Traditionally, Alexa has interpreted customer requests according to their intents and slots. If you say, “Alexa, play ‘What’s Going On?’ by Marvin Gaye,” the intent should be PlayMusic, and “‘What’s Going On?’” and “Marvin Gaye” should fill the slots SongName and ArtistName.
But simple intent-and-slot tagging won’t work for requests like “Alexa, add peanut butter and milk to the shopping list and play music.” Handling requests like this, which have compound intents and slot values, requires a semantic parser that analyzes both the structure of a sentence and the meanings of its component parts.
Using machine learning to build semantic parsers is difficult because the training data requires such complex annotation. At this year’s meeting of the North American Chapter of the Association for Computational Linguistics, we address this problem by combining two techniques.
The first is transfer learning, which reduces the amount of data necessary to train a new machine learning model by transferring knowledge from existing models. The other is a copying mechanism, which enables the model to deal with words it’s never seen before, such as the names of particular musical artists. That’s particularly important when training data is sparse.
We tested our parser on two tasks: natural-language understanding (NLU) and question answering. In tests involving NLU data from Alexa interactions, we found that the copy mechanism alone increased the accuracy of our semantic parser by an average of 61%, while transfer learning added a relative 6.4% on top of that.
For question-answering, we used two public data sets, which included free-form questions such as “What restaurant can you eat outside at?” or “How many steals did Kobe Bryant have in 2004?” There, we found that transfer learning improves performance by 10.8%.
In the traditional intent-slot paradigm, annotating the instruction “play music” would mean simply tagging the word “music” with the slot type Mediatype and the instruction as a whole with the intent PlayMusicIntent. But annotating utterances as semantic parse trees is more complex. Here, for instance, is the semantic parse tree of the instruction “Add apples and oranges to shopping list and play music”:
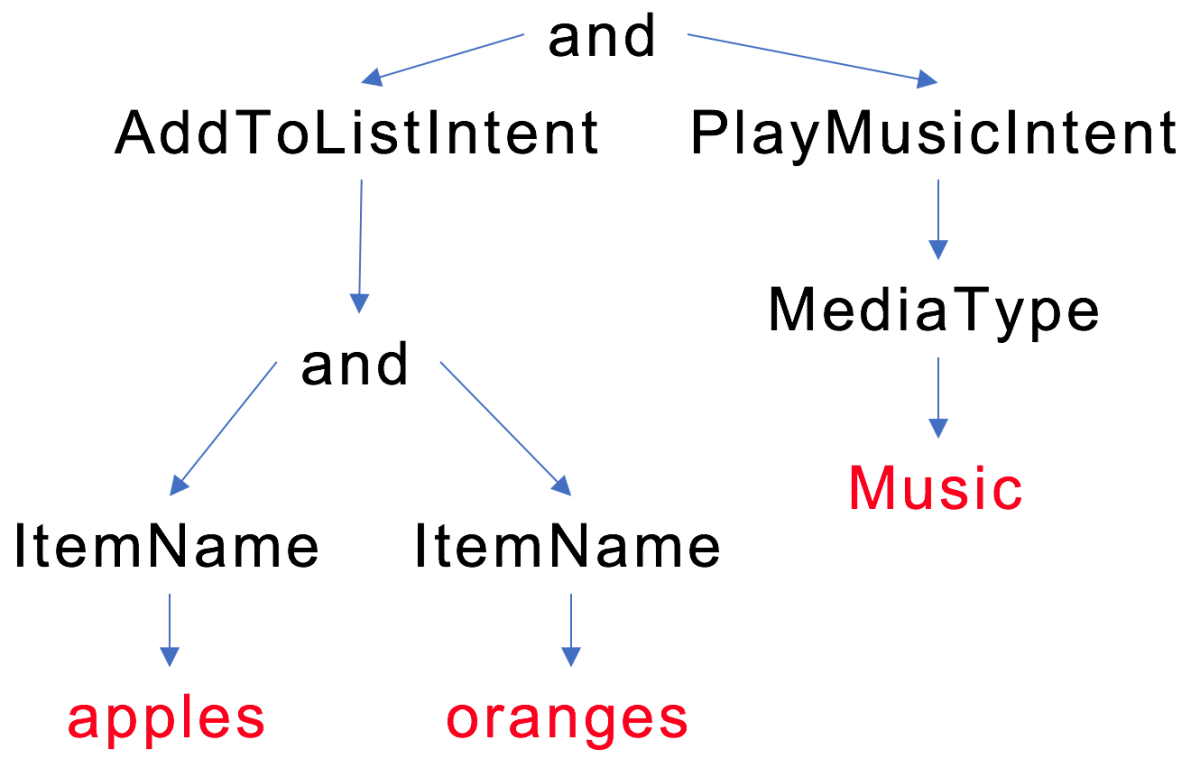
As in a syntactic parse, the tree depicts the grammatical structure of the request. For instance, the request consists of two main clauses (“add apples and oranges to shopping list” and “play music”), joined by the conjunction “and”. So “and” appears at the top of the tree, with a clause on either side.
