Amazon’s more than 40 papers at this year’s Conference on Empirical Methods in Natural-Language Processing (EMNLP) — including papers accepted to EMNLP’s new industry track — cover some familiar topics, such as natural-language understanding and question answering. But they also wander farther afield, taking in such disparate subjects as robotics and geospatial learning — and two of the papers concern pun generation.
Query rewriting, whose applications include self-learning and reference resolution, has emerged as a dynamic area of research at Amazon, with five related papers at this year’s EMNLP. And several papers explore the burgeoning field of prompt engineering, or priming large language models to produce the desired types of output.
Below is a quick guide to Amazon’s EMNLP papers, both academic track and industry track.
Continual learning
Iterative stratified testing and measurement for automated model updates
Elizabeth Dekeyser, Nicholas Comment, Shermin Pei, Rajat Kumar, Shruti Rai, Fengtao Wu, Lisa Haverty, Kanna Shimizu
Towards need-based spoken language understanding model updates: What have we learned?
Quynh Do, Judith Gaspers, Daniil Sorokin, Patrick Lehnen
Unsupervised training data reweighting for natural language understanding with local distribution approximation
Jose Garrido Ramas, Thu Le, Bei Chen, Manoj Kumar, Kay Rottmann
Dialogue
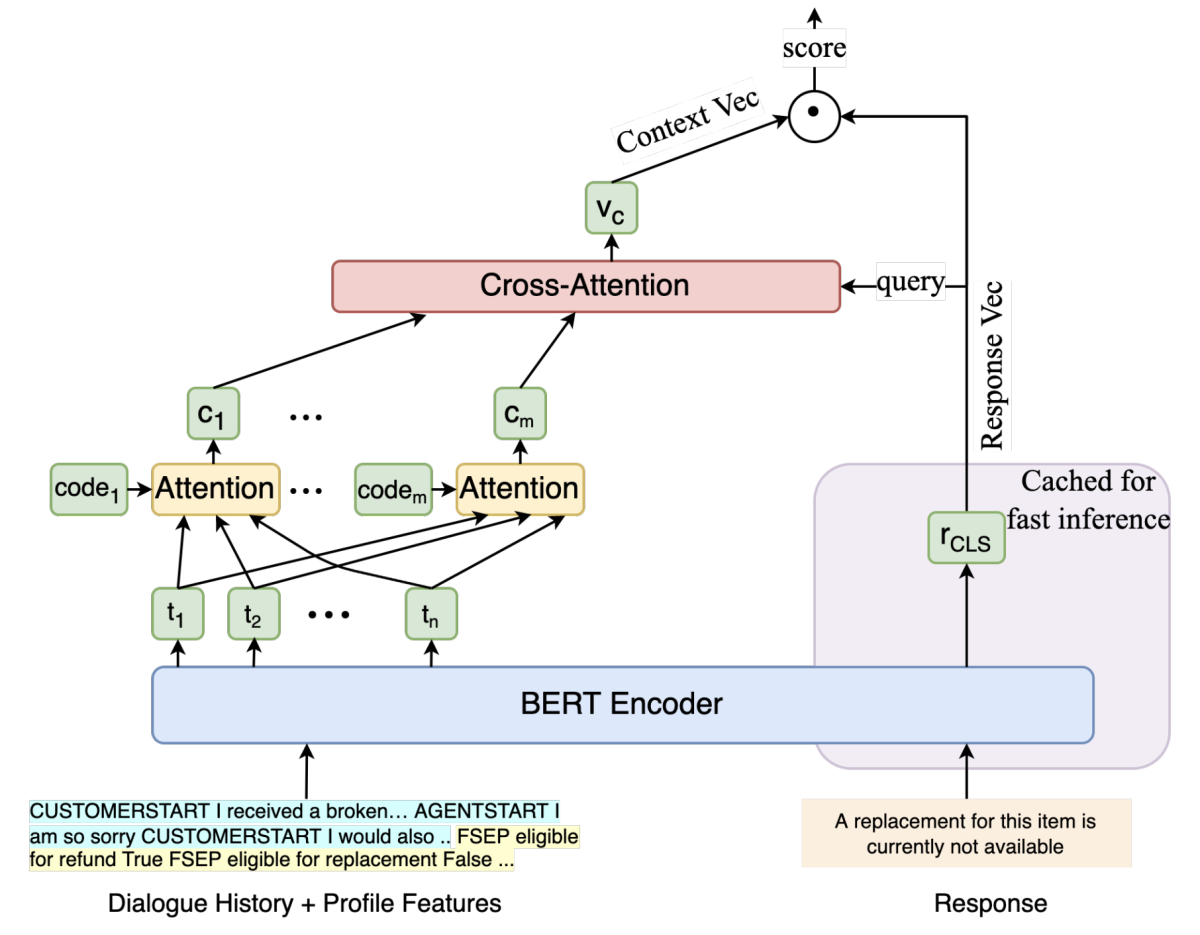
Deploying a retrieval based response model for task oriented dialogues
Lahari Poddar, Gyuri Szarvas, Cheng Wang, Georges Balazs, Pavel Danchenko, Patrick Ernst
Dialogue meaning representation for task-oriented dialogue systems
Xiangkun Hu, Junqi Dai, Hang Yan, Yi Zhang, Qipeng Guo, Xipeng Qiu, Zheng Zhang
Injecting domain knowledge in language models for task-oriented dialogue systems
Denis Emelin, Daniele Bonadiman, Sawsan Alqahtani, Yi Zhang, Saab Mansour
Evaluation
GEMv2: Multilingual NLG benchmarking in a single line of code
Sebastian Gehrmann, Abhik Bhattacharjee, Abinaya Mahendiran, Alex Wang, Alexandros Papangelis, Aman Madaan, Angelina McMillan-Major, Anna Shvets, Ashish Upadhyay, Bernd Bohnet, Bingsheng Yao, Bryan Wilie, Chandra Bhagavatula, Chaobin You, Craig Thomson, Cristina Garbacea, Dakuo Wang, Daniel Deutsch, Deyi Xiong, Di Jin, Dimitra Gkatzia, Dragomir Radev, Elizabeth Clark, Esin Durmus, Faisal Ladhak, Filip Ginter, Genta Indra Winata, Hendrik Strobelt, Jekaterina Novikova, Jenna Kanerva, Jenny Chim, Jiawei Zhou, Jordan Clive, Joshua Maynez, João Sedoc, Juraj Juraska, Kaustubh Dhole, Khyathi Raghavi Chandu, Laura Perez-Beltrachini, Leonardo F. R. Ribeiro, Lewis