Lantao Liu and his team at the Vehicle Autonomy and Intelligence Lab (VAIL) at Indiana University Bloomington want to help robots get better at navigating through complex and sometimes changing environments, while also boosting their ability to assess and process data. This challenge has significant applications, particularly in the realm of environmental modeling. Liu and his team are working to develop autonomous and machine learning methods and open-source libraries that can potentially benefit both the artificial intelligence and robotics communities.
“Machine learning algorithms are increasingly being developed for robotics missions. Many critical autonomy components are data-driven, where the data comes from onboard sensors such as LiDAR, sonar, and cameras,” says Liu who also is an assistant professor within the university’s Department of Intelligent Systems Engineering in the Luddy School of Informatics, Computing, and Engineering.
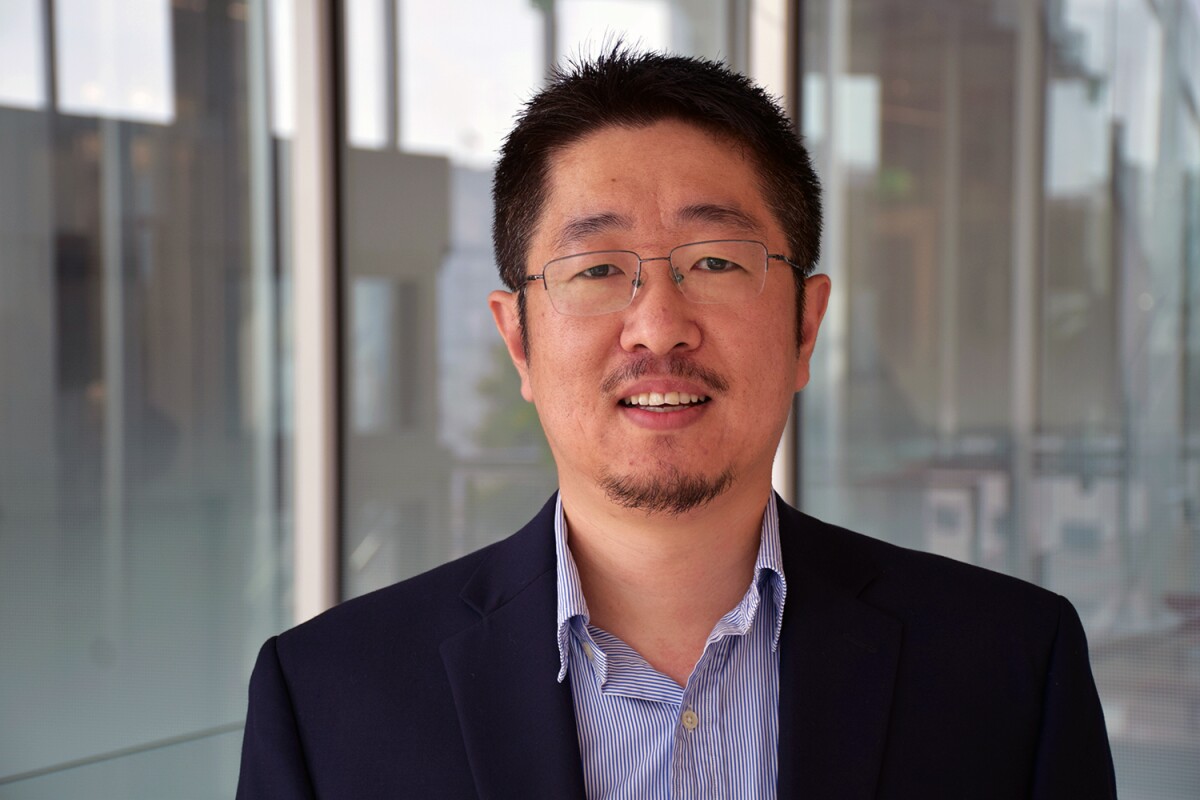
“The robots typically have weak computational capacity due to their limited dimensions and payloads, yet they require online learning with data processed on the fly,” he adds. “Unfortunately, many methods for solving these tasks entail large computational costs that can be very challenging for the robots. The key challenges have been computational-theoretical due to the increased complexity of stochastic modeling, but also practical due to the synergy of integrating hardware and software systems as well as customizing algorithms on the robots.”
Liu’s 2019 Amazon Machine Learning Research Award allows VAIL to access and leverage Amazon’s cloud computing tools and services for thousands of hours, boosting their work on both machine learning and autonomous systems.
“My lab works on various decision-making problems for different types of robots including aerial, ground, and aquatic vehicles. Our objective is to develop methodologies for autonomous robots to enhance their autonomy and intelligence in environmental sensing and modeling, search and rescue, among other applications of societal importance,” explains Liu.
Environmental sensing, modeling, and monitoring
One project being pursued by VAIL researchers involves a process that maps environmental attributes of interest, such as pollution in the water or air, by collecting corresponding measurement samples from different locations so that a “distribution map" (environment model) can be reconstructed.
