In his machine learning keynote at re:Invent on Tuesday, Swami Sivasubramanian, vice president of machine learning, Amazon Web Services (AWS), announced Amazon SageMaker Clarify, a new service that helps customers detect statistical bias in their data and machine learning models, and helps explain why their models are making specific predictions. Clarify saves developers time and effort by providing them the ability to better understand and explain how their machine learning models arrive at their predictions.
Developers today contend with both increasingly large volumes of data, as well as more complex machine learning models. In order to detect bias in those complex models and data sets, developers must rely on open-source libraries replete with custom code recipes that are inconsistent across machine learning frameworks. This tedious approach requires a lot of manual effort and often arrives too late to correct unintended bias.
“If you care about this stuff, it's pretty much a roll-your-own situation right now,” said University of Pennsylvania computer science professor and Amazon Scholar Michael Kearns, who provided guidance to the team of scientists that developed SageMaker Clarify. “If you want to do some practical bias detection, you either need to implement it yourself or go to one of the open-source libraries, which vary in quality. They're frequently not well-maintained or documented. In many cases, it's just, ‘Here is the code we used to run our experiments for this academic paper, good luck.’”
SageMaker Clarify helps address the challenges of relying on multiple open-source libraries by offering robust, reliable code in an integrated, cloud-based framework.
Increasingly complex networks
The efficacy of machine learning models depends in part on understanding how much influence a given input has on the output.
“A lending model for consumer loans might include credit history, employment history, and how long someone has lived at their current address,” Kearns explained. “It might also utilize variables that aren't specifically financial, such as demographic variables. One thing you might naturally want to know is which of these variables is more important in the model’s predictions, which may be used in lending decisions, and which are less important.”
With linear models, each variable is assigned some weight, positive or negative, and the overall decision is a sum of those weighted inputs. In those cases, the inputs with the bigger weights clearly have more influence on the output.
However, that approach falls short with neural networks or more complicated, non-linear models. “When you get to models like neural networks, it's no longer a simple matter of determining or measuring the influence of an input on the output,” Kearns said.
To help account for the growing complexity of modern machine learning models, the Amazon science team looked to the past — specifically to an idea from 1951.
Shapley values
The team wanted to design a solution to help machine learning pros be able to better explain their models’ decisions in the face of growing complexity. They found inspiration in a popular scientific method called Shapley values.
Shapley values were named in honor of Lloyd Shapley, who introduced the idea in 1951 and who won the Nobel Prize in Economics for it in 2012. The Shapley value approach, which is rooted in game theory, considers a wide range of possible inputs and outputs and offers “the average marginal contribution of a feature value across all possible coalitions”. The comprehensive nature of the approach means it can help provide a framework for understanding the relative weight of a set of inputs, even across complex models and multiple inputs.
“SageMaker Clarify utilizes Shapley values to essentially take your model and run a number of experiments on it or on your data set,” Kearns said. “It then uses that to help come up with a visualization and quantification of which of those inputs is more or less important.”
Nor does it matter which kind of model a developer uses. “One of the nice things about this approach is it is model agnostic,” Kearns said. “It performs input-output experiments and gives you some sense of the relative importance of the different inputs to the output decision.”
The science team also worked to be certain SageMaker Clarify had a comprehensive view. They designed it so everyday developers and data scientists can detect bias across the entire machine learning workflow — including data preparation, training, and inference. SageMaker Clarify is able to achieve that comprehensive view, Kearns explained, because (again) it is model agnostic. “Each of these steps has been designed to avoid making strong assumptions about the type of model that the user is building.”
Bias detection and explainability
Model builders who learn that their models are making predictions that are strongly correlated to a specific input may find those predictions fall short of their definition of fairness. Kearns offered the example of a lending company that discovers its model’s predictions are skewed. “That company will want to understand why its model is making predictions that might lead to decisions to give loans at a lower rate to group A than to group B, even if they're equally credit worthy.”
SageMaker Clarify can examine tabular data and help the modelers spot where gaps might exist. “This company would upload a spreadsheet of data showing who they gave loans to, what they knew about them, et cetera,” Kearns said. “What the data bias detection part does is say, ‘For these columns, there may be over or underrepresentation of certain features, which could lead to a discriminatory outcome if not addressed.’”