Tunstall, Li Zhang, Mahima Pushkarna, Mathias Creutz, Michael White, Mihir Sanjay Kale, Moussa Kamal Eddine, Nico Daheim, Nishant Subramani, Ondrej Dusek, Paul Pu Liang, Pawan Sasanka Ammanamanch, Qi Zhu, Ratish Puduppully, Reno Kriz, Rifat Shahriyar, Saad Mahamood, Salomey Osei, Samuel Cahyawijaya, Sanja Štajner, Sebastien Montella, Shailza Jolly, Simon Mille, Tianhao Shen, Tosin Adewumi, Vikas Raunak, Vipul Raheja, Vitaly Nikolaev, Vivian Tsai, Yacine Jernite, Ying Xu, Yisi Sang, Yixin Liu, Yufang Hou
Fact verification
Fact checking machine generated text with dependency trees
Alex Estes, Nikhita Vedula, Marcus D. Collins, Matthew Cecil, Oleg Rokhlenko

Fairness
MT-GenEval: A counterfactual and contextual dataset for evaluating gender accuracy in machine translation
Anna Currey, Maria Nadejde, Raghavendra Pappagari, Mia Mayer, Stanislas Lauly, Xing Niu, Benjamin Hsu, Georgiana Dinu
Humor
Context-situated pun generation
Jiao Sun, Anjali Narayan-Chen, Shereen Oraby, Shuyang Gao, Tagyoung Chung, Jing Huang, Yang Liu, Nanyun Peng
ExPUNations: Augmenting puns with keywords and explanations
Jiao Sun, Anjali Narayan-Chen, Shereen Oraby, Alessandra Cervone, Tagyoung Chung, Jing Huang, Yang Liu, Nanyun Peng
Information extraction
A hybrid approach to cross-lingual product review summarization
