En enero de 2023, Alexa lanzó en España una experiencia de aprendizaje de idiomas para ayudar a los hispanohablantes a aprender inglés para principiantes. Esta experiencia se desarrolló en colaboración con Vaughan, el principal proveedor de aprendizaje de inglés en España, con el objetivo de ofrecer un programa de aprendizaje de inglés inmersivo centrado en la mejora de la pronunciación.
Ahora estamos ampliando esta oferta a México y a la población de habla hispana de Estados Unidos, y en el futuro planeamos añadir más idiomas. Esta experiencia de aprendizaje de idiomas incluye lecciones estructuradas de vocabulario, gramática, expresión y pronunciación, con ejercicios prácticos y pruebas. Para probarla, configura el idioma de tu dispositivo a español y dile a Alexa "Quiero aprender inglés".
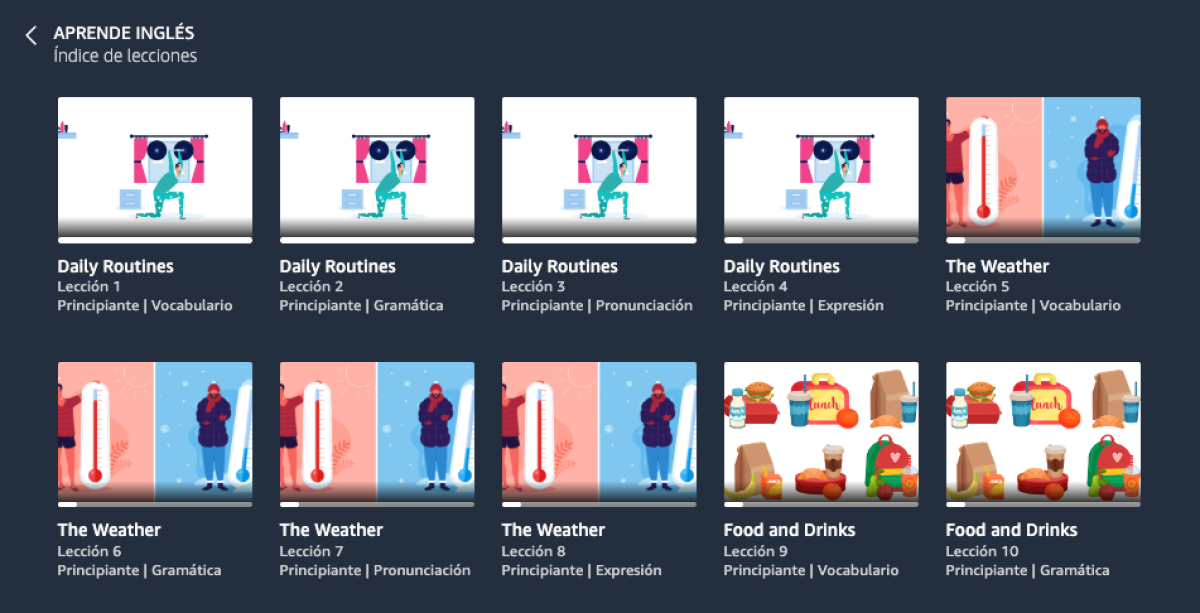
Lo más destacado de esta skill de Alexa es su función de pronunciación, la cual proporciona información precisa cada vez que un cliente pronuncia mal una palabra o frase. En la Conferencia Internacional de Acústica, Habla y Procesamiento de Señales (ICASSP por sus siglas en inglés) de este año, presentamos un artículo en el que describíamos nuestro innovador método de detección de errores de pronunciación.
