The International Conference on Acoustics, Speech, and Signal Processing (ICASSP), which wrapped up late last month, focuses, as its name suggests, on applications close to the acoustic speech signal, such as automatic speech recognition and text-to-speech.
But in recent years, the line between speech processing and natural-language understanding (NLU) — which focuses on texts’ semantic content — has grown fuzzier, and Alexa AI scientists had several papers on NLU at ICASSP.
Among the most common NLU tasks are domain classification, or determining the topic of an utterance, and intent classification, or determining the speaker’s goals. Usually, NLU models are trained on data labeled according to both domain and intent.
But in “ADVIN: Automatically discovering novel domains and intents from user text utterances”, Alexa AI researchers present a new method for automatically identifying and categorizing domains and intents that an NLU model has never seen before. In the researchers’ experiments, it significantly outperformed its predecessors.
In many contexts, NLU can be improved through personalization. If two different customers tell a smart device “Play funny videos”, for instance, they may have very different types of content in mind.
Personalization based on interaction histories is well studied, but in the real world, interaction histories are constantly being updated, revealing new aspects of a customer’s taste or, indeed, changes of taste. In “Incremental user embedding modeling for personalized text classification”, Alexa AI researchers present a new approach to dynamically updating personalization models to reflect recent transactions. In tests on two different datasets, the approach improved prediction accuracy by 9% and 30%, respectively, versus the state of the art.
A third Alexa AI paper, “Contrastive knowledge graph attention network for request-based recipe recommendation”, narrows in on the very particular problem of matching online recipes to customer requests. The problem with conventional machine learning approaches to recipe retrieval is that data on customer interactions with recipes is noisy and sparse.
The Alexa AI researchers use graphs to add structure to the data and contrastive learning to improve the resulting model’s reliability, improving on the state of the art by 5% to 7% on two different metrics and two different datasets.
Christophe Dupuy, an applied scientist in Alexa AI, described two other ICASSP papers that he coauthored, on privacy-protecting machine learning and federated learning, in a blog post we published before the conference.
Intent discovery
With voice agents like Alexa, domains are general high-level content categories, like Music or Weather, and intents are specific functions, like PlayMusic or GetTemperature.
In “ADVIN: Automatically discovering novel domains and intents from user text utterances”, the Alexa AI researchers tackle the problem of classifying previously unseen domains and intents in three stages.
In the first stage, a model simply recognizes that a dataset contains unfamiliar intents. This model is trained on labeled data for known intents and publicly available, labeled out-of-domain utterances, as a proxy for unlabeled data with unknown intents.
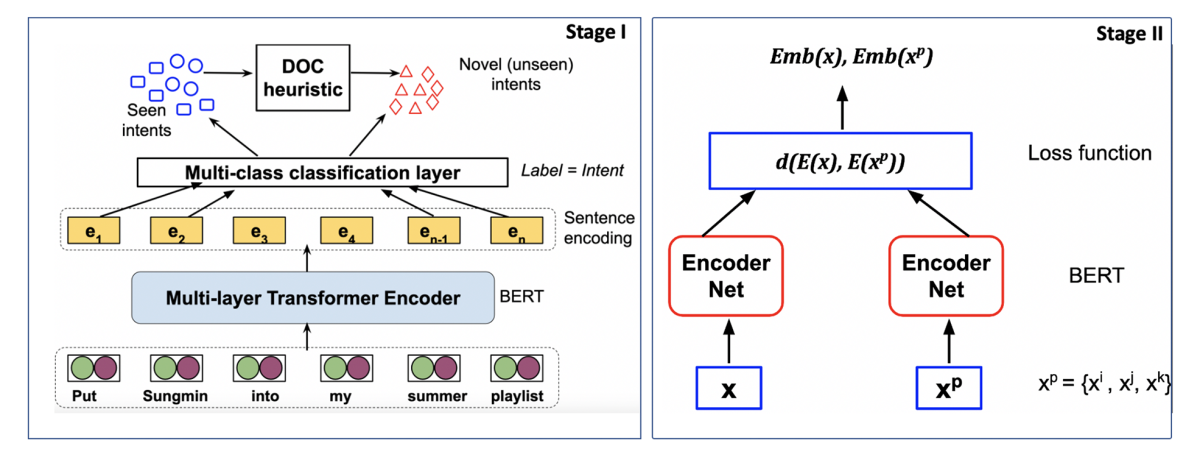
In the second stage, another model clusters both the labeled and unlabeled utterances, based on their semantic content. From the clusters of labeled intents, the researchers derive a threshold distance value that maximizes the model’s ability to distinguish intents. Then they apply that value to the unlabeled data, to identify clusters corresponding to new intents.