Saleh Soltan, Victor Soto, Ke Tran, Wael Hamza
Ask-and-Verify: Span candidate generation and verification for attribute value extraction
Yifan Ding, Yan Liang, Nasser Zalmout, Xian Li, Christan Grant, Tim Weninger
DORE: Document ordered relation extraction based on generative framework
Qipeng Guo, Yuqing Yang, Hang Yan, Xipeng Qiu, Zheng Zhang
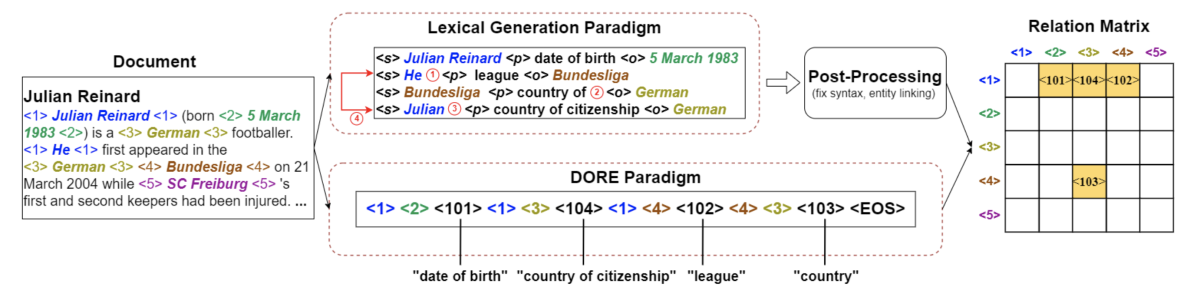
Learning to revise references for faithful summarization
Griffin Adams, Han-Chin Shing, Qing Sun, Christopher Winestock, Kathleen McKeown, Noémie Elhadad
Prototype-representations for training data filtering in weakly-supervised information extraction
Nasser Zalmout, Xian Li
Information retrieval
Accelerating learned sparse indexes via term impact decomposition
Joel Mackenzie, Antonio Mallia, Alistair Moffat, Matthias Petri
Machine translation impact in e-commerce multilingual search
Bryan Zhang, Amita Misra
Knowledge distillation
Distilling multilingual transformers into CNNs for scalable intent classification
Besnik Fetahu, Akash Veeragouni, Oleg Rokhlenko, Shervin Malmasi
Knowledge distillation transfer sets and their impact on downstream NLU tasks
Charith Peris, Lizhen Tan, Thomas Gueudre, Turan Gojayev, Pan Wei, Gokmen Oz
Machine learning
Calibrating imbalanced classifiers with focal loss: An empirical study
