Question answering (QA) is the machine learning task of learning to predict answers to questions. For example, given the question, “Where was Natalie Portman born?”, a QA model could predict the answer “Jerusalem”, using articles from the web, facts in a knowledge graph, or knowledge stored within the model. This is an example of a simple question, since it can be answered using a single fact or a single source on the web, such as the Natalie Portman Wikipedia page.
Not all questions are simple. For example, the question “What movie had a higher budget, Titanic or Men in Black II?” is a complex question because it requires looking up two different facts (Titanic | budget | 200 million USD and Men in Black II | budget | 140 million USD), followed by a calculation to compare values (200 million USD > 140 million USD).
While many state-of-the art question-answering models get good performance on simple questions, complex questions remain an open problem. One reason is a lack of datasets. Most existing QA datasets are large but simple, complex but small, or large and complex but synthetically generated, so they are less natural. A majority of QA datasets are also only in English.
To help bridge this gap, we have publicly released a new dataset: Mintaka, which we describe in a paper we're presenting at this year’s International Conference on Computational Linguistics (COLING).
Mintaka is a large, complex, natural, and multilingual question-answer dataset with 20,000 questions collected in English and professionally translated into eight languages: Arabic, French, German, Hindi, Italian, Japanese, Portuguese, and Spanish. We also ground Mintaka in the Wikidata knowledge graph by linking entities in the question text and answer text to Wikidata IDs.
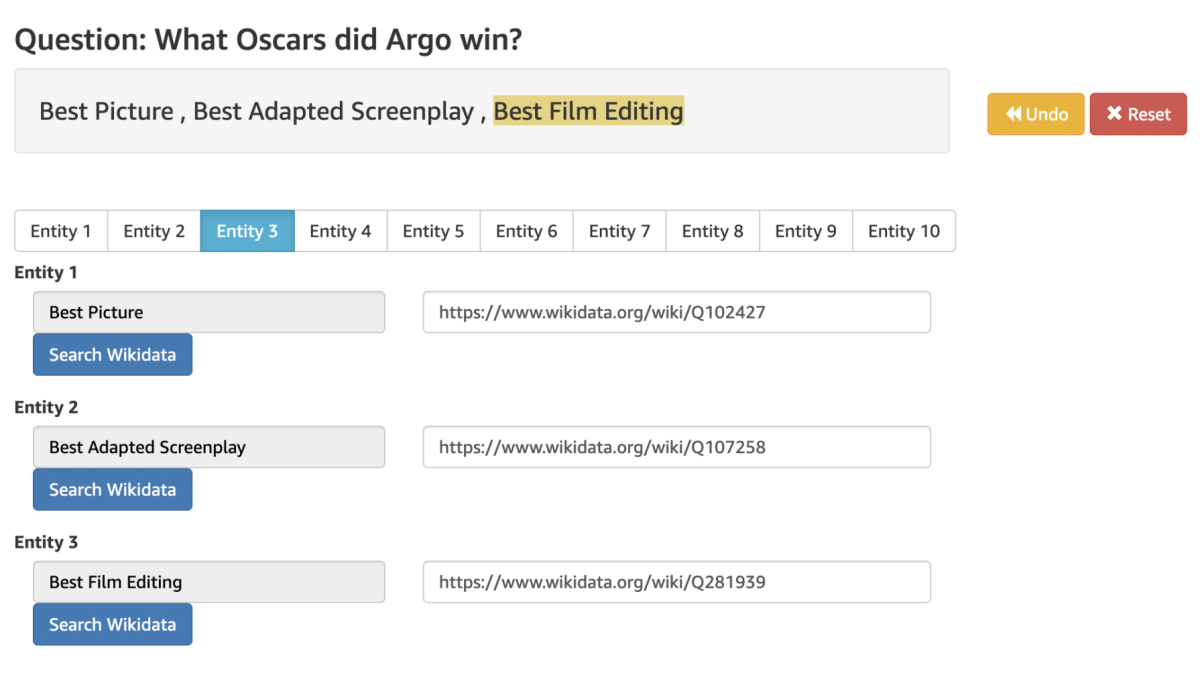
Building the dataset
We define complex questions as any question that requires an operation beyond a single fact lookup. We built Mintaka using the crowdsourcing platform Amazon Mechanical Turk (MTurk). First, we designed an MTurk task to elicit complex but natural questions. We asked workers to write question-answer pairs with one of the following complexity types:
- Count (e.g., Q: How many astronauts have been elected to Congress? A: 4)
- Comparative (e.g., Q: Is Mont Blanc taller than Mount Rainier? A: Yes)
- Superlative (e.g., Q: Who was the youngest tribute in the Hunger Games? A: Rue)
- Ordinal (e.g., Q: Who was the last Ptolemaic ruler of Egypt? A: Cleopatra)
- Multi-hop (e.g., Q: Who was the quarterback of the team that won Super Bowl 50? A: Peyton Manning)
- Intersection (e.g., Q: Which movie was directed by Denis Villeneuve and stars Timothee Chalamet? A: Dune)
- Difference (e.g., Q: Which Mario Kart game did Yoshi not appear in? A: Mario Kart Live: Home Circuit)
- Yes/No (e.g., Q: Has Lady Gaga ever made a song with Ariana Grande? A: Yes.)
