Depth information is essential to many robotic applications, e.g., localization, mapping, and obstacle detection. But existing depth acquisition devices, such as Lidar and structured-light sensors, are typically bulky and power-consuming, while binocular depth cameras require regular recalibration and may lack accuracy in low-texture scenes.
For some applications, monocular depth estimation (MDE), which predicts depth directly from a single image, is more practical. It has the advantages of low cost, small size, high power efficiency, and a calibration-free lifetime of use.
But cameras differ in both their hardware and software, which means that the images they produce are subtly different, too. A machine-learning-based MDE model trained on images from a single camera may take advantage of the camera's distinctive visual style. Consequently, the model may not generalize well to images produced by different cameras. This is known as the domain shift problem.
In a paper that we are presenting at this year's International Conference on Intelligent Robots and Systems (IROS), we propose a new deep-learning-based method for adapting an MDE model trained on one labeled dataset to another, unlabeled dataset. Our approach relies on the insight that depth cues in an image depend more on the image content — for example, the types of objects in the image — than on the image style.
In experiments, we compared our approach to its leading predecessors and found that, on average, it reduced the depth error rate by about 20% while also reducing computational costs by more than 27%, as measured in MACs (multiply-accumulate operations).
Style vs. content
A human who closes one eye can still derive a good deal of depth information about a visual scene, thanks to extensive prior knowledge. To mimic that feat, MDE needs to not only learn objects’ depth-related structure but also extract some empirical knowledge, which can be more sensitive to particularities of camera design or image setting. Even changes of imaging environment may result in inferior depth prediction accuracy — e.g., low lighting or foggy conditions.
Collecting ground-truth depth annotations for multiple cameras and imaging conditions is costly and labor-intensive. Hence, developing algorithms that transfer the knowledge learned from a labeled dataset to a different, unlabeled dataset becomes increasingly important.
We approach this domain shift problem via unsupervised domain adaptation, in which, given a labeled source dataset and an unlabeled target dataset, the objective is to learn an MDE model that generalizes well to the target data.
We assume that the image feature space can be decomposed into content and style components. The content component consists of semantic features that are shared across different domains. For example, consider images of indoor scenes from two different datasets. Objects like tables, chairs, and beds are content information. Such semantic features are more domain-invariant, so it is easier to align the content features from different domains.
In contrast, the style component is domain-specific. For instance, style features like texture and color are unique to the scenes captured by a particular camera, so aligning style features across domains may not be beneficial.
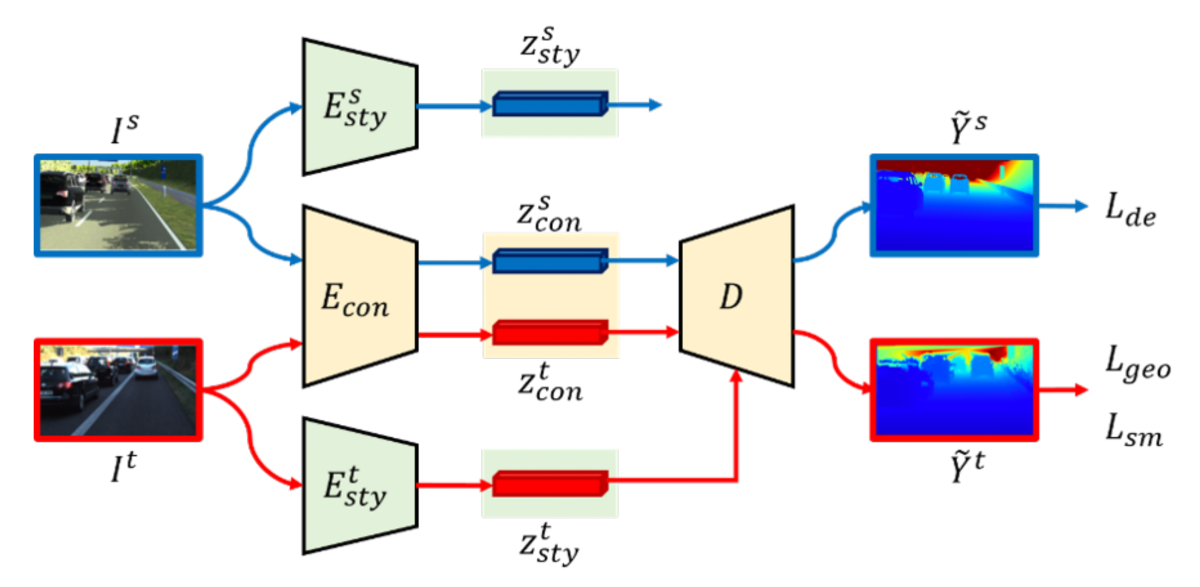
Loss functions
Our method relies on a deep neural network and a loss function with three components: a feature decomposition loss, a feature alignment loss, and — the primary objective — a depth estimation loss.