Cheng Wang, Georges Balazs, Gyuri Szarvas, Patrick Ernst, Lahari Poddar, Pavel Danchenko
Model adaptation
Meta-learning the difference: Preparing large language models for efficient adaptation
Zejiang Hou, Julian Salazar, George Polovets
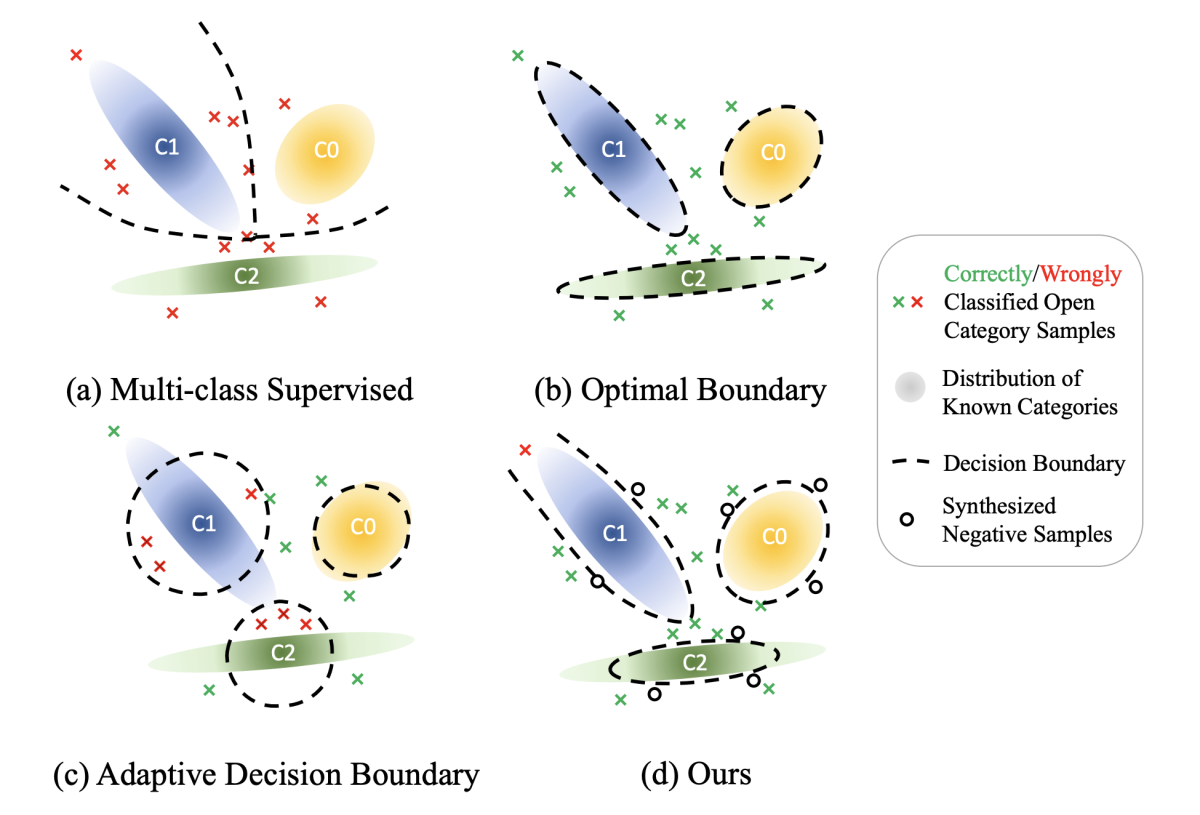
Open world classification with adaptive negative samples
Ke Bai, Guoyin Wang, Jiwei Li, Sunghyun Park, Sungjin Lee, Puyang Xu, Ricardo Henao, Lawrence Carin
Multimodal interaction
Multimodal context carryover
Prashan Wanigasekara, Nalin Gupta, Fan Yang, Emre Barut, Zeynab Raeesy, Kechen Qin, Stephen Rawls, Xinyue Liu, Chengwei Su, Spurthi Sandiri
Natural-language processing
McPhraSy: Multi context phrase similarity and clustering
Amir DN Cohen, Hila Gonen, Ori Shapira, Ran Levy, Yoav Goldberg
Unsupervised syntactically controlled paraphrase generation with abstract meaning representations
Kuan-Hao Huang, Varun Iyer, Anoop Kumar, Sriram Venkatapathy, Kai-Wei Chang, Aram Galstyan
Natural-language understanding
Improving large-scale conversational assistants using model interpretation based training sample selection
Stefan Schroedl, Manoj Kumar, Kiana Hajebi, Morteza Ziyadi, Sriram Venkatapathy, Anil Ramakrishna, Rahul Gupta, Pradeep Natarajan
Improving text-to-SQL semantic parsing with fine-grained query understanding