Finally, in the third stage, they repeat this process, but at a higher level of generality, clustering intents discovered in the previous stage into domains.
Dynamic personalization
Every interaction between a customer and an online service generates new data that could be used to update a profile that encodes the customer’s preferences, but it would be highly impractical to update the profile after each one of those interactions.
In “Incremental user embedding modeling for personalized text classification”, Alexa researchers instead propose keeping a running record of a customer’s most recent interactions and using that to update the customer’s preference in a dynamic way.
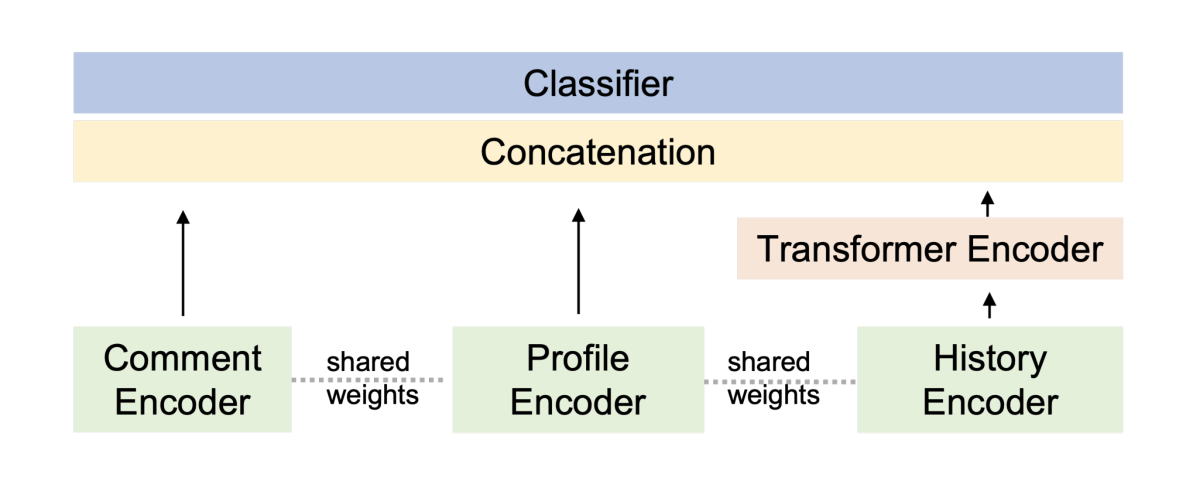
They present a machine learning model that takes as input the request that the NLU model is currently trying to resolve and representations of the customer’s long-term history and short-term history. An attention mechanism determines which aspects of the short-term history are most informative in light of the long-term history and vice versa.
The output of the attention mechanism is an ad hoc customer profile that the model can use to process the current request.
Recipe retrieval
“Contrastive knowledge graph attention network for request-based recipe recommendation” also addresses the question of personalization, although in the specific context of recipe recommendation — deciding which recipes to return, for instance, when the customer says, “Show me recipes for chicken breasts.”
Customers interact with recipe recommendation services in many different ways, such as browsing through recipes or checking ingredient lists. The most telling interaction, however — the one that proves that the recommended recipe met the customer’s needs — is a “cook along” service, that steps through the recipe to guide meal preparation.