The feature decomposition loss involves a secondary transformation task, in which a generator is trained to recombine images’ style and content embeddings to (1) reconstruct the original images in each dataset and (2) transfer the style of each dataset to the content of the other.
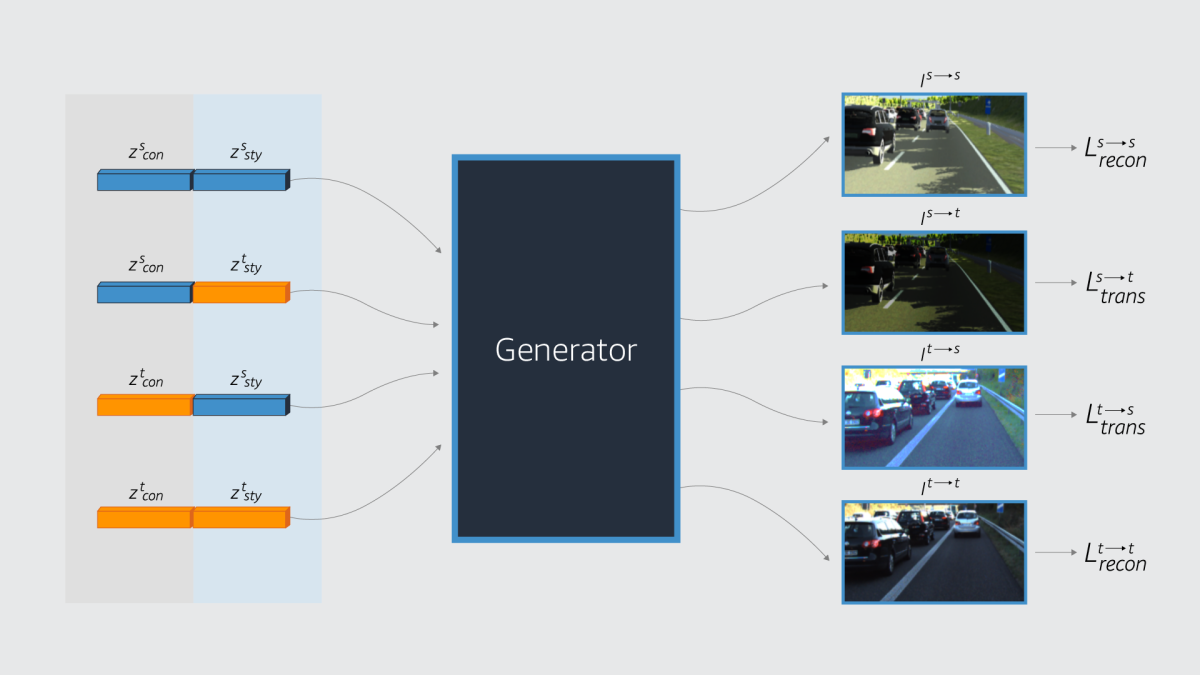
The feature decomposition loss leverages the internal representations of a pretrained image recognition network, whose lower layers tend to respond to pixel-level image features (such as color gradations in image patches) and whose higher levels tend to respond to semantic characteristics (such as object classes).