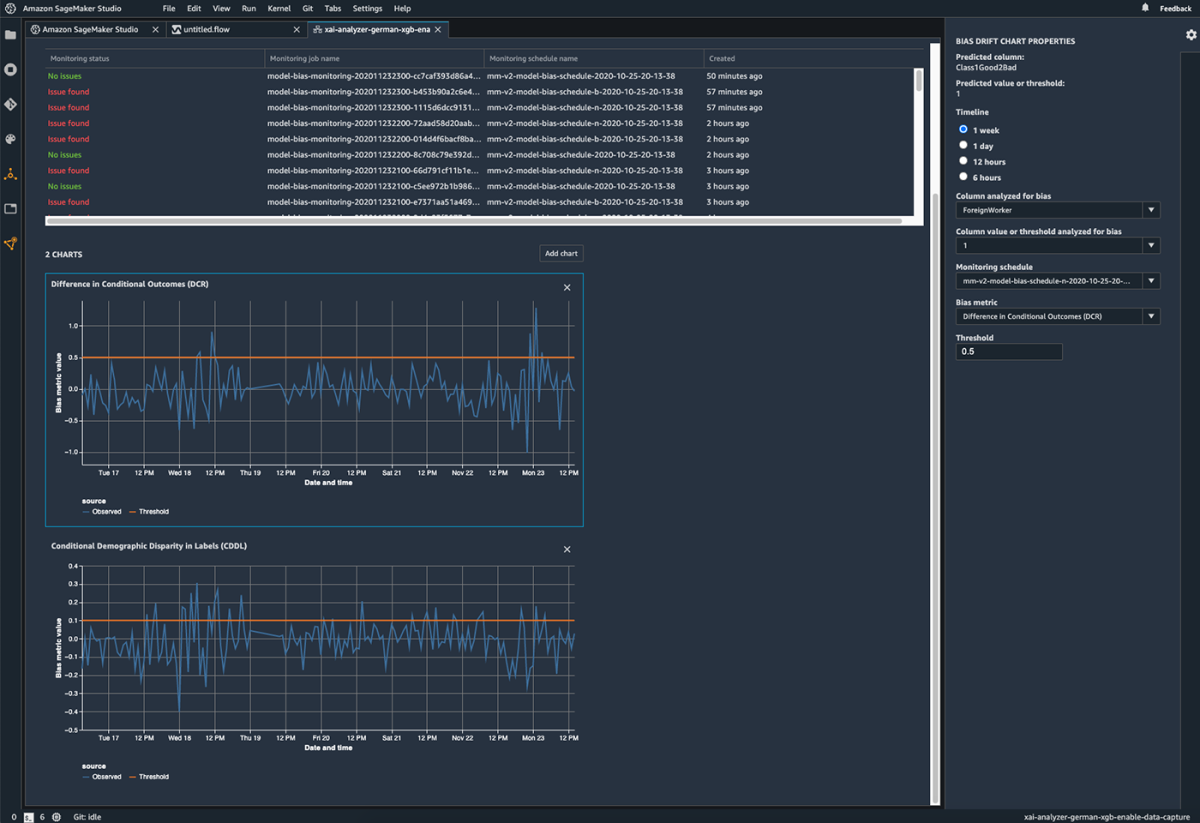
That can be influenced by a number of factors, including simply lacking the correct data to build accurate predictions. For example, SageMaker Clarify can indicate whether modelers have enough data on certain groups of applicants to expect an accurate prediction. The metrics provided by SageMaker Clarify can then be used to correct unintended bias in machine learning models, and automatically monitor model predictions in production to help ensure they are not trending toward biased outcomes.
Future applications
The SageMaker Clarify science team is already looking to the future.
Their research areas include algorithmic fairness and machine learning, as well as explainable AI. Team members have published widely in the academic literature on these topics, and worked hard in the development of SageMaker Clarify to balance the science of fairness with engineering solutions and practical product design. Their approaches are both statistical and causal, and focus not only on bias measurement in trained models, but also bias mitigation. It is that last part that has Kearns particularly excited about the future.
“The ability to not just identify problems in your models, but also have the tools to train them in a different way would go a long way toward mitigating that bias,” he said. “It’s good to know that you have a problem, but it's even better to have a solution to your problem.”
Best practices
The notions of bias and fairness are highly application dependent and the choice of the attributes for which bias is to be measured, as well as the choice of the bias metrics, may need to be guided by social, legal, and other non-technical considerations,” said principal applied scientist Krishnaram Kenthapadi, who led the scientific effort behind SageMaker Clarify. “For successful adoption of fairness-aware machine learning and explainable AI approaches in practice, it’s important to build consensus and achieve collaboration across key stakeholders such as product, policy, legal, engineering, and AI/ML teams, as well as end users and communities,” he said. “Further, it’s good to take into account fairness and explainability considerations during each stage of the ML lifecycle, for example, Problem Formation, Dataset Construction, Algorithm Selection, Model Training Process, Testing Process, Deployment, and Monitoring/Feedback.