Cook-along interactions are relatively rare, however, and the other types of interactions can be extremely noisy, reflecting stray clicks, misinterpretations of recipe titles, and the like. Building a reliable recipe recommendation service requires maximizing the high-value interaction data and filtering out the noise.
Graph-based models are a good way to do both, since they explicitly encode patterns in the data that would otherwise have to be inferred from training examples. The researchers begin by building a recipe graph, in which each node is a recipe, and recipes share edges if they belong to the same cuisine type, share ingredients, include related keywords, and so on.
Next, they add nodes representing customers to the graph. Edges between customer nodes and recipes indicate that customers have interacted with those recipes, and they also encode the types of interactions.
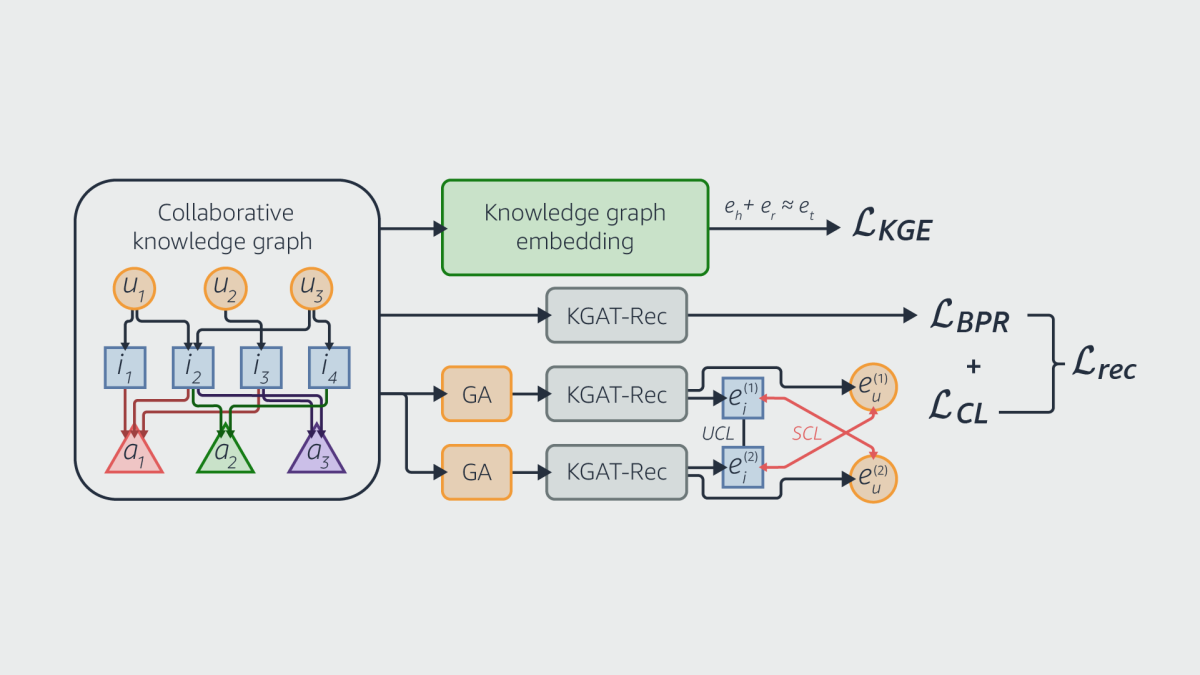
Finally, they train a model to create representations of the graph nodes using contrastive learning, in which the model is trained on pairs of examples, one that belongs to the target class — say, recipes that a particular customer has interacted with — and one that doesn’t. The model learns to produce representations that push contrasting examples far apart from each other in the representation space and pull related examples together.
To produce related examples, the researchers create synthetic variations on the real examples, in which nodes or edges have been randomly dropped. This trains the model to focus on essential features of the data and ignore inessential features, so it generalizes better.