Jun Wang, Patrick Ng, Alexander Hanbo Li, Jiarong Jiang, Zhiguo Wang, Ramesh Nallapati, Bing Xiang, Sudipta Sengupta
Learning geolocations for cold-start and hard-to-resolve addresses via deep metric learning
Govind, Saurabh Sohoney
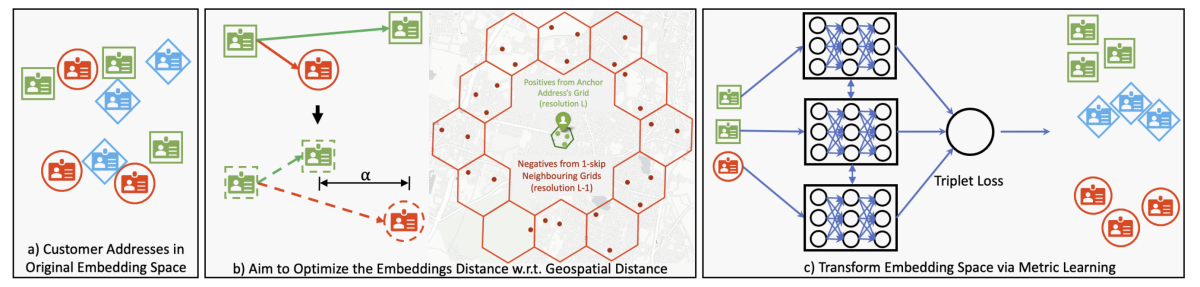
Prompt engineering
DynaMaR: Dynamic prompt with mask token representation
Xiaodi Sun, Sunny Rajagopalan, Priyanka Nigam, Weiyi Lu, Yi Xu, Iman Keivanloo, Belinda Zeng, Trishul Chilimbi
Inducer-tuning: Connecting prefix-tuning and adapter-tuning
Yifan Chen, Devamanyu Hazarika, Mahdi Namazifar, Yang Liu, Di Jin, Dilek Hakkani-Tür
Query rewriting
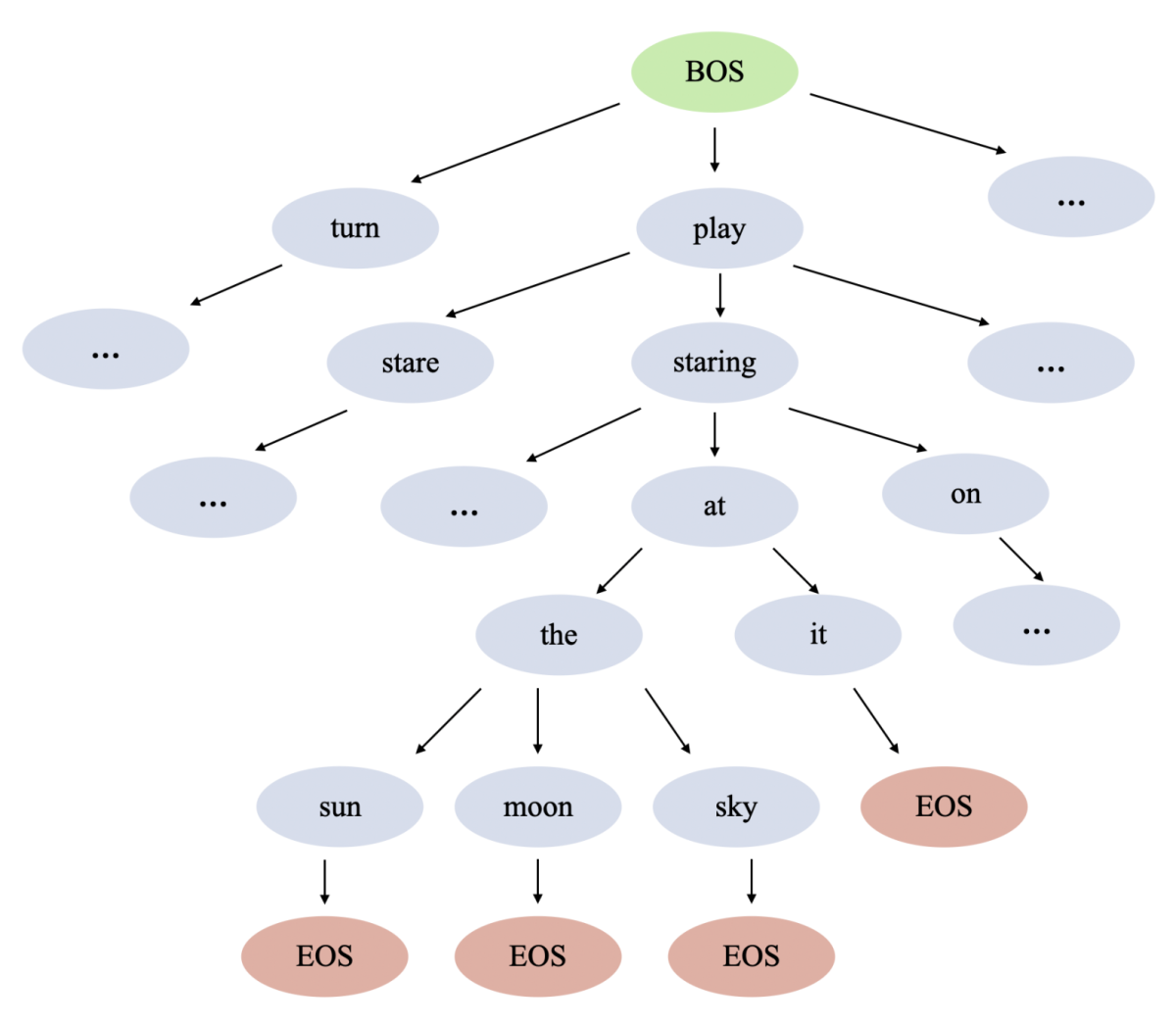
CGF: Constrained generation framework for query rewriting in conversational AI
Jie Hao, Yang Liu, Xing Fan, Saurabh Gupta, Saleh Soltan, Rakesh Chada, Pradeep Natarajan, Edward Guo, Gokhan Tur
CycleKQR: Unsupervised bidirectional keyword question rewriting
Andrea Iovine, Anjie Fang, Besnik Fetahu, Jie Zhao, Oleg Rokhlenko, Shervin Malmasi
PAIGE: Personalized adaptive interactions graph encoder for query rewriting in dialogue systems
Daniel Bis, Saurabh Gupta, Jie Hao, Xing Fan, Edward Guo
PENTATRON: PErsonalized coNText-aware transformer for retrieval-based cOnversational uNderstanding
Niranjan Uma Naresh, Ziyan Jiang, Ankit, Sungjin Lee, Jie Hao, Xing Fan, Edward Guo
