(Editor’s note: This is the fourth in a series of articles Amazon Science is publishing related to the science behind products and services from companies in which the Amazon Alexa Fund has invested. The Alexa Fund completed a strategic investment in Inpher, Inc., earlier this year; the New York and Swiss-based company develops privacy-preserving machine learning and analytics solutions that help organizations unlock the value of sensitive, siloed data to enable secure collaboration across organizations. This article is co-authored by Dimitar Jetchev, the cofounder and chief technology officer of Inpher, and Joan Feigenbaum, an Amazon Scholar and the Grace Murray Hopper professor of computer science at Yale University.)
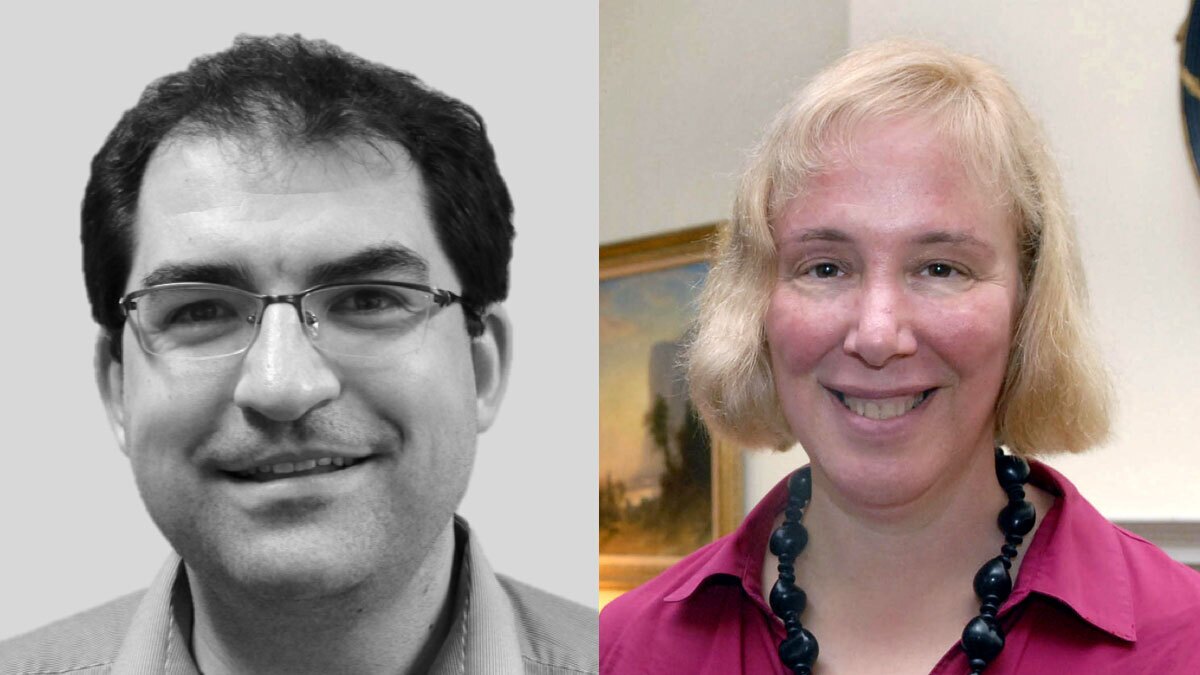
Machine learning (ML) is increasingly important in a wide range of applications, including market forecasting, service personalization, voice and facial recognition, autonomous driving, health diagnostics, education, and security analytics. Because ML touches so many aspects of our lives, it’s of vital concern that ML systems protect the privacy of the data used to train them, the confidential queries submitted to them, and the confidential predictions they return.
Privacy protection — and the protection of organizations’ intellectual property — motivates the study of privacy-preserving machine learning (PPML). In essence, the goal of PPML is to perform machine learning in a manner that does not reveal any unnecessary information about training-data sets, queries, and predictions.
