What makes two images similar? The question is of vital importance for the training of computer vision systems, but it’s notoriously difficult to answer. That’s because, for a human observer, the similarity of two images is not just visual but conceptual: images whose pixel patterns are very different may nonetheless express the same concept.
In a paper we presented at this year’s Computer Vision and Pattern Recognition Conference (CVPR), we propose a method for measuring the conceptual distance between two images. Our method uses a large vision-language model in two ways: first, we use it to generate multiple descriptions of each image, at different lengths; then we use it to compute the probability that each description refers to either image.
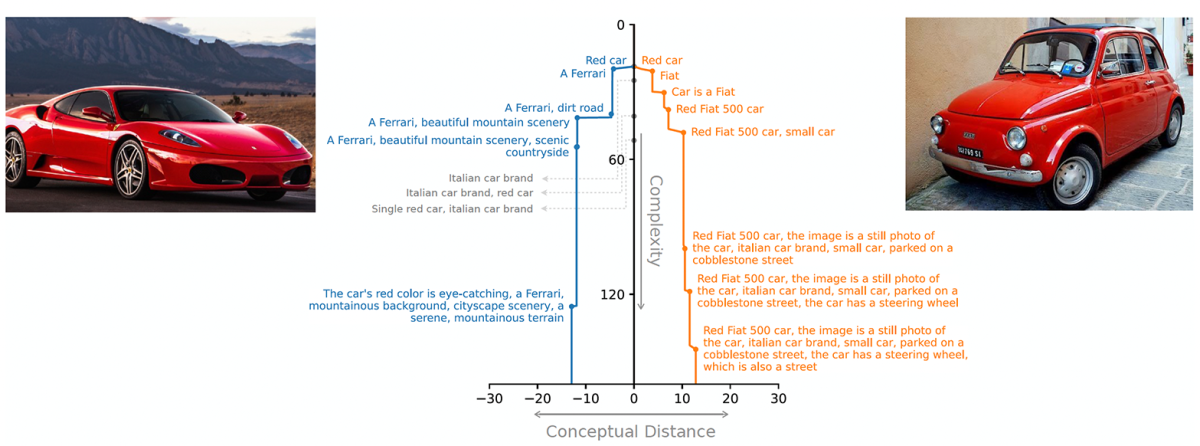
The core idea is to assess discriminability as a function of description length: if two images are easily discriminated by short descriptions, they’re not very similar, but if it takes a lot of text to reliably distinguish one from the other, they must be similar. And because our method relies on natural-language descriptions of increasing granularity, it’s also explainable: it’s easy for a human observer to determine exactly why the images received the similarity scores they did.
To evaluate our method, we compared it to the state-of-the-art technique for measuring image similarity, which uses contrastive-learning embeddings, on two different datasets, in which human annotators had scored pairs of images according to similarity. On both datasets, our method better predicted the human annotations, by an average of 9%.
Conceptual similarity
Defining a conceptual-distance metric faces three main challenges:
- Randomness dominates: Any two images will have a large number of small differences that predominate over structural similarities, so mapping conceptual similarity onto similarity in pixel values is difficult.
- No canonical properties: Which properties of an image are important for conceptual similarity can’t be specified a priori: sometimes the color of an object, the location of a scene, or the font of a text may be irrelevant; sometimes it may be essential.
- Adversarial discriminability: Someone trying to thwart a similarity detector might make cosmetic changes to an image — say, changing the color or orientation of particular objects or figures — in the hopes that enough such differences will decrease the similarity measure. A good metric needs to be resilient against such adversarial techniques.
Our method addresses all these difficulties. Because it begins by constructing accurate descriptions of the images and only then considers differences between descriptions, it provides no elementary notion of discriminability that an adversary could game, as in (3). And because those descriptions start out short, they perforce ignore the random variation identified in (1).
Our paper pays a little more attention to challenge (2). It may be intuitive that conceptual similarity has no canonical properties, but we formally prove the point. Essentially, we show that if a method enumerates enough image properties to identify any instance of conceptual similarity, then it will enumerate so many properties as to find similarities between any two samples it considers, rendering the concepts of similarity and difference empty.
By choosing natural language as our medium of comparison, however, we sidestep the question of canonical definitions of structure: natural language is flexible enough to accommodate any similarities between images.
The model
In our model, we begin with a space of hypotheses and a space of images; in practice, we use natural-language descriptions as our hypotheses, but the model can accommodate any other choice, so long as the hypothesis has an associated notion of length, akin to the notion of program length in Kolmogorov complexity.
Next, we define a decoder that computes the probability that a given hypothesis refers to a given image. Again, the model is agnostic as to choice of decoder, but in practice, we use a large vision-language model.
Our notion of conceptual similarity depends on how well we can describe an image using natural-language hypotheses of various lengths. The rate of improvement as the descriptions get longer reflects the images’ conceptual content. Random images would require long strings to describe them well enough to distinguish them from each other. On the other hand, “A bulldog wearing a pink tutu and riding a unicycle”, while unusual, is not very random because it can be succinctly described. When longer descriptions cease to improve our target-image likelihood by some margin, then we can say that we have captured all the conceptual (non-random) information in the image.
For a given hypothesis length, we would like to find the description that maximizes the target-image likelihood. The space of possible descriptions, however, is huge, so it can’t be efficiently searched, and it’s discrete, so it can’t be explored through gradient descent. We thus relax the optimality requirement slightly, instead identifying a distribution of hypotheses of bounded length that are likely descriptions of the target. This turns the challenge of discovering effective descriptions into a tractable optimization problem.
We can now define our distance metric. Given two images, A and B, and, for each image, a near-optimal description of a given length, we first compute the probabilities that the A hypothesis describes both images, A and B; then we take the difference between those probabilities. We repeat this process for the B hypothesis. The average of the two differences is the conceptual distance between the images for that particular hypothesis length.
Our metric is based on the rate at which that distance changes with hypothesis length. A slow rate of change indicates similarity: the images are hard to distinguish; a fast rate of change indicates that they’re easy to distinguish. Consequently, when it’s necessary to use a single value to score the similarity of two images, we use the area under the curve of the distance function over a range of hypothesis lengths.
Although our experiments validate the utility of our approach, at present, we use only the vision-language model’s text outputs to measure distance. It may be that directly measuring visual properties will provide an added layer of discrimination, without, hopefully, courting the dangers of sensitivity to randomness (1 above) or adversarial manipulation (3). We’re exploring that possibility in ongoing work.