Teaching large language models (LLMs) to reason is an active topic of research in natural-language processing, and a popular approach to that problem is the so-called chain-of-thought paradigm, in which a model is prompted not just to answer questions but to provide rationales for its answers.
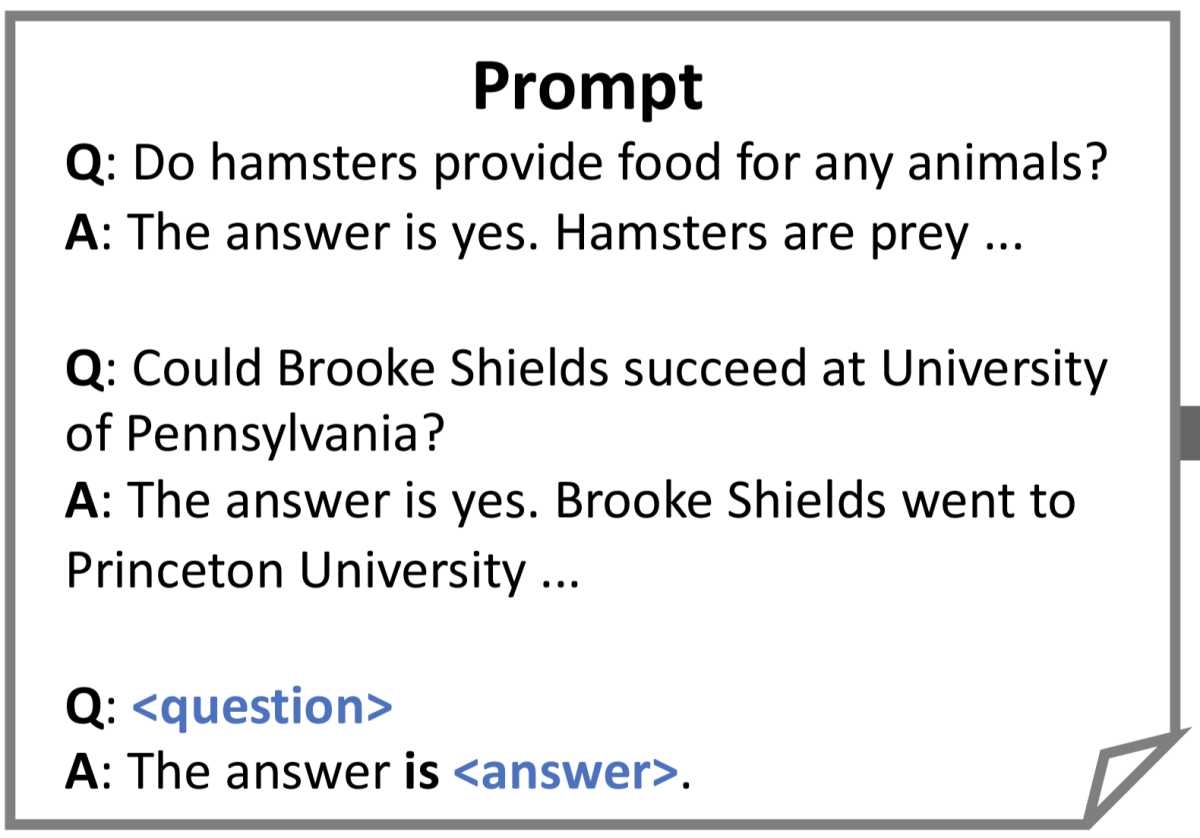
However, given LLMs’ tendency to hallucinate (that is, make spurious factual assertions), the generated rationales may be inconsistent with the predicted answers, making them untrustworthy.
In a paper we presented at this year’s meeting of the Association for Computational Linguistics (ACL), we show how to improve the consistency of chain-of-thought reasoning through knowledge distillation: given pairs of questions and answers from a training set, an LLM — the “teacher” — generates rationales for a smaller “student” model, which learns to both answer questions and provide rationales for its answers. Our paper received one of the conference’s outstanding-paper awards, reserved for 39 of the 1,074 papers accepted to the main conference.