“This mapping mechanism is also called environmental state estimation, a learning process where the parameters of an underlying environment model must be learned using streams of incoming sampling data collected by robots,” Liu explains.
“However, the environments can be dynamic, as can the associated environmental attributes to be mapped. A drawback to using robots is that the collection of samples requires a series of sequential, ordered, sampling operations (so data may not well represent the ground-truth map), and the entire sampling process is time consuming because the samples are typically spread over different spatial locations.
“To provide a good estimate of the state of the environment at any time, the robot information-gathering sensing must be persistent to keep up with evolving environmental dynamics,” Liu explains. “One focus of our research has been developing principles that use data-driven methods to guide robots to learn the spatio-temporal and stochastic environment model, and utilize the learned model for path planning and decision-making solutions. This, in turn, benefits future environmental exploration and exploitation for subsequent modeling and monitoring.”
The VAIL team has been developing methods and software that can accurately characterize the spatiotemporal environment by designing a non-stationary modeling framework based on a variant of Gaussian processes (GPs).
“The map will not be the same everywhere,” says Liu. “There are locations on the map that vary more rapidly than others, and we need to accurately model both rapidly and slowly changing parts. It is even more challenging when the underlying map is dynamic, such as when we’re mapping pollution dispersion.
“In addition,” he explains, “the model computation must be fast for in-the-moment decisions. However, sensing data is continuously received, and the accumulated data quickly overwhelms the robots’ computing resources. To boost the learning performance, our researchers recently developed an adaptive learning approach where the key idea is a sparse approximation mechanism that incrementally incorporates the new incoming data with a learned model supported by ‘summarized’ old data.”
Robotic anomaly detection
In a related project, the lab has been developing a generic robotic anomaly detection framework, motivated by field experiments.
“Commonly, robots in the field encounter sensing and behavioral anomalies,” Liu explains. “For example, one of the thrusters of the autonomous surface vehicle (ASV) might malfunction in operation, resulting in a forward motion becoming a turning motion. Or the ASV might get stuck in aquatic plants or other underwater obstacles, which are difficult to perceive using cameras or LiDARs. The inertial measurement unit (IMU) can be sensitive to external disturbances such as magnetic fields and provide drifting readings. Surrounding objects, such as a tall tree near the shore, might block the GPS signals, which leads to inaccurate localization. Sonar data can also be affected by dynamic underwater objects or environmental disturbances.
“Resilient and adaptive robotic systems require cognitive capabilities to avoid anomalies and recover and learn from failures with minimal human intervention,” Liu adds. “Equipping robots with the self-examination ability to detect sensing and behavioral faults is an essential step. The intuitive idea of anomaly detection is to develop some concept of normality and treat the observations that deviate considerably from that as anomalies.
“It is difficult, if not impossible, to handcraft a model representing the expected behaviors of different kinds of robots in various applications,” Liu explains. “The framework learns the concept of normality via deep representation learning and graph neural networks. We train the framework using contrastive learning in a semi-supervised manner that utilizes the information in a large amount of unlabeled data and, optionally, a small amount of labeled data. During the development of this framework, the AWS EC2 instances have drastically accelerated the prototyping, training, and testing processes. We are currently finalizing this framework and will open-source software.
“Hopefully,” he adds, “it will also benefit the robotics and machine learning communities at large.”
Off-road autonomy
The AWS Machine Learning Research Award also helps VAIL research off-road autonomy.
“An important challenge is the stochastic modeling of unexpected robot behaviors,” he explains. “Basically, the robots operating in real-world complex environments need to reason about the long-term results of their physical interactions with the environment, but due to the high complexity of the real world, it is generally impossible to predict future events in an accurate manner.
“For example,” says Liu, “the effect of uneven road conditions or various disturbances on the robot’s motion is hard to model (or learn from data) precisely. It is even more challenging to model the interaction between the robot and the environment, especially when the environment is dynamic. Other representative scenarios include drones flying with strong winds or submarines moving under ocean currents, where air and water flows vary significantly in both space and time.
“Thus, it is necessary for the robots to consider these epistemic uncertainties caused by a lack of precise modeling of the environment while making decisions,” he explains. “We use Markov decision process as a basis to model autonomous decision-making under uncertainty problems. The solution to these problems is a closed-loop policy that maximizes a long-term goal and satisfies the safety constraints under a probabilistic interaction model between the robot and the environment. In principle, the resulting policy can generate a sequence of motor commands that complete the task assigned by a human, given that the probabilistic model can well describe the uncertainty of the world, and the computational method can allow the robot to calculate the policy within a reasonable amount of time.
“However,” Liu continues, “many real-world problems are non-trivial, and obtaining the required probabilistic model of the world is generally impossible. Our research focuses on solving these two challenges by developing novel methods and leveraging the strong computational power of GPUs. Our current focus is on addressing the computational part of the challenge by developing two planning algorithms that allow the robot to reason about its continuous motion on complicated terrain surfaces based on the kernel method (mesh-free) and finite-element method (mesh-based). Both methods leverage a set of discrete elements to represent the value function over the continuous space. The computation over the discrete parts can be parallelized, which allows our robot to reason and compute optimal policies in real-time to navigate through complicated terrains safely and efficiently.”