But the parse is semantic because it also interprets the meaning of the utterance. For instance, the words “to shopping list”, which appear in the request, do not appear in the tree, because the semantic content of “add … to shopping list” is entirely captured by the intent AddToListIntent.
Data used to train semantic parsers can be annotated using a formal language that encodes tree structures. This is the encoding of the tree above:
(and(addToListIntent(add(ItemName(Apples))(ItemName(Oranges))))(PlayMusicIntent(Mediatype(Music))))
Obviously, producing this type of annotation requires expertise, and even for experts it can be time consuming and error prone. That’s why it’s important to use the small amount of annotated data available as efficiently as possible.
We generated a training set by automatically converting data annotated according to the intent-slot model into parse trees. Here, for example, is the intent-slot annotation of the question “Which cinemas screen Star Wars tonight?”:

And here is the parse tree generated by our algorithm:
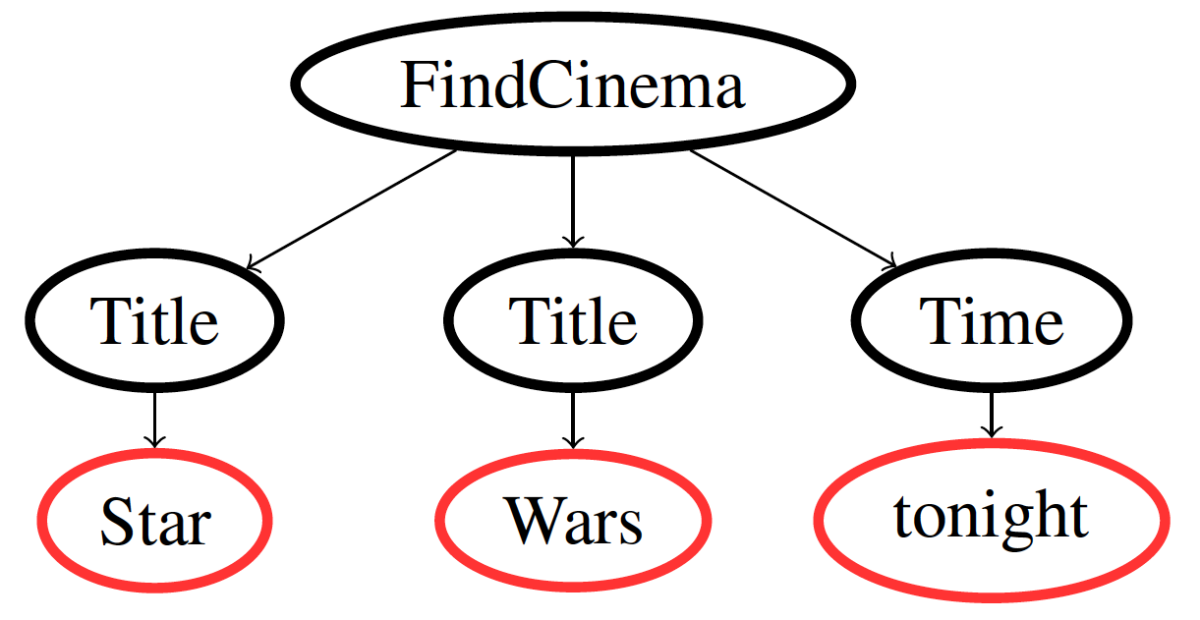
Our semantic parser is a shift-reduce parser, which allows us to construct a tree like the one above through a series of shift and reduce operations. “Shift” means that the parser moves to the next word in the input; “reduce” means that a word is assigned its final position in the tree. Our design is based on one from Mirella Lapata’s group at the University of Edinburgh.
One of our modifications to the Edinburgh model was the addition of a copy mechanism. The upper regions of a parse tree will generally contain words from a limited lexicon: either the intent and slot categories for a particular application or frequently occurring words such as “and”.
But the bottom of the tree — the “leaves”, or terminal nodes — will often contain named entities, as in the examples above. When assigning a value to a terminal node, the parser must decide whether to use a word from its lexicon or simply copy over a word from the input stream. In our model, this decision is facilitated by an attention mechanism, which tracks words recently examined by the parser and assesses the probability that each is a candidate for copying. On our data set of Alexa utterances, the addition of the copy mechanism improved the accuracy of parse tree construction by 61%.
We also experimented with two different techniques for doing transfer learning. With the first, called pretraining, we trained our model on all but one of a data set’s categories and then retrained it on just the remaining category. With the second, called multitasking, we trained the model on all of a data set’s categories but added a separate output layer for each category, rendering the model general rather than category-specific.
Pretraining worked better for some data categories, multitasking for others. But overall, across data sets, if we take the best-performing systems, transfer learning afforded an average improvement of 9.3%.
The fact that our semantic parser improves performance on both natural-language-understanding and question-answering tasks indicates its promise as a general-purpose technique for representing meaning, which could have other applications, as well.
Acknowledgments: Marco Damonte, Tagyoung Chung