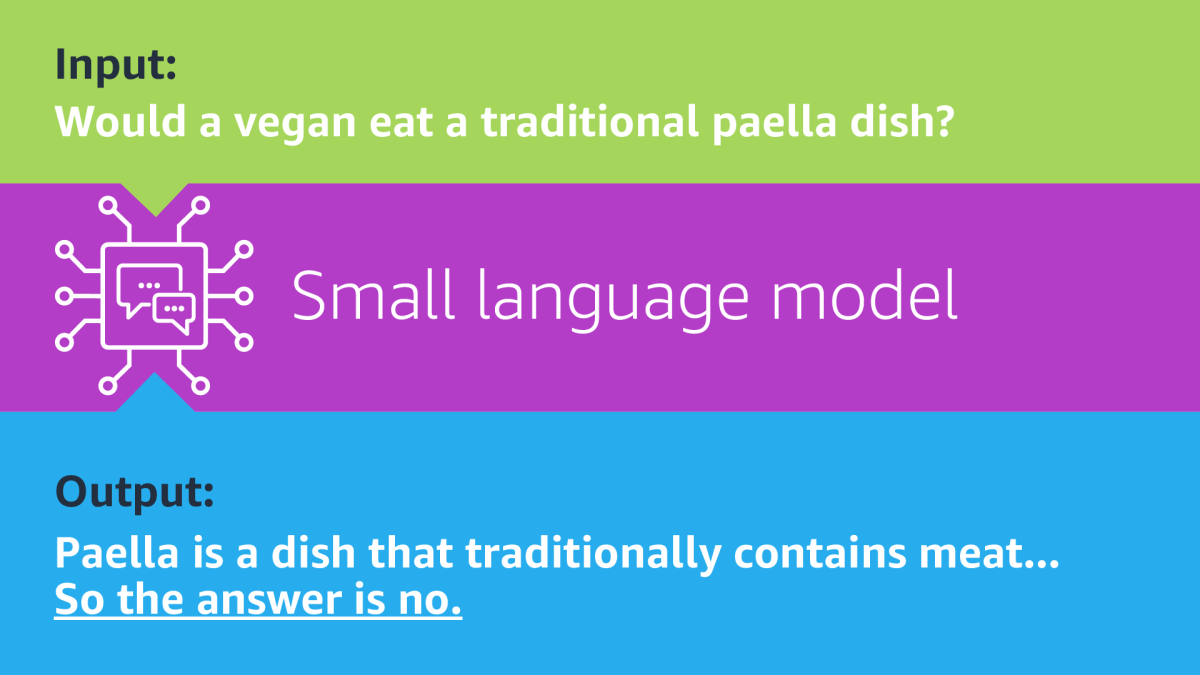
With knowledge distillation (KD), we still have to contend with the possibility that the rationales generated by the teacher are spurious or vacuous. On the student side, the risk is that while the model may learn to produce rationales, and it may learn to deliver answers, it won’t learn the crucial logical relationships between the two; it might, for instance, learn inferential short cuts between questions and answers that bypass the whole reasoning process.
