On Dec. 1 at re:Invent 2020, Amazon Web Services (AWS) announced Amazon Lookout for Vision, an anomaly detection solution that uses machine learning to process thousands of images an hour to spot manufacturing defects and anomalies — with no machine learning experience required.
The new offering means manufacturers can send camera images to Lookout for Vision to identify defects, such as a crack in a machine part, a dent in a panel, an irregular shape, or an incorrect product color. Lookout for Vision also utilizes few shot learning, so customers can assess machine parts or manufactured products by providing small batches — sometimes as few as 30 images (10 images of defects or anomalies plus 20 “normal” images). Lookout for Vision then reports the images that differ from baselines so that appropriate action can be taken — quickly.
Because modern manufacturing systems are so finely tuned, defect rates are often 1% or less. However, even small defects can be enormously expensive in terms of replacements, refunds, or waning customer trust, so finding and flagging those missed defects remains critically important. And while the percentage of defects may be small, finding and identifying them is a significant challenge. A team of Amazon scientists and engineers with extensive experience in machine learning, deep learning, and computer vision tackled that challenge when developing Amazon Lookout for Vision.
Barath Balasubramanian, AWS principal product manager, Anant Patel, AWS senior program manager, and Joaquin Zepeda, AWS senior applied scientist, talked about the unique and complex challenges they faced, how they were able to overcome them — and how building a mock factory helped them achieve their vision.
The (many, many) challenges
“There are two predominant ways in which defects are spotted. One is human inspection. They've been doing that since time immemorial,” Balasubramanian said. “The other one uses machine vision systems, purpose-built systems that take a picture, have hard-coded rules around them, and don't learn along the way.”
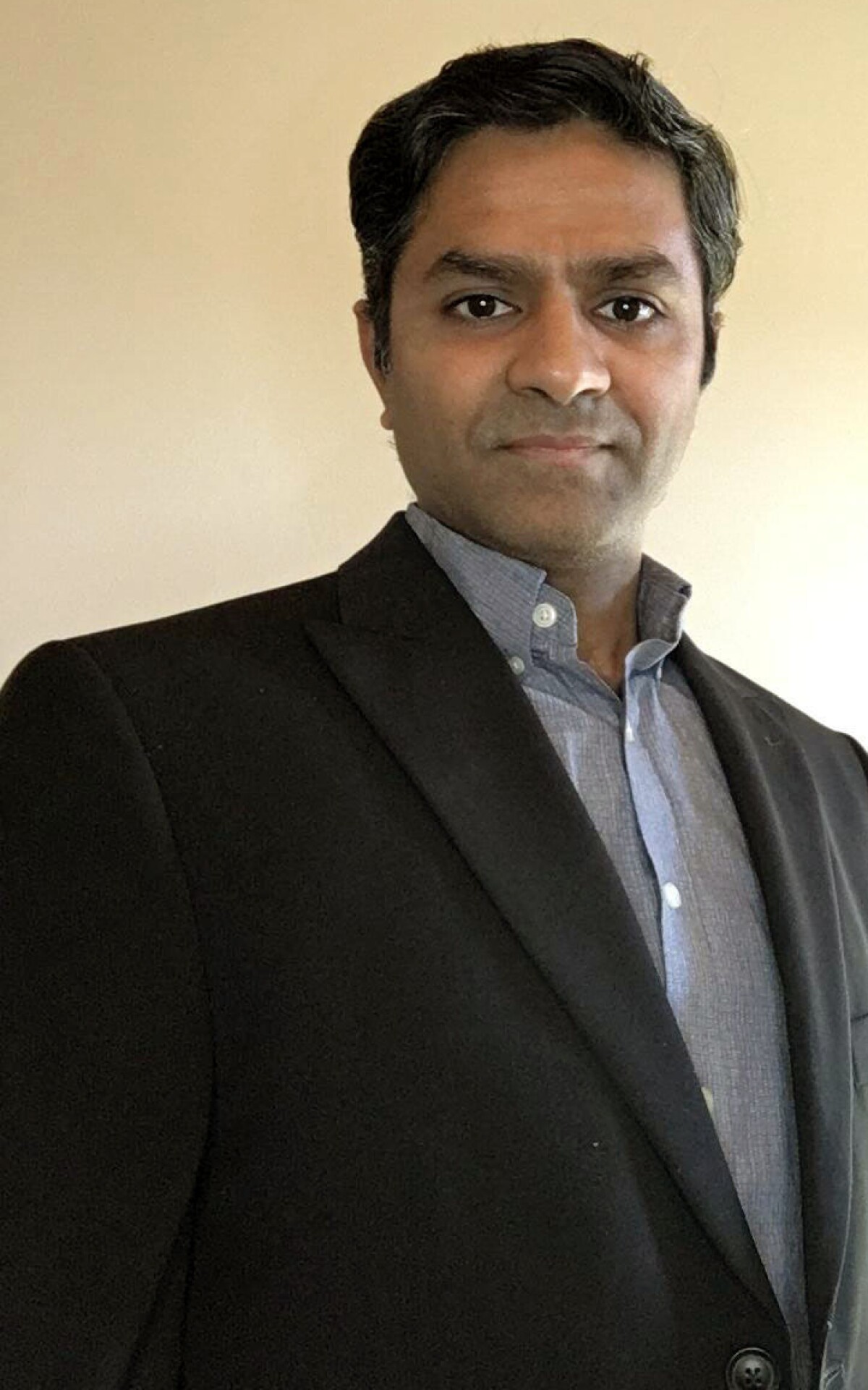