When comparing the styles of the generator’s outputs, the feature decomposition loss gives added weight to the representations encoded by the network’s lower layers; when comparing content, it gives added weight to the representations produced by the upper layers. This guides the encoder toward embeddings that distinguish style and content.
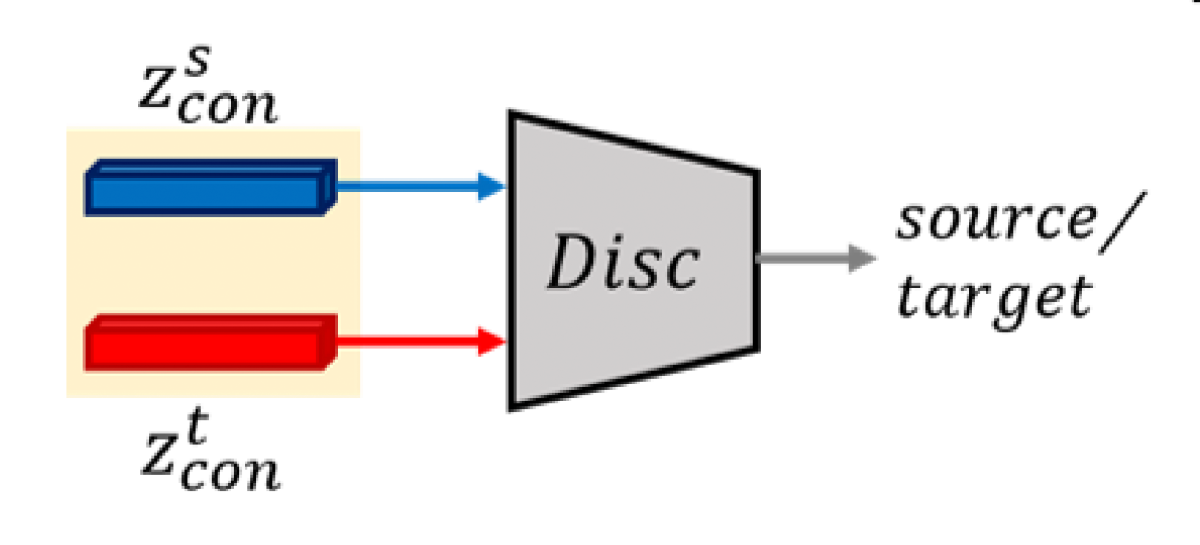
The feature alignment loss also relies on a secondary task: adversarial discrimination. The content encodings from both the source and target datasets pass to a discriminator, which attempts to determine which input came from which dataset. Simultaneously, the encoder attempts to learn embeddings that frustrate the discriminator.
To further improve content feature alignment, we use a technique called separatebatch normalization, in which the model learns the statistics of source and target data individually, further peeling off their uniqueness during the encoding and decoding process. The features are then normalized by the individual statistics and aligned into a common space.
