Question answering through reading comprehension is a popular task in natural-language processing. It’s a task many people know from standardized tests: a student is given a passage and questions based on the passage — say, an article on William the Conqueror and the question “When did William invade England?” The student reads the passage and learns that the answer is 1066. In natural-language processing, we aim to teach machine learning models to do the same thing.
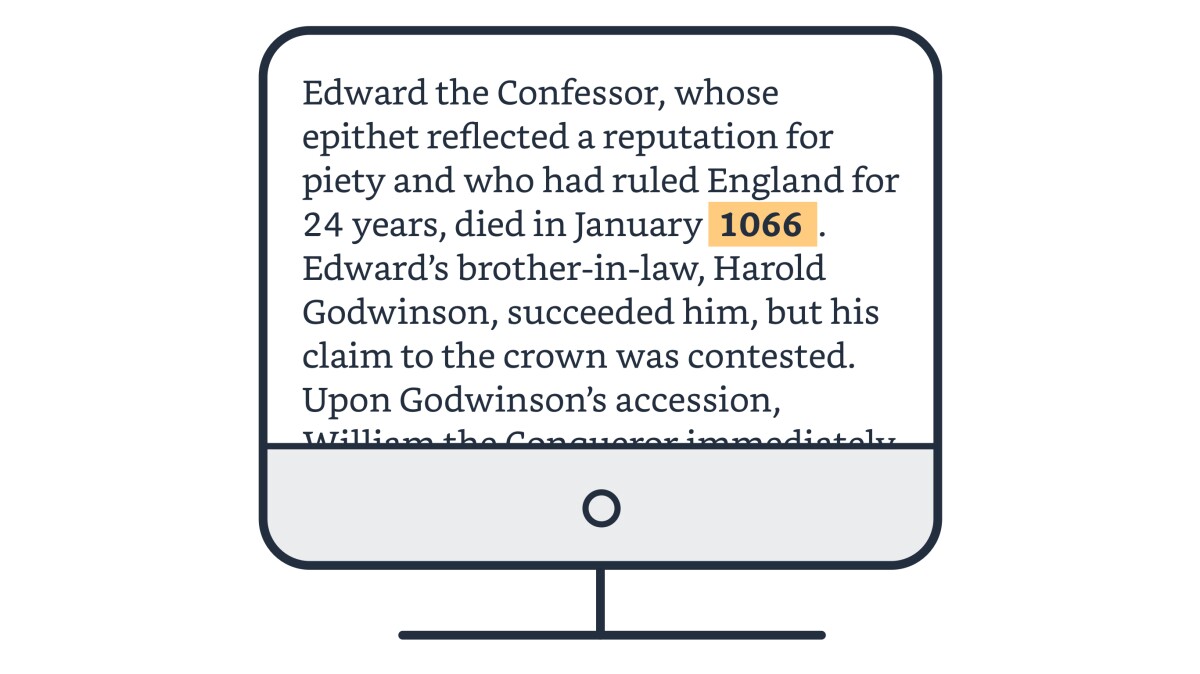
In recent years, question-answering models have made a lot of progress. In fact, models have started outperforming human baselines on public leaderboards such as SQuAD 2.0.
Are the models really learning question answering, or are they learning heuristics that work only in some circumstances? We investigate this question in our paper “What do models learn from question answering datasets?”, which we’re presenting at the Conference on Empirical Methods in Natural Language Processing (EMNLP).
In this paper, we subject question-answering models built atop the popular BERT linguistic model to a variety of simple yet informative attacks. We identify shortcomings that cast doubt on the idea that models are really outperforming humans. In particular, we find that
(1) Models don’t generalize well
A student who is a good critical reader should be able to answer questions about a variety of articles. A student who can answer questions about William the Conqueror but not Julius Caesar may not have learned reading comprehension —just information about William the Conqueror.