Suppose, for example, that schools supplied encrypted student records to educational researchers who used them to train ML models. Suppose further that students, parents, teachers, and other researchers could feed encrypted queries to the models and receive encrypted predictions in return. By taking advantage of PPML techniques in this manner, all of the participants could mine the knowledge contained in educational-record databases without compromising the privacy of the data subjects or the data users.
PPML is a very active area, with an eponymous annual workshop and many strong papers in general-ML and security venues. Techniques have been developed for privacy-preserving training and prediction on a wide range of ML model types, e.g., neural nets, decision trees, and logistic-regression formulae.
In the sections below, we describe PPML methods for training and prediction in extreme gradient boosting.
Training
Gradient boosting is an ML method for regression and classification problems that yields a set of prediction trees, typically classification and regression trees (CARTs), which together constitute a model. A CART is a generalization of a binary decision tree; while a binary tree produces a binary output, classifying each input query as a “yes” or “no,” a CART assigns each input query a (real) numerical score.
Interpretation of scores is application dependent. If v is a query, then each CART in the model assigns a score to v, and the final prediction of the model on input v is the sum of these scores. In some applications, the softmax function may be used instead of sum to produce a probability distribution over the predicted output classes.
Extreme gradient boosting (XGBoost) is an optimized, distributed, gradient-boosting framework that is efficient, portable, and flexible. In this section, we consider confidentiality of training data in the creation of XGBoost models for disease prediction — specifically, for prediction of multiple sclerosis (MS).
Early diagnosis and treatment of MS is crucial to prevent degenerative progression of the disease and patient disabilities. A recent paper proposes an early-diagnosis method that applies XGBoost to electronic health records and uses three types of features: diagnostic, epidemiologic, and laboratory.
How cryptographic computing can accelerate the adoption of cloud computing
In a previous Amazon Science article, Joan Feigenbaum reviewed secure multiparty computation and privacy-preserving machine learning – two cryptographic techniques employed to address cloud-computing privacy concerns and accelerate enterprise cloud adoption.
The presence of another neurological disease (e.g., acute disseminated encephalomyelitis (ADEM)) is an example of a diagnostic feature. Epidemiologic features include age, gender, and total number of visits to a hospital. Two more features that are discovered by lab tests are used in the model and referred to as laboratory features: hyperlipidemia (abnormally elevated levels of any or all lipids) and hyperglycemia (elevated blood sugar). The proposed XGBoost model significantly outperforms other ML techniques (including naïve Bayes methods, k-nearest neighbor, and support vector machines) that have been proposed for early diagnosis of MS.
Collecting a sufficient number of high-quality data samples and features to train such a diagnostic model is quite challenging, because the data reside in different private locations. The training data can be split in different ways among these locations: horizontally split, vertically split, or both.
If the private data sources contain samples with the same feature set (as would be the case if, say, the same features are extracted from health records residing in different hospitals), the dataset is said to be horizontally split. The other extreme — vertically split data — occurs when a private data source contributes a new feature for all of the training samples. For example, a health-insurance company could supply reimbursement receipts for past medication (the new feature) to complement the features in clinical health records. In these scenarios, aggregating the training data on a central server violates GDPR regulations.
The figure below illustrates one possible CART in the trained model. The weights at the leaves might indicate probabilities of MS resulting from the various paths from root to leaf.