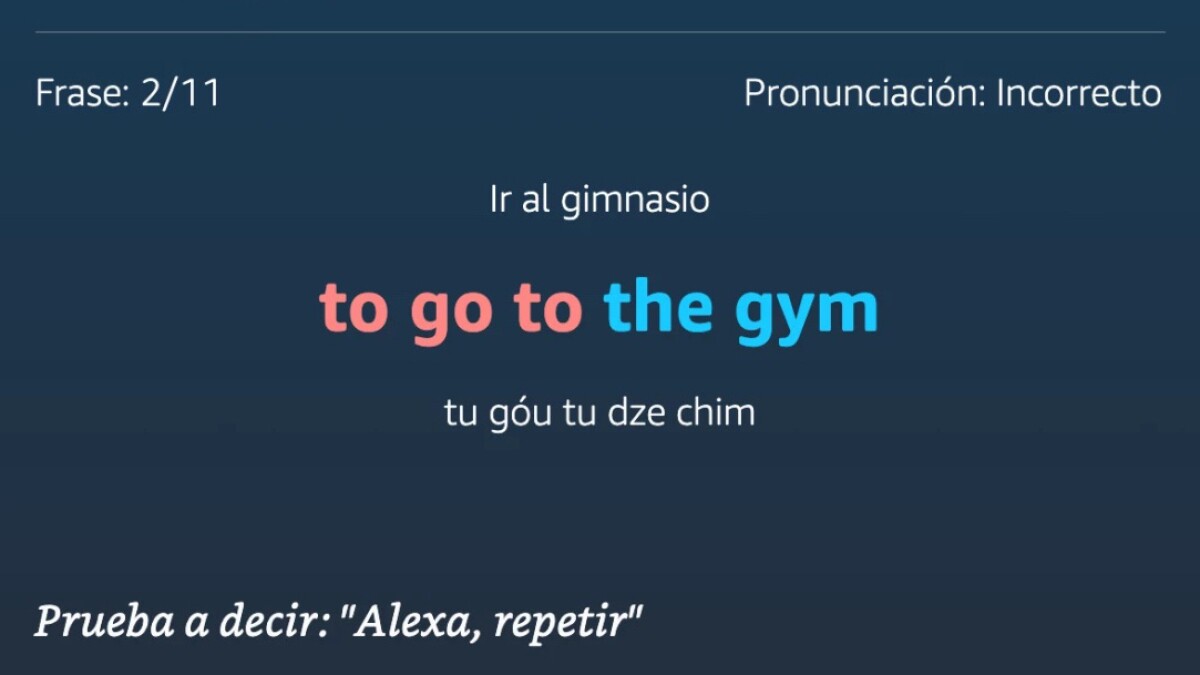
Nuestro método utiliza un novedoso modelo fonético de redes neuronales recurrentes (RNN-T por sus siglas en inglés) que predice los fonemas, las unidades más pequeñas del habla, a partir de la pronunciación del alumno. Por lo tanto, el modelo puede proporcionar una evaluación detallada de la pronunciación a nivel de palabra, sílaba o fonema. Por ejemplo, si un alumno pronuncia incorrectamente la palabra "rabbit" como "rabid", el modelo mostrará la secuencia de cinco fonemas R AE B IH D. Posteriormente, puede detectar los fonemas (IH D) y la sílaba (-bid) mal pronunciados utilizando la alineación de Levenshtein para comparar la secuencia de fonemas con la secuencia de referencia "R AE B AH T".
El artículo destaca dos brechas de conocimiento que no se habían abordado en anteriores modelos de pronunciación. La primera es la capacidad de distinguir fonemas similares en distintos idiomas (por ejemplo, la "r" rodada en español vs. la "r" en inglés). Para ello, diseñamos un léxico de pronunciación multilingüe y creamos un inmenso conjunto de datos fonéticos mixtos para el programa de aprendizaje.
La otra brecha de conocimiento es la capacidad de aprender patrones únicos de pronunciación errónea de los alumnos de idiomas. Para ello, aprovechamos la autorregresividad del modelo RNN-T, es decir, la dependencia de sus resultados de las entradas y salidas anteriores. Este conocimiento del contexto significa que el modelo puede captar patrones frecuentes de pronunciación errónea a partir de los datos del entrenamiento. Nuestro modelo de pronunciación ha obtenido los mejores resultados tanto en precisión de predicción de fonemas, como de detección de errores de pronunciación.