Apart from not being iterative, some companies lack the internal expertise to fine tune those systems for their specific environments. “It can take many months for an outside expert to come in, understand your environment, and set up rules,” Balasubramanian said. “Then you change one supplier part and the machine vision systems start saying, ‘This is a difference.’ Then you have to bring back that expert to recalibrate your system.”
In addition, everything from the manufacturing process, to the ways in which defects are identified, and even the defects themselves are influenced by a staggering number of variables.
“Not only do you have to consider the type of anomaly, but also the distribution of anomalies that you would find,” Zepeda said. “That's one big challenge that we've had to address with training models and with data collection. We worked with a customer that had defect rates in the 0.1% range, but those are the critical defects that must be found. As a result, the data we develop our system on should, as much as possible, reflect that distribution.”
Moreover, the scientists and engineers realized early on that the sample defects that they were training their models on didn’t match the shop-floor reality.
“We had examples of pretty obvious anomaly types, a huge scratch, a terrible box, and while that may happen in some objects or some use cases, the type of anomalies our customers are solving for are much more subtle,” Patel said.
As the teams of scientists and engineers considered the scope of the challenge facing them, they realized they had a data problem. “One of our challenges early on was around data, and whether we had enough data to really formulate a strong opinion about what the service should do,” Patel said.
Working with a third-party vendor who handles computer vision annotation tasks, the teams started exploring actual factories.
“We were trying to capture data in the manufacturing space, to bootstrap some of the data collection process,” Patel said. “And then we had a Think Big idea.”
A mock solution
The teams realized that their quest to find the sheer variety of data they required was going to require a unique approach. “Based on customer conversations, we knew we needed to replicate a production environment as closely as possible,” Patel said.