- Generic (e.g., Q: Where was Michael Phelps born? A: Baltimore, Maryland)
Question-answer pairs were limited to eight categories: movies, music, sports, books, geography, politics, video games, and history. They were collected as free text, with no restrictions on what sources could be used.
Next, we created an entity-linking task where workers were shown question-answer pairs from the previous task and asked to either identify or verify the entities in either the question or answer and provide supporting evidence from Wikipedia entries. For example, given the question “How many Oscars did Argo win?”, a worker could identify the film Argo as an entity and link to its Wikidata URL.
Examples of Mintaka questions are shown below:
Q: Which Studio Ghibli movie scored the lowest on Rotten Tomatoes?
A: Earwig and the Witch
Q: When Franklin D. Roosevelt was first elected, how long had it been since someone in his party won the presidential election?
A: 16 years
Q: Which member of the Red Hot Chili Peppers appeared in Point Break?
A: Anthony Kiedis
Results
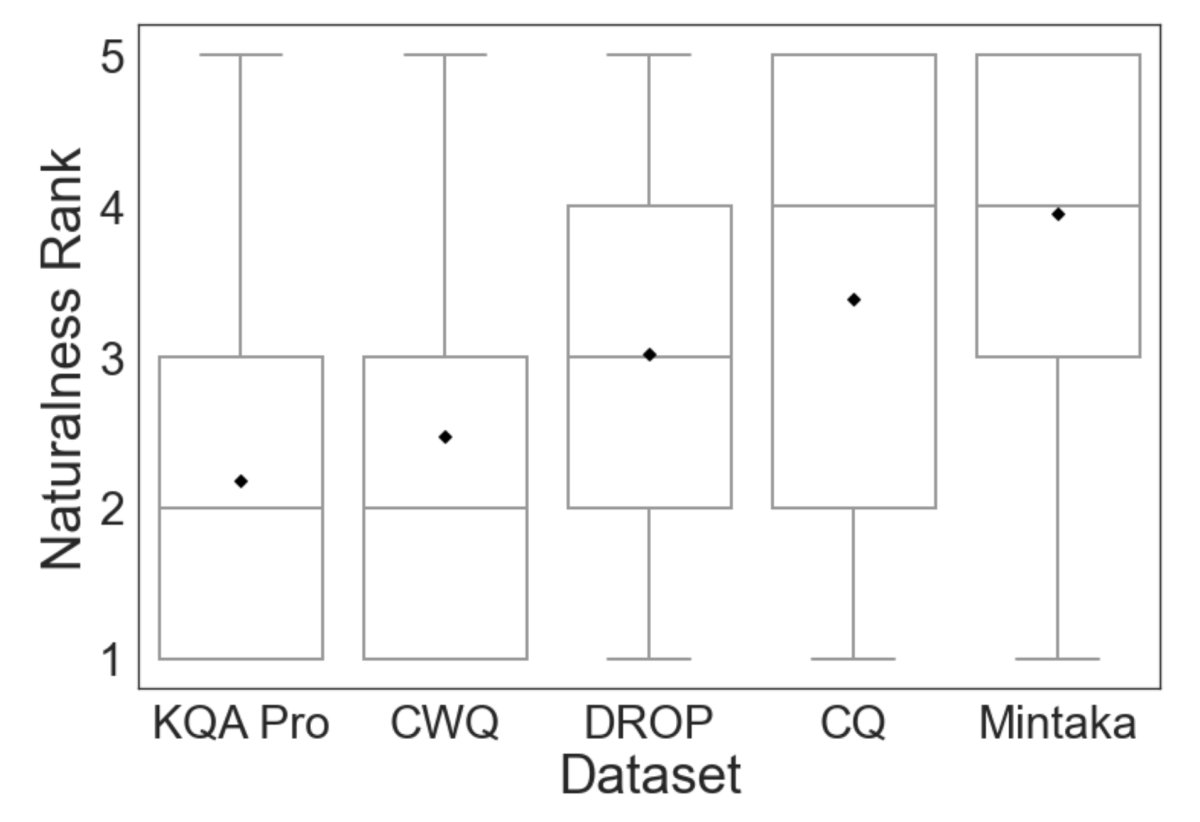
To see how Mintaka compares to previous QA datasets in terms of naturalness, we ran an evaluation on MTurk with four comparison datasets: KQA Pro, ComplexWebQuestions (CWQ), DROP, and ComplexQuestions (CQ). Workers were shown five questions, one from each dataset, and asked to rank them from 1 (least natural) to 5 (most natural). On average, Mintaka ranked higher in naturalness than the other datasets. This shows that Mintaka questions are perceived as more natural than automatically generated or passage-constrained questions.