Aumento de datos L2
Uno de los principales retos técnicos a la hora de crear un modelo de reconocimiento fonético para hablantes no nativos (L2) es que los conjuntos de datos para el diagnóstico de errores de pronunciación son muy limitados. En nuestro artículo de Interspeech 2022 "L2-GEN: Un enfoque neuronal de parafraseo de fonemas para el diagnóstico de errores de pronunciación en síntesis del habla L2", planteamos cerrar esta brecha mediante el incremento de datos. En concreto, creamos un parafraseador de fonemas que puede generar fonemas realistas de L2 para hablantes de un lugar específico, por ejemplo, fonemas que representen a un hablante nativo de español hablando en inglés.
Como es habitual en las tareas de corrección de errores gramaticales, utilizamos un modelo de secuencia a secuencia, pero invertimos la dirección de la tarea entrenando al modelo para pronunciar mal las palabras en lugar de corregir los errores de pronunciación. Además, para enriquecer y diversificar aún más las secuencias de fonemas L2 generados, propusimos un componente de decodificación diversificado y consciente de las preferencias que combina una búsqueda en haz diversificada con una pérdida de preferencia que se inclina hacia los errores de pronunciación similares a los humanos.
Para cada tono de entrada o fragmento del habla, el modelo produce varios fonemas posibles como salidas, y las secuencias de fonemas se modelan como un árbol en el que las posibilidades proliferan con cada nuevo tono. Normalmente, las secuencias de fonemas mejor clasificadas se extraen del árbol mediante las búsquedas en haz que persigue solo las ramas del árbol con las probabilidades más altas. En nuestro trabajo, sin embargo, propusimos un método de búsqueda en haz que da prioridad a los fonemas inusuales, o candidatos a fonema que difieren de la mayoría de los demás en la misma profundidad del árbol.
A partir de fuentes establecidas en la documentación sobre aprendizaje de idiomas, también elaboramos listas de errores de pronunciación comunes a nivel de fonema, representados como pares de fonemas, uno del fonema estándar de la lengua y otro de su variante no estándar. Construimos una función de pérdida que, durante el proceso de aprendizaje del modelo, da prioridad a los resultados que utilizan las variantes no estándar de nuestra lista.
En los experimentos observamos mejoras de precisión de hasta el 5% en la detección de errores de pronunciación con respecto a un modelo de referencia entrenado sin datos adicionales.
Equilibrando el falso rechazo y la falsa aceptación
Una consideración clave a la hora de diseñar un modelo de pronunciación para una experiencia de aprendizaje de idiomas es equilibrar la proporción de falsos rechazos y falsas aceptaciones. Un falso rechazo se produce cuando el modelo de pronunciación detecta un error de pronunciación, pero en realidad el alumno estaba en lo cierto o utilizaba una pronunciación coherente pero ligeramente acentuada. Una falsa aceptación se produce cuando un alumno pronuncia mal una palabra y el modelo no lo detecta.
Nuestro sistema tiene dos características de diseño enfocadas a equilibrar estas dos métricas. Para reducir las falsas aceptaciones, primero combinamos nuestros léxicos de pronunciación estándar para inglés y español en un léxico único con múltiples fonemas correspondientes a cada palabra. Después, utilizamos ese léxico para analizar automáticamente muestras de habla no comentadas que se clasifican en tres categorías: español nativo, inglés nativo y español e inglés codificados. El entrenamiento del modelo con este conjunto de datos le permite distinguir diferencias muy sutiles entre fonemas.
Para reducir los falsos rechazos utilizamos un léxico de pronunciación multirreferencial en el que cada palabra se asocia a varias pronunciaciones de referencia. Por ejemplo, la palabra "data" puede pronunciarse como "day-tah" o "dah-tah" y el sistema aceptará ambas variaciones como correctas.
Actualmente seguimos estudiando varios métodos para mejorar nuestra función de evaluación de la pronunciación. Uno de ellos es la creación de un modelo multilingüe que pueda utilizarse para evaluar la pronunciación en muchos idiomas. También estamos ampliando el modelo para diagnosticar más características de pronunciación errónea, como el tono y el acento léxico.