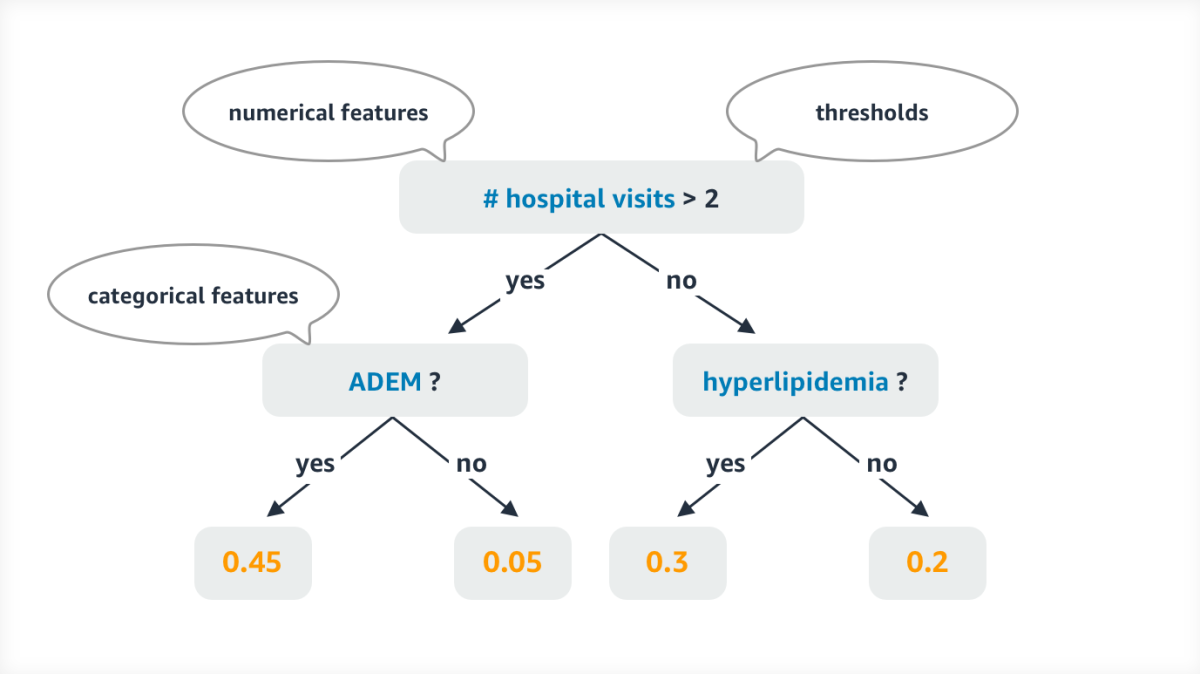
Research on privacy-preserving training of XGBoost models for prediction of MS uses two distinct techniques: secure multiparty computation (SMPC) and privacy-preserving federated learning (PPFL). We briefly describe both of them here.
An SMPC protocol enables several parties, each of whom holds a private input, to jointly evaluate a publicly known function on these inputs without revealing anything about the inputs except what is implied by the output of the function. Private inputs are secret shared among the parties, e.g., via additive secret sharing, in which each owner of a private input v generates random “shares” that add up to v.
For instance, suppose that Alice’s private input is v = 5. She can secret share it among herself, Bob, and Charlie by generating two random integers SBob =125621 and SCharlie = 56872, sending Bob’s share to him and Charlie’s to him, and keeping SAlice = v - SBob - SCharlie = -182488. Unless an adversary controls all three parties, he cannot learn anything about Alice’s private input v.
In an execution of an SMPC protocol, the inputs to each elementary operation (addition or multiplication) are secret shared, and the output of the operation is a set of secret shares of the result. We say that a secret-shared value y (which may be the final output of the computation) is revealed to party P if all the parties send their shares to P, thus enabling P to reconstruct y. Further discussion of SMPC and its relevance to cloud computing can be found here and in Inpher’s Secret Computing Explainer Series.
A recent paper by researchers at Inpher proposes an SMPC protocol, called XORBoost, for privacy-preserving training of XGBoost models. It improves the state of the art by several orders of magnitude and ensures that
- The CARTs computed by the protocol are secret shared among the training-data owners and revealed only to a designated party, namely the data analyst.
- The training algorithm not only protects the input data but also reveals no information about the paths in the CARTs taken by any of the training samples.
- XORBoost supports both numerical and categorical features, thus providing enough flexibility and generality to support the above model.