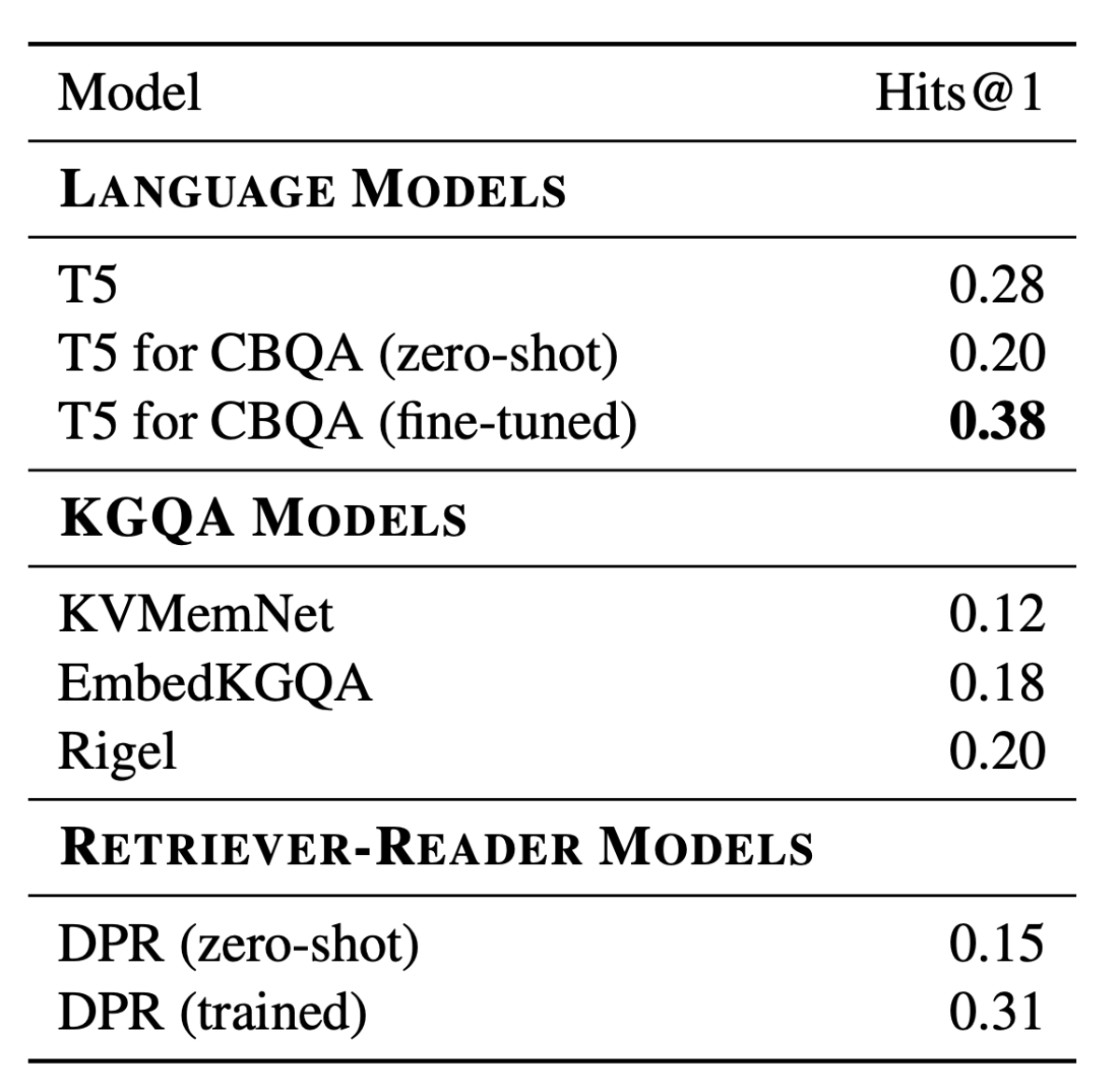
We also evaluated eight baseline QA models trained using Mintaka. The best-performing was the language model T5 for Closed Book QA, which scored 38% hits@1. The baselines show that Mintaka is a challenging dataset, and there is ample room for improving model design and training procedures.
Mintaka bridges a significant gap in QA datasets by being large-scale, complex, naturally elicited, and multilingual. With the release of Mintaka, we hope to encourage researchers to continue pushing question-answering models to handle more-complex questions in more languages.