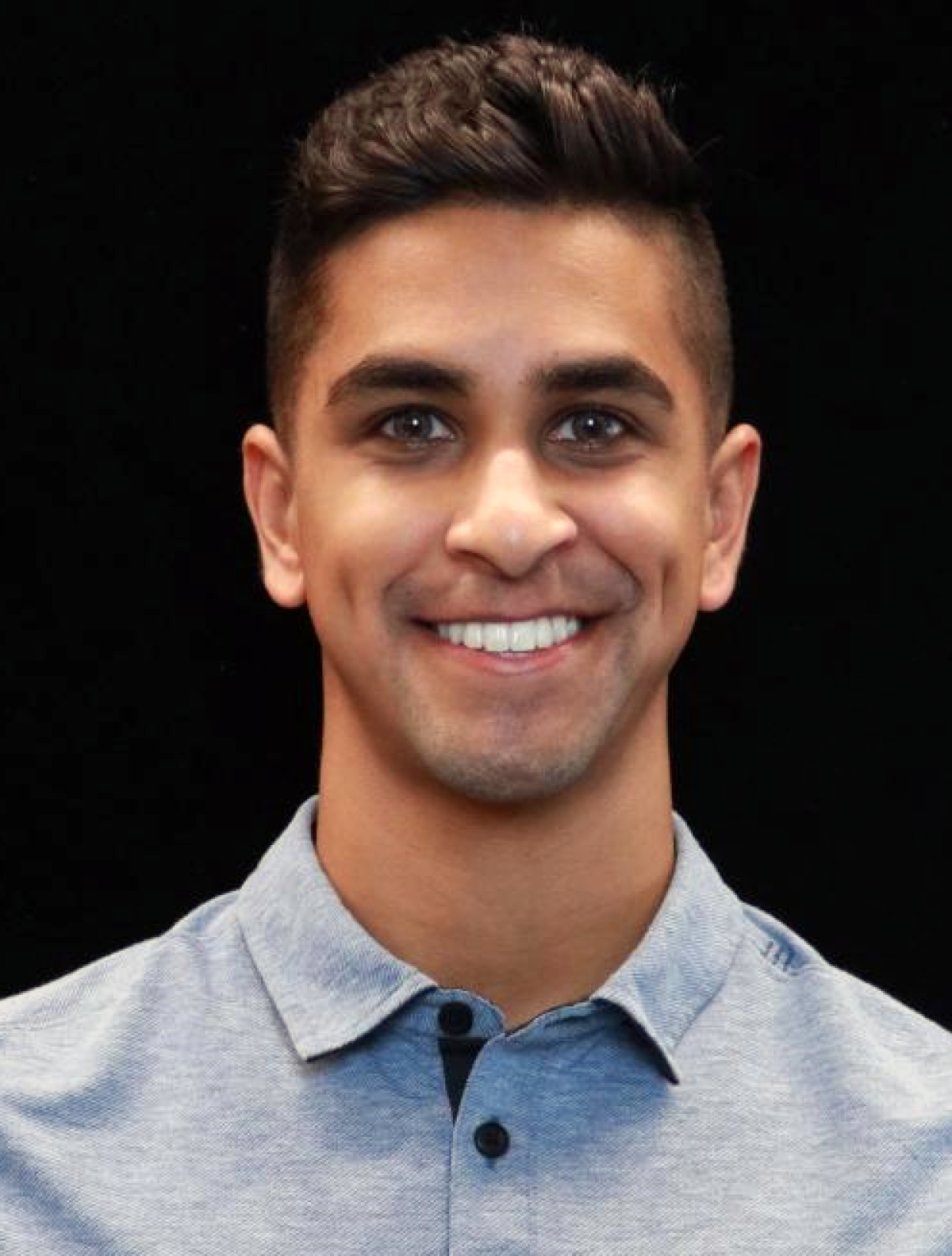
The solution: create a mock factory in India. The teams began procuring conveyor belts and cameras, and objects of various types to simulate various manufacturing environments. The goal: create data sets that included normal images and objects, and then draw or create synthetic anomalies — missing components, scratches, discolorations, and other effects.
“We had multiple cameras of different qualities because we wanted to account for things like RGB, grayscale, and cameras with different price points,” Zepeda said. “The conveyor belt had multiple variations that we could try, for example, by changing the belt texture or the belt color.”
“We were also trying to solve for, or monitor, lighting conditions, distance to the object, camera in a fixed position, things that customers would realistically try to implement themselves,” Patel added.
Fine tuning the mock factory so its outputs were useful also necessitated a lot of collaboration.
“Trying to bridge the gap between science and engineering was an iterative process,” Patel said. “I think we started with five to ten training data sets. We would review them with the science team who inevitably would have feedback on what was useful, or not. We learned a lot in the process.”
This is where the team’s application of few-shot learning (classifying data with as little training as possible) came in handy too, allowing them to occasionally work with no images of defects at all.