XORBoost works well for training datasets of reasonable size — hundreds of thousands of samples and hundreds of features. However, many real-world applications require training on more than a million samples. To achieve that type of scale, one can use federated learning (FL), which is an ML technique used to train a model on data samples held locally by multiple, decentralized edge devices without requiring the devices to exchange the samples.
FL differs from XORBoost mainly in that FL does not perform the entire training exercise on secret-shared values. Rather, each device trains a local model on its local data samples and sends its local model to one or more servers for aggregation. The aggregation protocol typically uses simple operations such as sum, average, and oblivious comparisons but no complex optimization.
If the server receives the plaintext local-model updates from all of the devices, it could, in principle, recover the local training-data samples using model-inversion attacks. SMPC and other privacy-preserving computational techniques can be applied to aggregate local models without revealing them to the server. See the diagram below for the overall architecture.
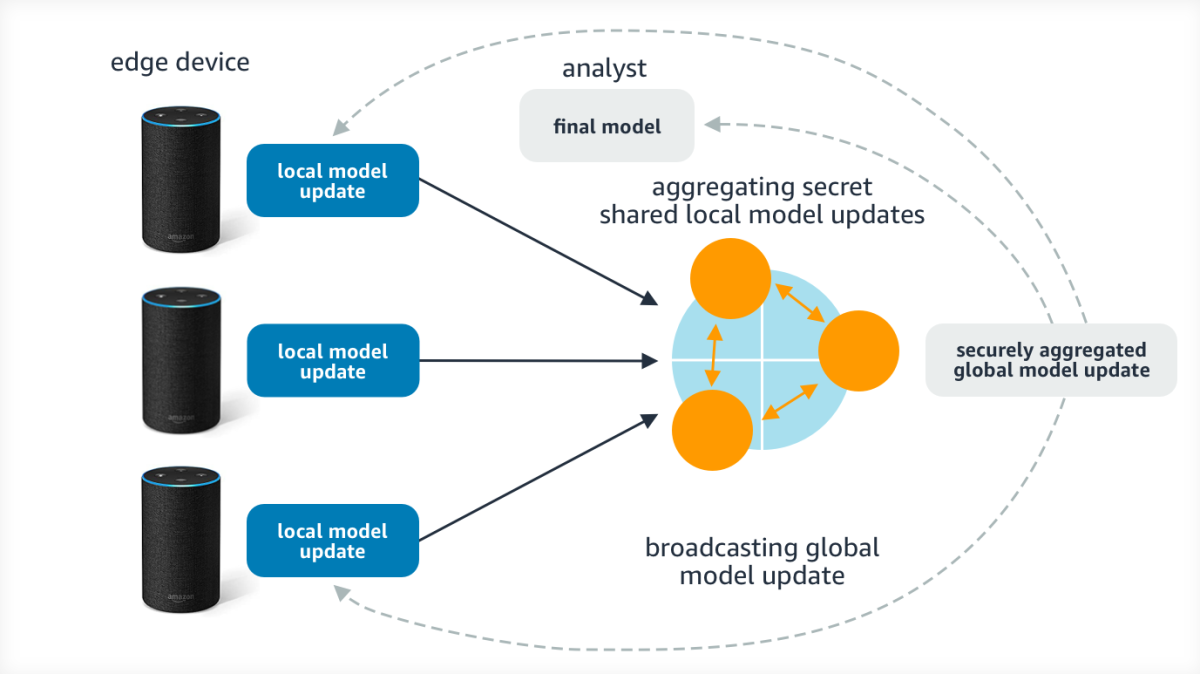
Prediction
PPXGBoost is a privacy-preserving version of XGBoost prediction. More precisely, it is a system that supports encrypted queries to encrypted XGBoost models. PPXGBoost is designed for applications that start by training a plaintext model Ω on a suitable training-data set and then create, for each user U, a personalized, encrypted version ΩU of the model to which U will submit encrypted queries and from which she will receive encrypted results.
