If you’re in a room where a child has just fallen asleep, and someone else walks in, you might start speaking in a whisper, to indicate that you’re trying to keep the room quiet. The other person will probably start whispering, too.
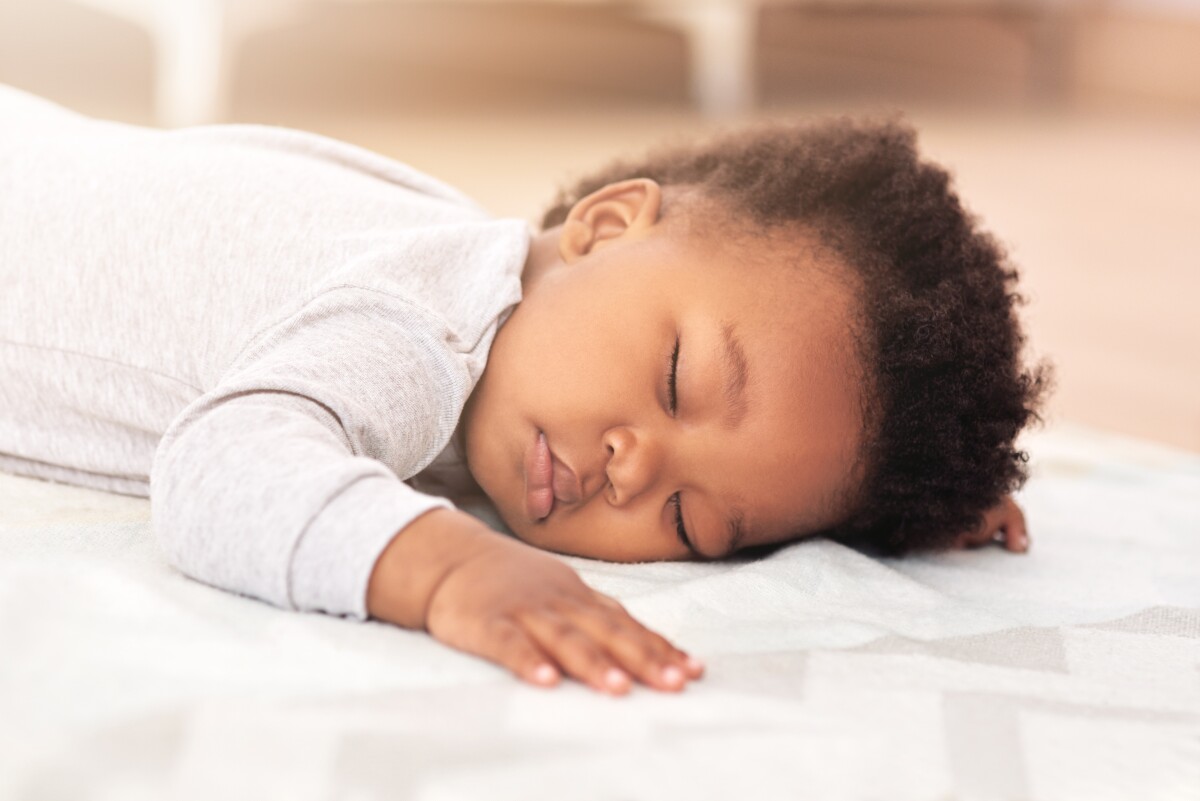
We would like Alexa to react to conversational cues in just such a natural, intuitive way, and toward that end, Amazon last week announced Alexa’s new whisper mode, which will let Alexa-enabled devices respond to whispered speech by whispering back. (The U.S. English version will be available in October.)
At the IEEE Workshop on Spoken Language Technology, in December, my colleagues and I will present a paper that describes the techniques we used to enable whisper mode. The ultimate implementation differs somewhat, but the basic principles are the same.
Whispered speech is predominantly unvoiced, meaning that it doesn’t involve the vibration of the vocal cords, and it has less energy in lower frequency bands than ordinary speech. Previously, researchers have sought to exploit these facts by training their classifiers, not on raw speech signals, but on “features” extracted from the signals, which are designed to capture information that could help discriminate whispers from normal speech.
In our paper, we compare the performance of two different neural nets on the whisper detection task. One is a relatively simple, feed-forward network known as a multilayer perceptron (MLP), and the second is a more sophisticated long short-term memory (LSTM) network.
The models are trained on two categories of features. One is log filter-bank energies, a fairly direct representation of the speech signal that records the signal energies in different frequency ranges. The other is a set of features specifically engineered to exploit the signal differences between whispered and normal speech.
We found that an LSTM network that doesn’t use handcrafted features performs as well as an MLP that does, indicating that LSTMs are capable of learning which signal attributes are most useful for whisper detection. In the paper, we also report an experiment in which the LSTM received the handcrafted features as well as the log filter-bank energies, and its performance improved still further.
After the paper’s acceptance, however, we found that the more data the LSTM saw, the less of an improvement the handcrafted features provided, until the difference evaporated. So the model we moved into production doesn’t use the handcrafted features at all.