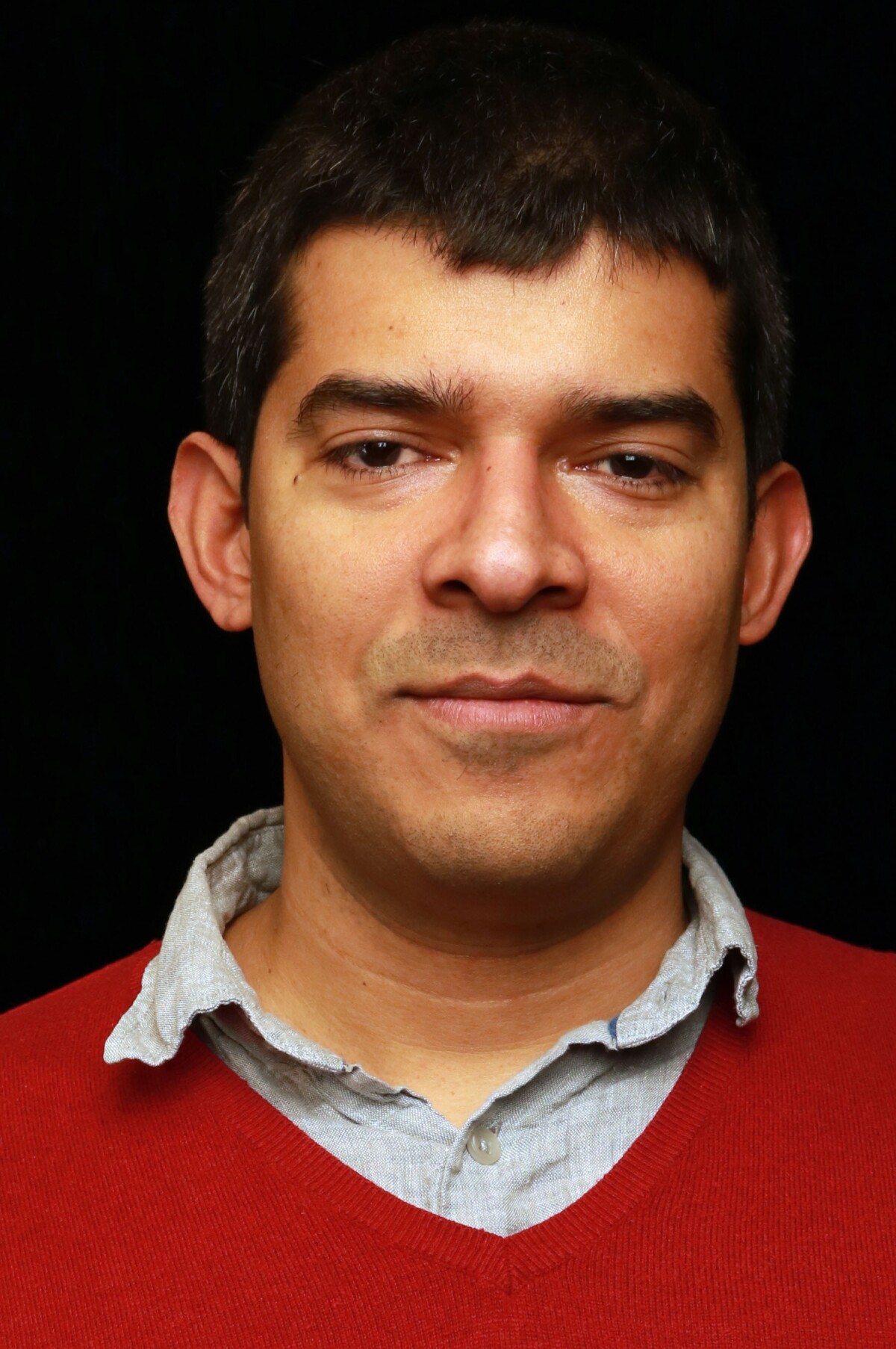
“Anomalies in manufacturing are intrinsically infrequent and thus difficult to source when assembling a training set,” Zepeda said. “Our models can be trained with only normal images to address this difficulty. The resulting models can be deployed or used to mine for anomalies in unlabeled collections of images using our ‘trial detection’ functionality to expand the training set.”
That real-life, trial-and-error iterative process eventually led to the development of Lookout for Vision — and that process of learning has only just begun.
“We realize that this launch really represents a beginning, not an end. Once deployed, we know we’ll confront unique situations that will challenge the service and we’ll learn from,” Patel said. “Lookout has to be versatile enough to generalize to all sorts of applications; it will get even better over time. The more feedback we get from our customers across the launch cycle, the more knowledge we’ll gain, and the better the system will perform.”