Reinforced question rewriting for conversational question answering
Zhiyu Chen, Jie Zhao, Anjie Fang, Besnik Fetahu, Oleg Rokhlenko, Shervin Malmasi
Question answering
Ensemble transformer for efficient and accurate ranking tasks: An application to question answering systems
Yoshitomo Matsubara, Luca Soldaini, Eric Lind, Alessandro Moschitti
FocusQA: Open-domain question answering with a context in focus
Gianni Barlacchi, Ivano Lauriola, Alessandro Moschitti, Marco Del Tredici, Xiaoyu Shen, Thuy Vu, Bill Byrne, Adrià de Gispert
Knowledge transfer from answer ranking to answer generation
Matteo Gabburo, Rik Koncel-Kedziorski, Siddhant Garg, Luca Soldaini, Alessandro Moschitti
Pre-training transformer models with sentence-level objectives for answer sentence selection
Luca Di Liello, Siddhant Garg, Luca Soldaini, Alessandro Moschitti
RLET: A reinforcement learning based approach for explainable QA with entailment trees
Tengxiao Liu, Qipeng Guo, Xiangkun Hu, Yue Zhang, Xipeng Qiu, Zheng Zhang
Robotics
ALFRED-L: Investigating the role of language for action learning in interactive visual environments
Arjun R. Akula, Spandana Gella, Aishwarya Padmakumar, Mahdi Namazifar, Mohit Bansal, Jesse Thomason, Dilek Hakkani-Tür
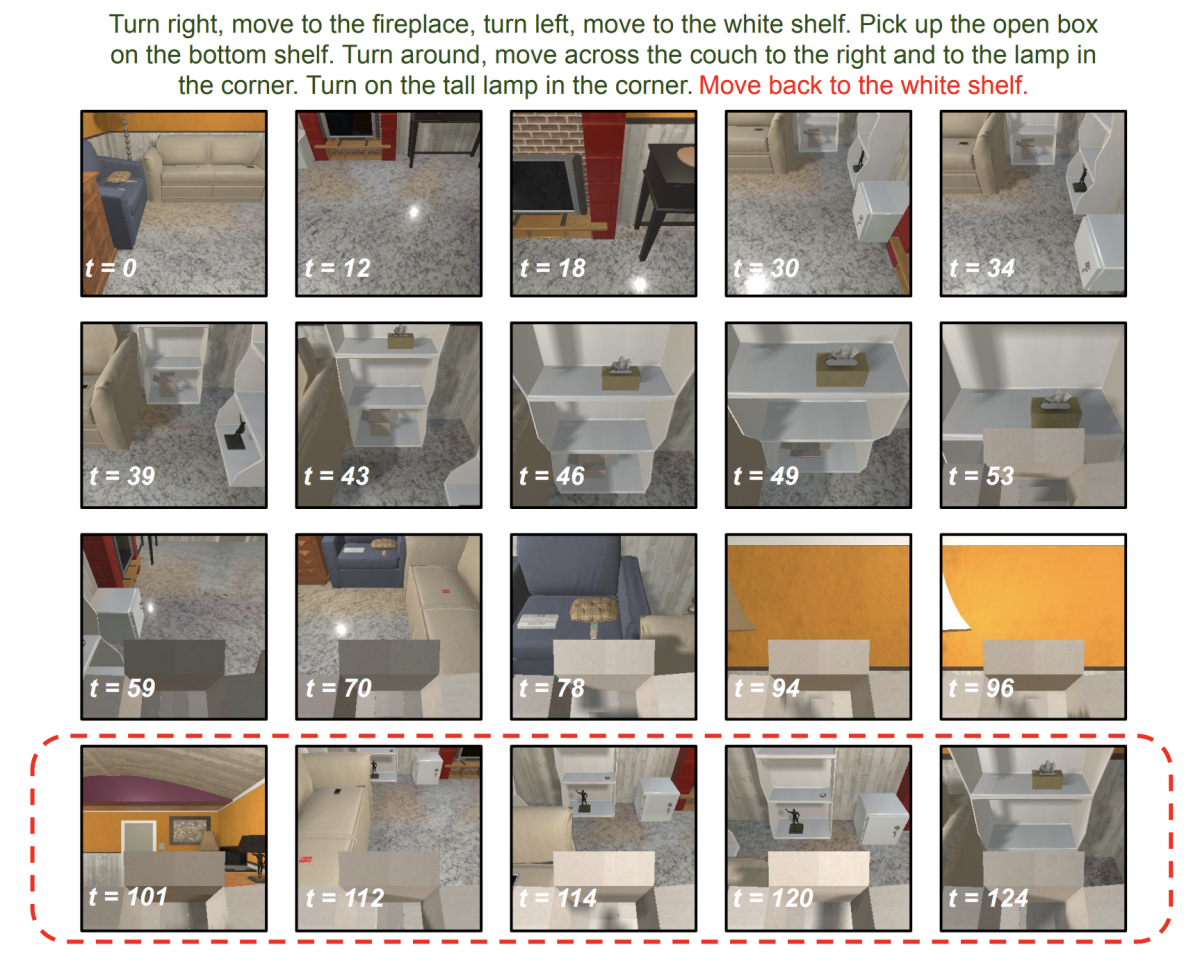