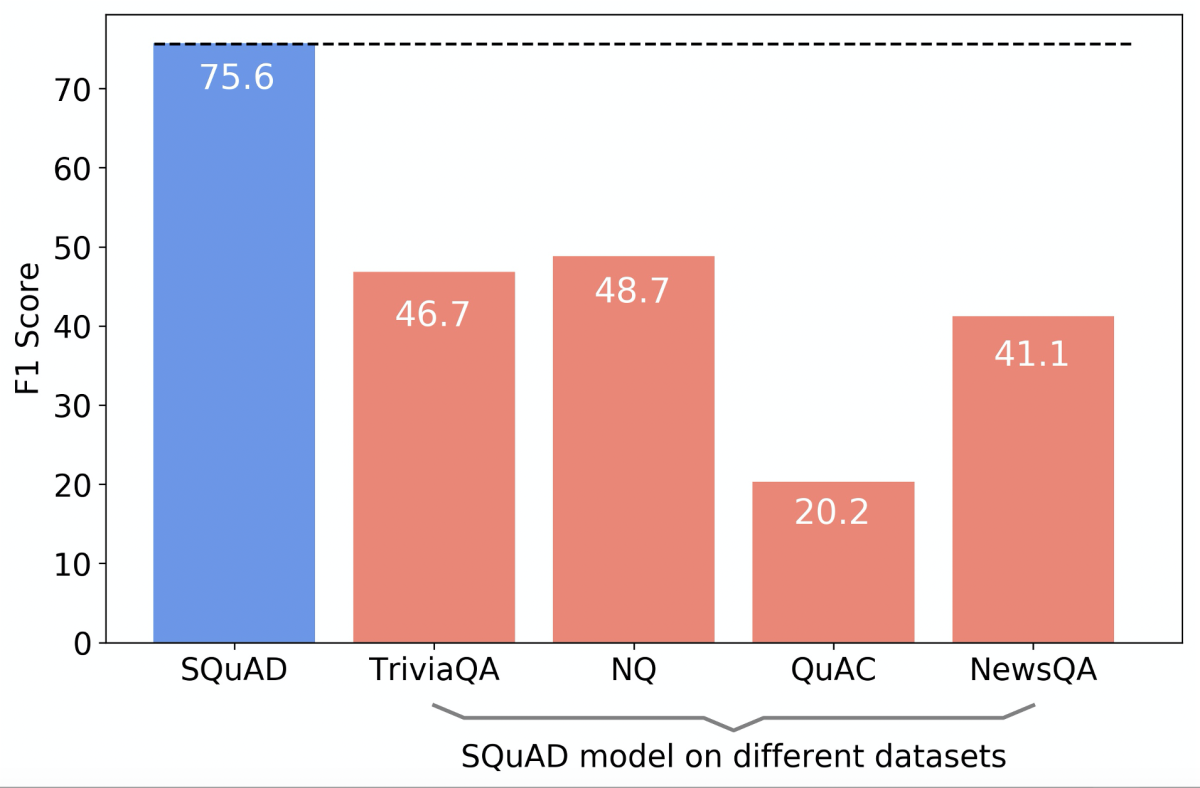
Question-answering models do not generalize well across data sets. A model that does well on the SQuAD data set doesn’t do well on the Natural Questions data set, even though both contain questions about Wikipedia articles. This suggests that models can solve individual data sets without necessarily learning reading comprehension more generally.
(2) Models take short cuts
When testing question-answering models, we assume that high performance means good understanding of the subject. But tests can be flawed. If a student takes a multiple-choice test where every answer is “C”, it’s hard to judge whether the student really understood the material or exploited the flaw. Similarly, models may be picking up on biases in test questions that let them arrive at the correct answer without doing reading comprehension.
To probe this, we conducted three experiments. The first was a modification at training time: we corrupted training sets by replacing correct answers with incorrect answers — for instance, “Q: ‘When did William invade England?’ A: ‘William is buried in Caen’”.
The other two were modifications at test time. In one, we shuffled the sentences in the input articles so that they no longer formed coherent paragraphs. In the other, we gave models incomplete questions (“When did William?”, “When?”, or no words at all).
In all these experiments, the models were suspiciously robust, continuing to return correct answers. This means that they didn’t need to do reading comprehension at training time or at test time to understand the structure of the articles or be asked the full question.
How can this be? It turns out that some questions in some data sets can be answered trivially. In our experiments, for example, one model was just answering all “who” questions with the first proper name in the passage. Simple rules like this can get us to almost 40% of current model baselines.