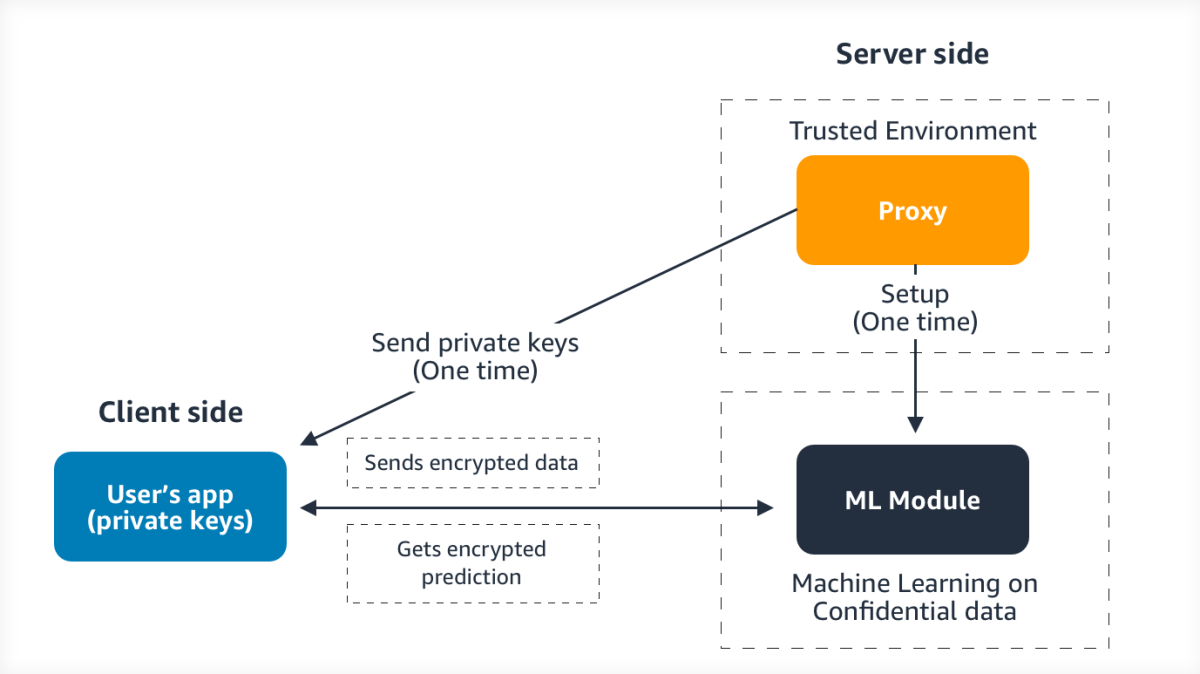
The PPXGBoost system architecture is shown in the figure above. On the client side, there is an app with which a user encrypts queries and decrypts results. On the server side, there is a module called Proxy that runs in a trusted environment and is responsible for setup (i.e., creating, for each authorized user, a personalized, encrypted model and a set of cryptographic keys) and an ML module that executes the encrypted queries. PPXGBoost uses two specialized types of encryption schemes (symmetric-key, order-preserving encryption and public-key, additive, homomorphic encryption) to encrypt models and evaluate encrypted queries. Each user is issued keys for both schemes during the setup phase.
Note that PPXGBoost is a natural choice for researchers, clinicians, and patients who wish to make disease predictions repeatedly as the patients’ circumstances change. Potentially relevant changes include exposure to new environmental factors, experimental treatment for another condition, or simply aging. An individual patient can create a personalized, encrypted version of a disease-prediction model and store it on a server owned by the medical center at which he is receiving treatment. Patient and physician can then use it to monitor, in a privacy-preserving manner, changes in the patient’s likelihood of contracting the disease.
Conclusion
We have described the use of PPML to address privacy challenges in XGBoost training and prediction. In a future post, we will elaborate on how privacy-preserving federated learning enables researchers to train more-complex ML models on millions of samples stored on hundreds of thousands of devices.