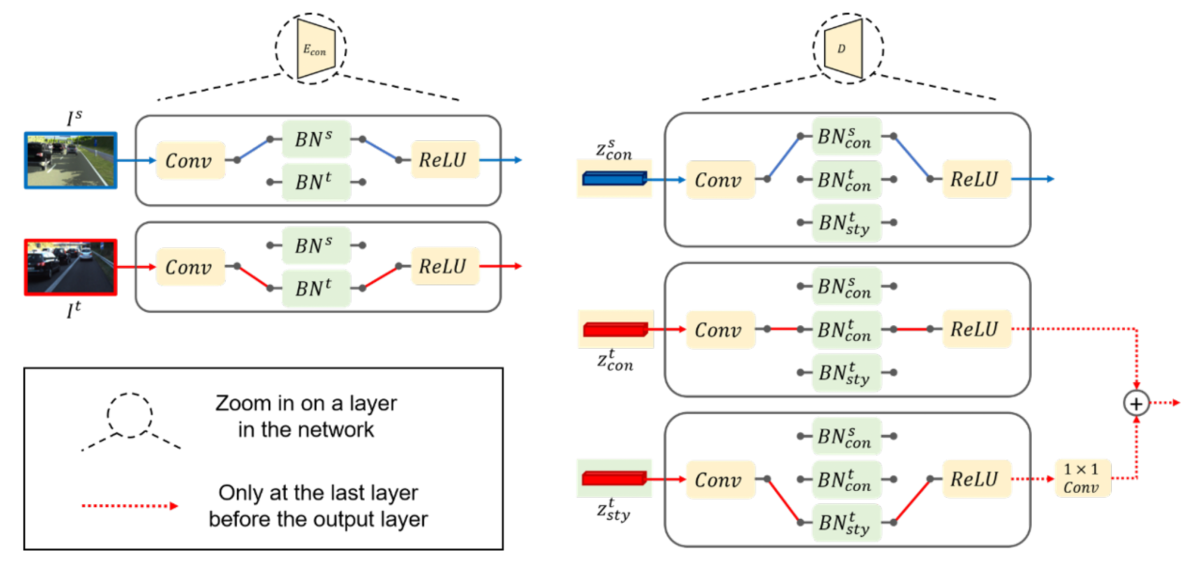
Finally, the model’s loss function also includes a term that assesses depth estimation error.
Our model keeps a relatively compact structure at inference time, so it’s less complex than predecessors that require a sophisticated image translation network for inference. And where most existing approaches rely on multistage training procedures that pretrain each sub-network separately and fine-tune them together, our method can be trained end-to-end in a single stage, making it easier to deploy in practical applications.
We evaluated our model in three broad scenarios: (1) cross-camera adaptation, (2) synthetic-to-real adaptation, and (3) adverse-weather adaptation. To the best of our knowledge, our paper is the first attempt to address all three scenarios for the MDE task. Particularly, it is the first to explore adverse-weather adaptation for MDE.
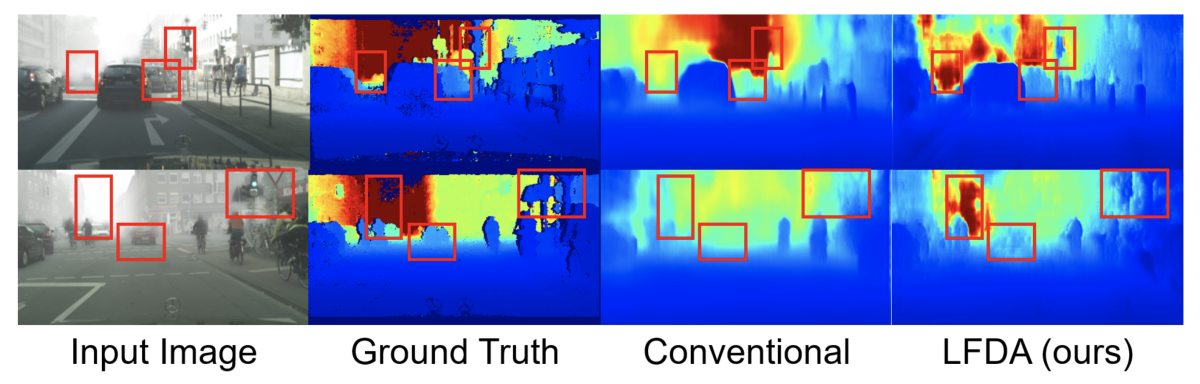
We hope our work will inspire other researchers to push the boundary of domain adaptive monocular depth estimation and that we will soon see the related technologies in Amazon products.