VAIL researchers have been working on using sampling methods to optimize over a class of parameterized policies.
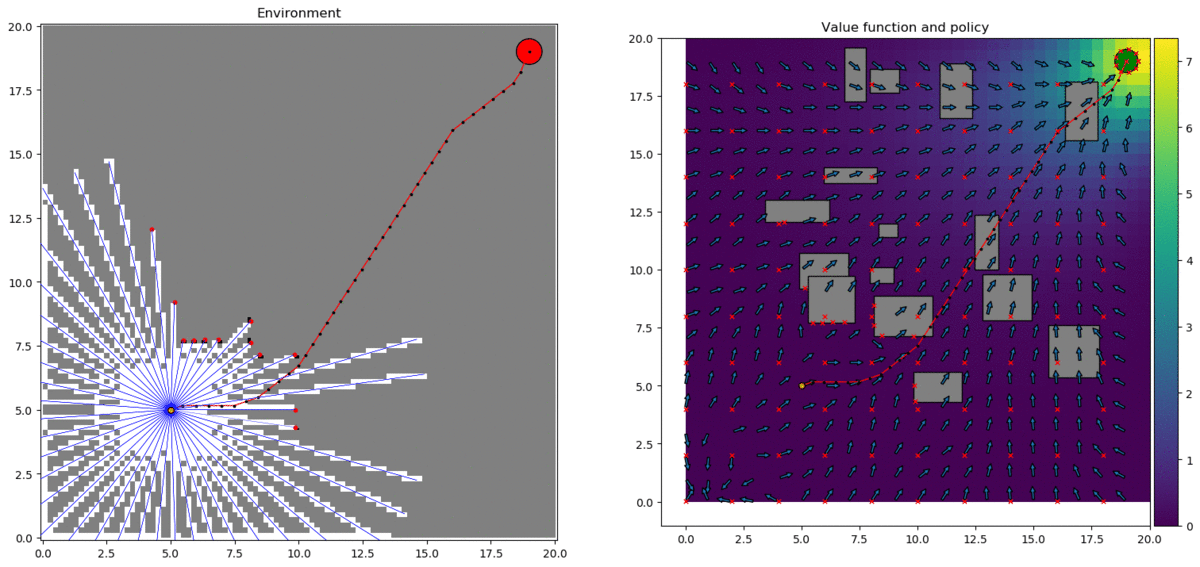
“To do so, we first need to sample a large number of robot trajectories under the current policy, which can be computed quickly by the parallel architecture of Nvidia GPU CUDA cores,” Liu explains. “They use the gradient-based method for optimization of policy parameters: the policy is updated by computing the policy parameter gradients based on the sampled trajectories. The gradient computation and policy update involve large matrix operations, which can also be parallelized by GPUs for real-time solutions. They leverage AWS computation for this task.”
Navigable space segmentation for navigation
Liu notes that the AWS resources have also been very useful for the team’s visual autonomy research. Visual information has become increasingly important for robotic autonomy as it can provide rich information about surrounding environments, and VAIL’s visual data processing capability has been significantly improved due to the breakthrough on deep neural networks (DNNs). To develop deep approaches to process the vision perception, the team needs to develop models with complicated learning architectures, huge volumes of data, as well as various training strategies.
“A crucial capability for mobile robots to navigate in unknown environments is to construct obstacle-free space where the robot could move without collision,” Liu explains. “Roboticists have been developing methods for detecting such free space with the ray tracing of LiDAR beams to build occupancy maps in 2D or 3D space. Mapping methods with LiDAR require processing of large point cloud data, especially when a high-resolution LiDAR is used. As a much less expensive alternative, cameras have also been widely used for free space detection by leveraging DNNs to perform multi-class or binary-class segmentation of images.
“However,” he adds, “most existing DNN-based methods are built on a supervised-learning paradigm and rely on annotated datasets. The datasets usually contain a large amount of pixel-level annotated segmented images, which are prohibitively expensive and time-consuming to obtain for robotic applications in outdoor environments. To overcome limitations of fully supervised learning, we have been developing a new deep model based on variational auto-encoders. We target a representation learning-based framework to enable robots to learn navigable space segmentation in an unsupervised manner, with the aim of learning a polyline representation that compactly outlines the desired navigable space boundary. This is different from prevalent segmentation techniques which heavily rely on supervised learning strategies and typically demand immense pixel-level annotated images.
“We trained our model with the data from public datasets using GPUs,” Liu explains. “The large number of computing cores and memory space on AWS have enabled us to train our model fast and with high efficacy. This is crucial as it allows us to test and redesign models rapidly and provides great convenience to deploy the trained model to the robot systems.
“We then train our model with a small set of collected unlabeled images in real mission environments,” Liu adds. “Early testing shows that our model is able to detect navigable space in real time with high accuracy. “The computational resources provided by Amazon have greatly accelerated our design process.”