(3) Models aren’t prepared to handle variations
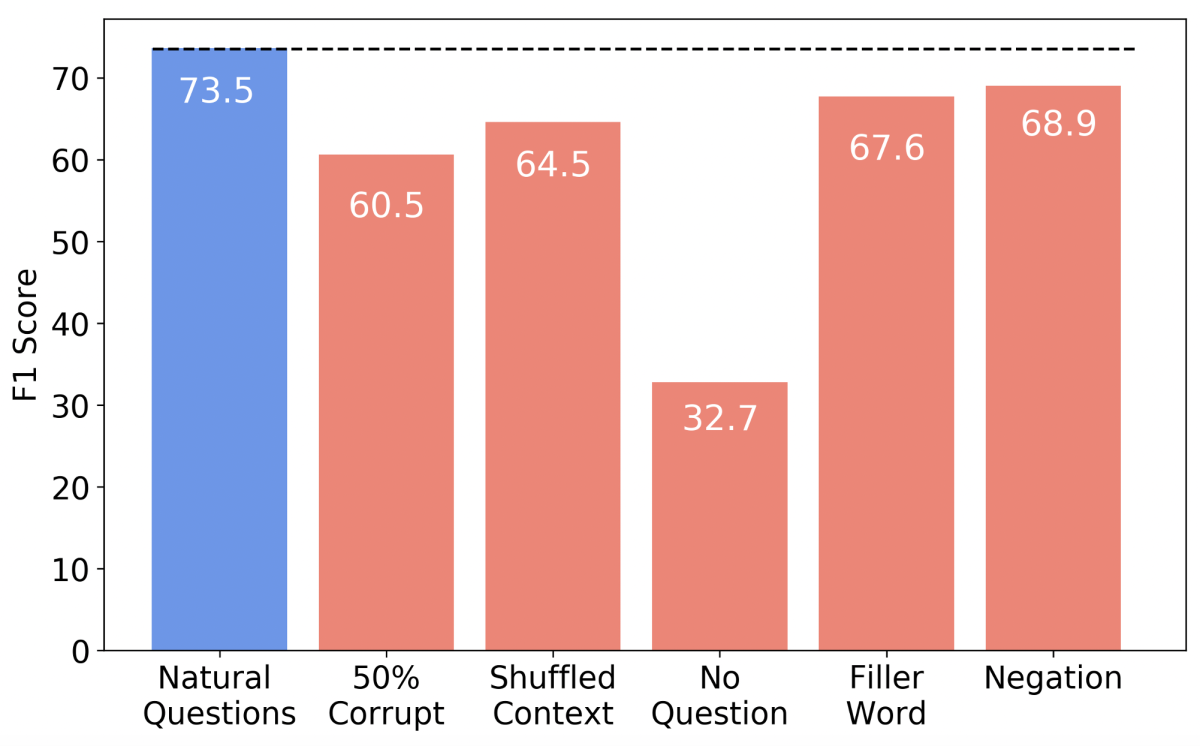
A student should understand that “When did William invade England?”, “When did William march his army into England?”, and “When was England invaded by William?” are all asking the same question. But models can still struggle with this.
We conducted two experiments where we ran variations of questions through reading comprehension models. First, we tried the very simple change of adding filler words to questions (“When did William really invade England?”). In principle, this should have no effect on performance, but we found that it reduces the model’s F1 score — a metric that factors in both false positives and false negatives — by up to 8%.
Next, we added negation (“When didn’t William invade England?”) to see if models understood the difference between positive and negative questions. We found that models ignore negation up to 94% of the time and return the same answers they would to positive questions.
Conclusions
Our experiments suggest that models are learning short cuts rather than performing reading comprehension. While this is disappointing, it can be fixed. We believe that following these five suggestions can lead to better question-answering data sets and evaluation methods in the future:
- Test for generalizability: Report performance across multiple relevant data sets to make sure a model is not just solving a single data set;
- Challenge the models: Discard questions that can be solved trivially — for example, by always returning the first proper noun;
- Good performance does not guarantee understanding: Probe data sets to ensure models are not taking short cuts;
- Include variations: Add variations to existing questions to check model flexibility;
- Standardize data set formats: Consider following a standard format when releasing new data sets, as this makes cross-data-set experimentation easier. We offer some help in this regard by releasing code that converts the five data sets in our experiments into a shared format.