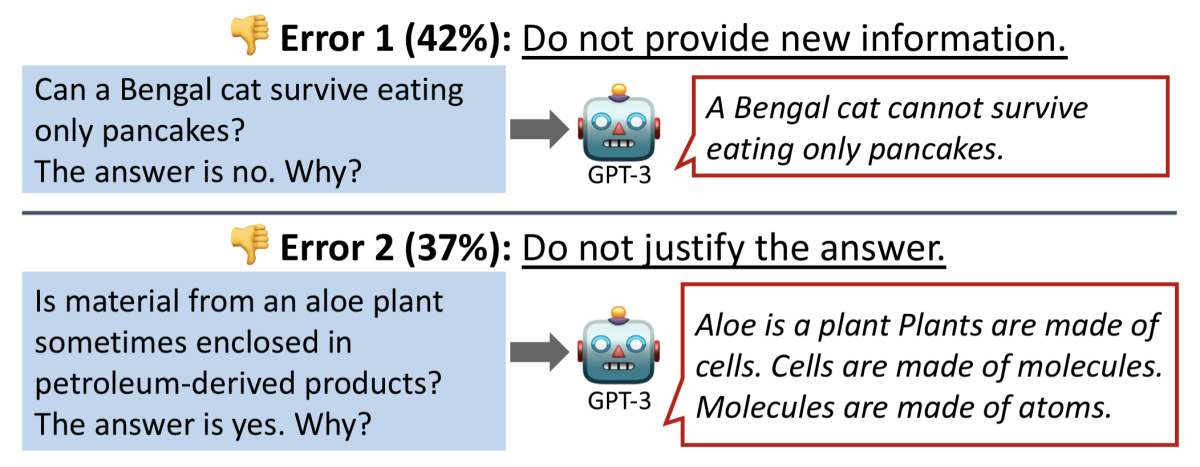
To curb hallucination, on the teacher side, we use contrastive decoding, which ensures that the rationales generated for true assertions differ as much as possible from the rationales generated for false assertions.
To train the student model, we use counterfactual reasoning, in which the model is trained on both true and false rationales and must learn to provide the answer that corresponds to the rationale, even if it’s wrong. To ensure that this doesn’t compromise model performance, during training, we label true rationales “factual” and false rationales “counterfactual”.
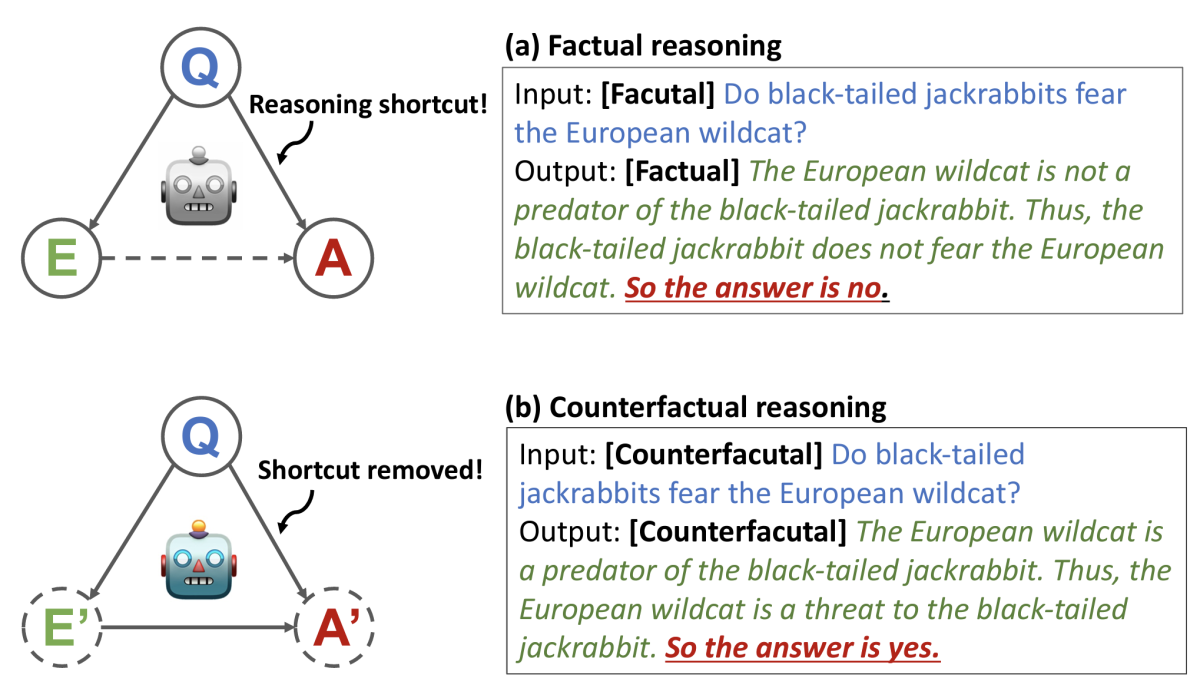
To evaluate our model, we compared it to a chain-of-thought model built using ordinary knowledge distillation, on datasets for four different reasoning tasks. We asked human reviewers to evaluate the rationales generated by the teacher models. To evaluate the student models, we used the leakage-adjusted simulatability (LAS) metric, which measures the ability of a simulator (an external model) to predict the student’s outputs from the generated rationales. Across the board, our models outperformed the baselines, while preserving accuracy on the reasoning tasks.