There are several advantages to this approach. One is that other components of Alexa’s speech recognition system rely solely on log filter-bank energies. Using the same inputs for different components makes the system as a whole more compact, which is crucial if it is to be used offline, as we envision it will be.
Another advantage is that the handcrafted features are tailored to the data that we’ve seen so far. One of the features we used in our paper, for instance, is the ratio of the energy in the 6,875- to 8,000-hertz frequency band to that in the 310- to 620-hertz band. But it might be that, as we see more training data from more diverse populations, we find that ratios of energies in different frequency bands work better. A network that can learn features on its own is more scalable and can adapt more readily to new data.
LSTMs are widely used in speech recognition and natural-language understanding because they process inputs in sequential order, and their judgments about any given input are conditioned by what they’ve already seen.
This can pose a problem for whisper detection, however. In our system, before passing to the LSTM, the input utterance is broken into overlapping 25-millisecond segments called “frames”, which the LSTM processes in sequence. Because the LSTM’s output for a given frame reflects its outputs for the preceding frames, its confidence in its classifications tends to increase as the utterance progresses.
In a process called “end-pointing”, however, Alexa recognizes the end of an utterance by the short period of silence that follows end of speech, and that silence is part of the input to the whisper detector. When we apply the detector to live data, we typically see that its confidence increases across most of the duration of an utterance then falls off precipitously in the final 50 or so frames.
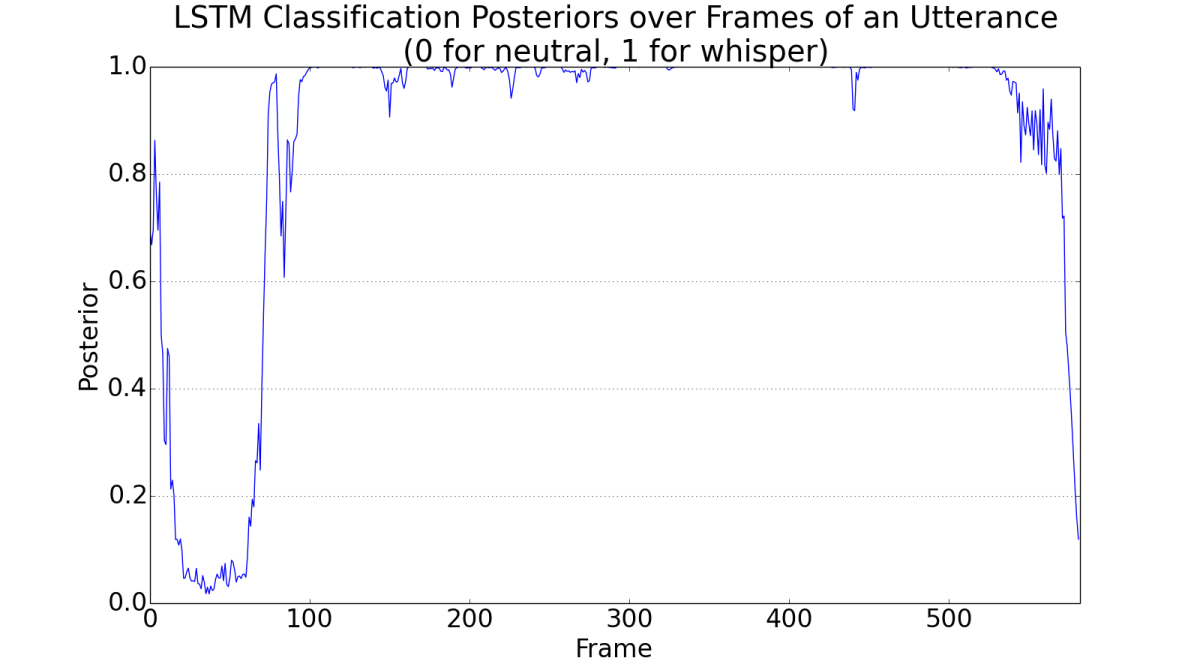
In the experiments reported in the paper, we tried to solve this problem in several different ways. One was to average the LSTM’s outputs for the entire utterance; one was to drop the last 50 frames and average what was left; and the third was to drop the last 50 frames and average only the preceding 100 frames, when the LSTM’s confidence should be at its peak.
Unexpectedly, averaging the entire signal — including the troublesome final 50 frames — yielded the best results. We suspect, however, that that’s because the samples of whispered speech that we used in our experiments were manually segmented, while the samples of normal speech were automatically segmented, using Alexa’s production end-pointer. There could be some consistent difference between manual and automatic segmentation that the system was actually exploiting to distinguish the two types of input, and dropping the final 50 frames made that difference more difficult to detect.
Nevertheless, in production, where both whispered speech and normal speech are segmented by the end-pointer, we’ve found that dropping the final 50 frames of data is crucial to maintaining performance and that averaging across a subset of the preceding frames, rather than the whole remaining signal, yields the best results.
Acknowledgments: Kellen Gillespie, Chengyuan Ma, Thomas Drugman, Jiacheng Gu, Roland Maas, Ariya Rastrow, Björn Hoffmeister