few in-context examples, which is remarkable given that it was pretrained only on public text from the web and is not specialized for semantic parsing.
For our experiments on data generation for semantic parsing, the baselines we selected include grammar sampling (drawback: unrealistic examples) and translation with alignment (drawback: alignment is challenging and introduces noise).
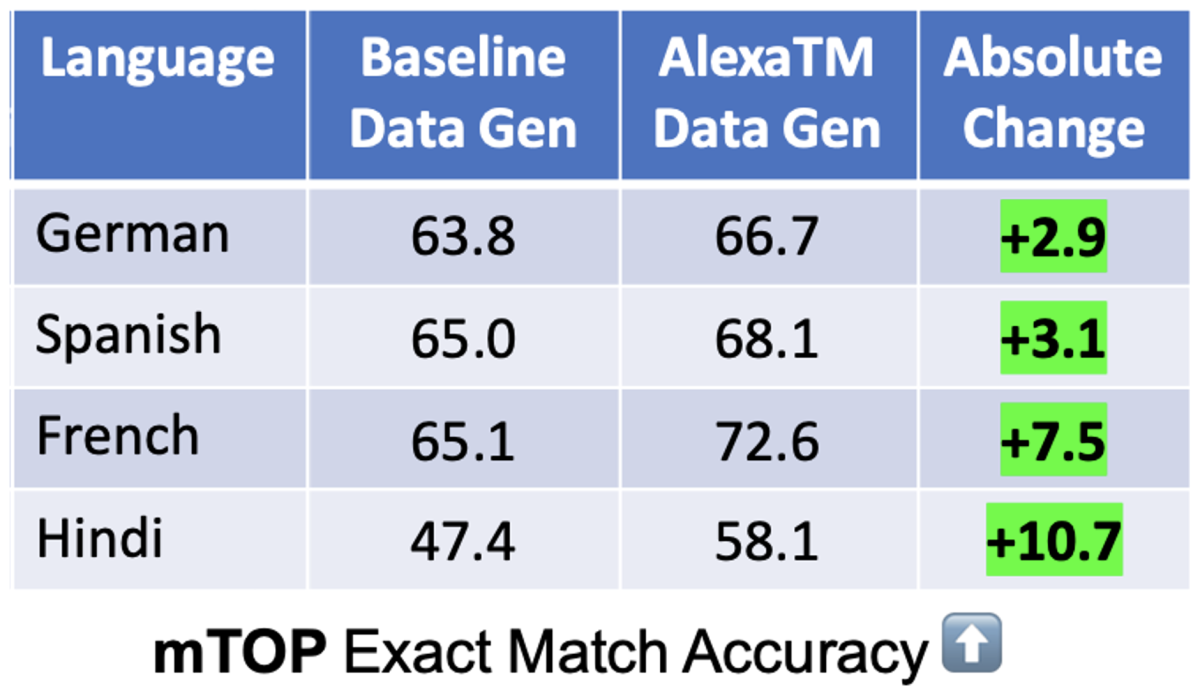
Using English-language examples from the PIZZA dataset, in the low-resource setting with only 16 real examples, we improve exact-match accuracy by 5 points absolute, topping 85%. On the popular mTOP dataset, we improve over machine translation by 6 points absolute across four new languages, by leveraging only one annotated example from each language.
At Amazon Alexa AI, we continue to explore TvD for tasks such as question answering and dialogue and for additional languages. We have just scratched the surface of what’s possible and are optimistic about the future of TvD. We look forward to continuing to invent methods to improve our models and make our customers’ lives better and easier every day.