Contrastive decoding
As our teacher model, we use a trained LLM whose parameters are frozen. To generate training examples for the student model, we use in-context learning, in which we provide the teacher with a handful of examples of questions, answers, and human-annotated rationales, then supply a final question-answer pair. The model generates the rationale for the final pair.
During training, LLMs learn the probabilities of sequences of words. At generation time, they either select the single most probable word to continue a sequence or sample from the top-ranked words. This is the standard decoding step, which doesn’t guarantee that the generated rationales justify the model’s answers.
We can control the decoding process without making any adjustments to the LLM parameters. With contrastive decoding, we perform the same in-context rationale generation twice, once with the true answer in the final question-answer pair and once with a perturbed answer.
Then, when we’re decoding the true question-answer pair, we select words that are not only probable given the true pair but relatively improbable given the false pair. In other words, we force the rationale for the true pair to diverge from the rationale for the false pair. In this way, we ensure that the output skews toward rationales particularized to the answers in the question-answer pairs.
In our experiments, we considered two types of perturbation to the true answers: null answers, where no answer at all was supplied, and false answers. We found that contrastive decoding using false answers consistently yielded better rationales than contrastive decoding using null answers.
Counterfactual reasoning
Past research has shown that question-answering models will often exploit short cuts in their training data to improve performance. For instance, answering “who?” questions with the first proper name encountered in a source document will yield the right answer with surprising frequency.
Similarly, a chain-of-thought model might learn to use shortcuts in answering questions and generate rationales as a parallel task, without learning the crucial connection between the two. The goal of training our model on a counterfactual-reasoning objective is to break that short cut.
To generate counterfactual training data, we randomly vary the answers in question-answer pairs and generate the corresponding rationales, just as we did for contrastive decoding. Then we train the student model using the questions and rationales as input, and it must generate the corresponding answers.
This means that the student model may very well see the same question multiple times during training, but with different answers (and rationales). The “factual” and “counterfactual” tags prevent it from getting confused about its task.
In our experiments, we compared our approach to one that also uses in-context learning but uses greedy decoding to produce rationales — that is, a decoding method that always selects the highest-probability word. We also used two other baselines: an LLM that directly generates rationales from in-context learning and a model trained on human-annotated rationales.
Our study with human evaluators showed that in-context learning with contrastive decoding generated more persuasive rationales than in-context learning with greedy decoding:
Teacher Model | Grammaticality | New Info | Supports Answer |
Greedy | 0.99 | 0.65 | 0.48 |
Contrast.-Empty | 0.97 | 0.77 | 0.58 |
Contrast.-Wrong | 0.97 | 0.82 | 0.63 |
Table: Human evaluation of data generated with greedy decoding, contrastive decoding using empty answers, and contrastive decoding using incorrect answers.
In the experiments using the LAS metric, knowledge distillation using contrastive decoding alone consistently outperformed all three baselines, and knowledge distillation with counterfactual reasoning and contrastive decoding consistently outperformed knowledge distillation with contrastive decoding alone. The model trained on the human-annotated dataset yielded the most-accurate results on downstream tasks, but its rationales fared badly. On average, our model was slightly more accurate than the one trained using greedy decoding.
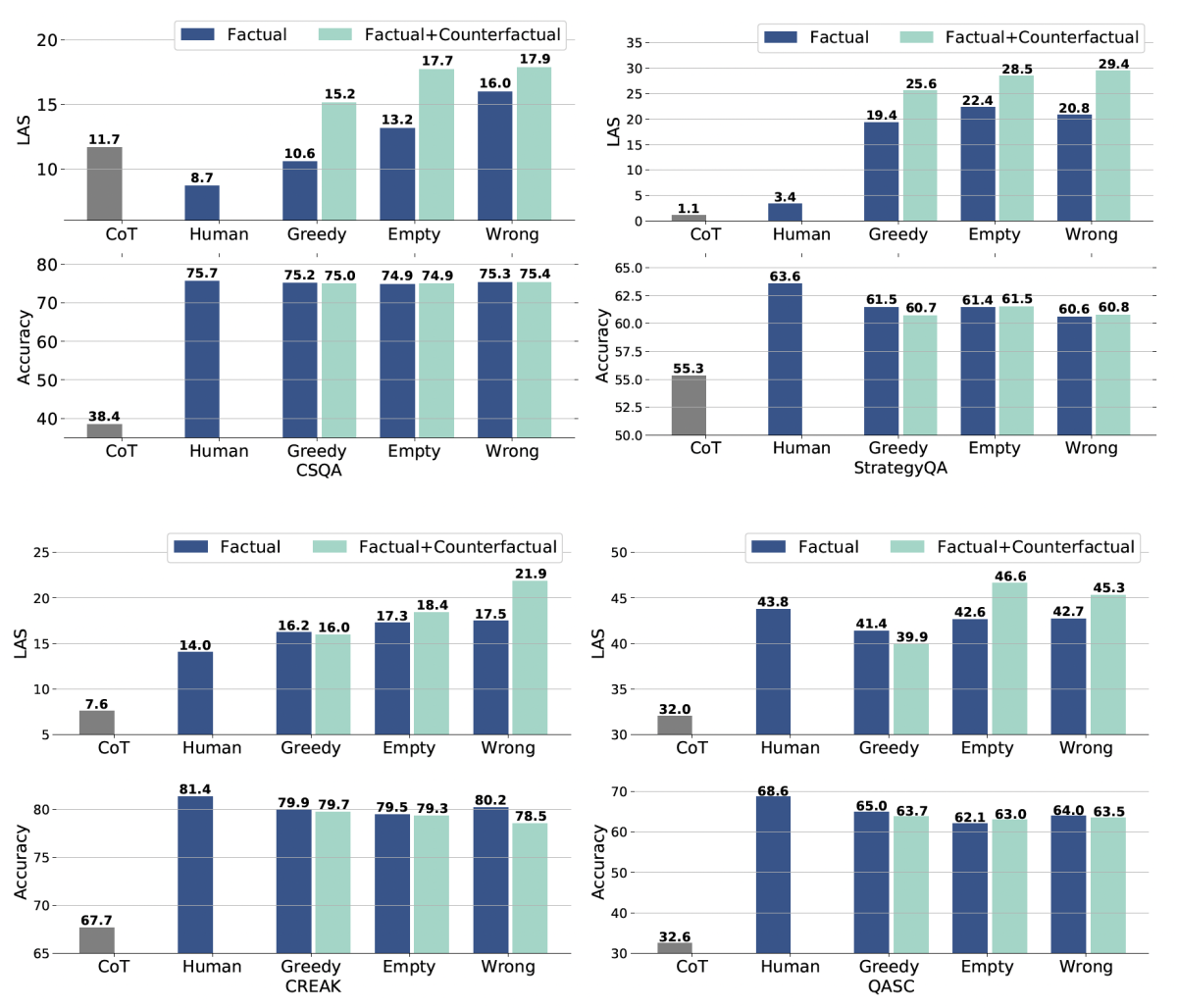