The computational notebook is an interactive, web-based programming interface based on the concept of a lab notebook. Users can describe the computations they’re performing — including diagrams — and embed code in the notebook, and the notebook backend will execute the code, integrating the results into the notebook layout.
Jupyter Notebook is the most popular implementation of computational notebooks, and it has become the tool of choice for data scientists. By September 2018, there were more than 2.5 million public Jupyter notebooks on GitHub, and this number has been growing rapidly.
However, using Jupyter Notebook poses several challenges related to code maintenance and machine learning best practices. We recently surveyed 2,669 machine learning (ML) practitioners, and 33% of them mentioned that notebooks get easily cluttered due to the mix of code, documentation, and visualization. Similarly, 23% found silent bugs hard to detect, and 18% agreed that global variables are inconsistently used. Another 15% found reproduction of notebooks to be hard, and 6% had difficulty detecting and remediating security vulnerabilities within notebooks.
We are excited to share our recent launch of the Amazon CodeGuru extension for JupyterLab and SageMaker Studio. The extension seamlessly integrates with JupyterLab and SageMaker Studio, and with a single button click, it can provide users feedback and suggestions for improving their code quality and security. To learn more about how to install and use this extension, check out this user guide.
Static analysis
Traditional software development environments commonly use static-analysis tools to identify and prevent bugs and enforce coding standards, but Jupyter notebooks currently lack such tools. We on the Amazon CodeGuru team, which has developed a portfolio of code analysis tools for Amazon Web Services customers, saw a great opportunity to adapt our existing tools for notebooks and build solutions that best fit this new problem area.
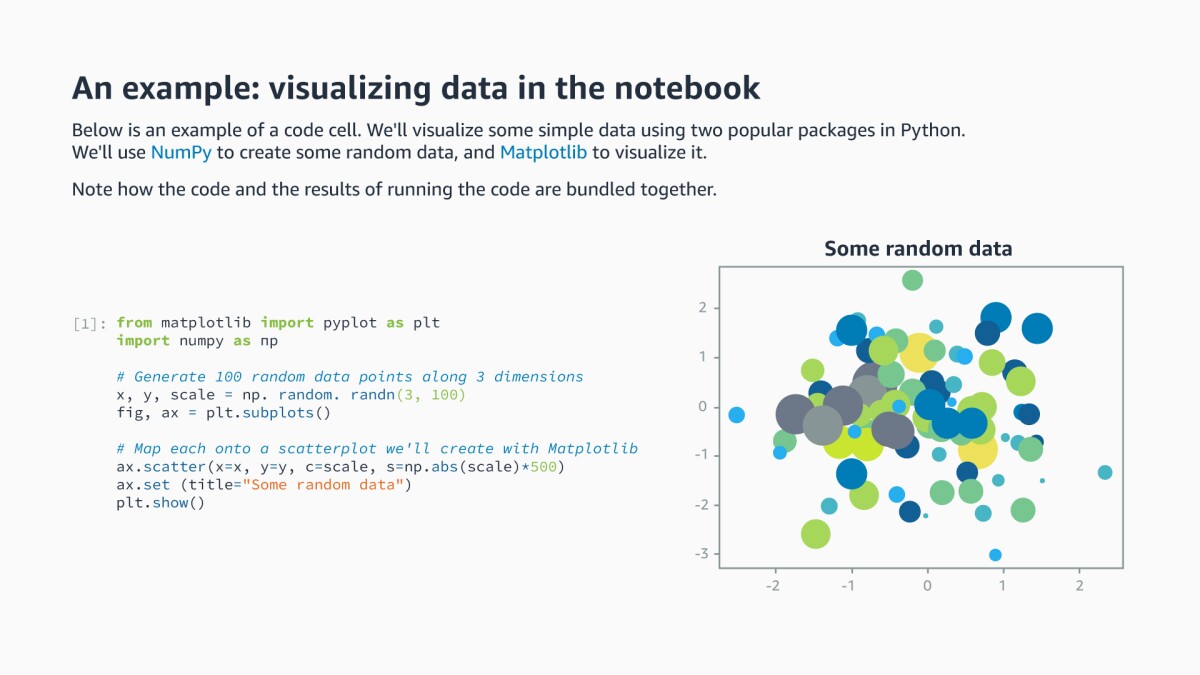
We presented our initial efforts in a paper published at the 25th International Symposium on Formal Methods in March 2023. The paper reports insights from our survey and from interviews with ML practitioners to understand what specific issues need to be addressed in the notebook context. In the following, we give two examples of how our new technologies can help machine learning experts to be more productive.
Execution order
Code is embedded in computational notebooks in code cells, which can be executed in an arbitrary order and edited on the fly; that is, cells can be added, deleted, or changed after other cells have been executed.
While this flexibility is great for exploring data, it raises problems concerning reproducibility, as cells with shared variables can produce different results when running in different orders.
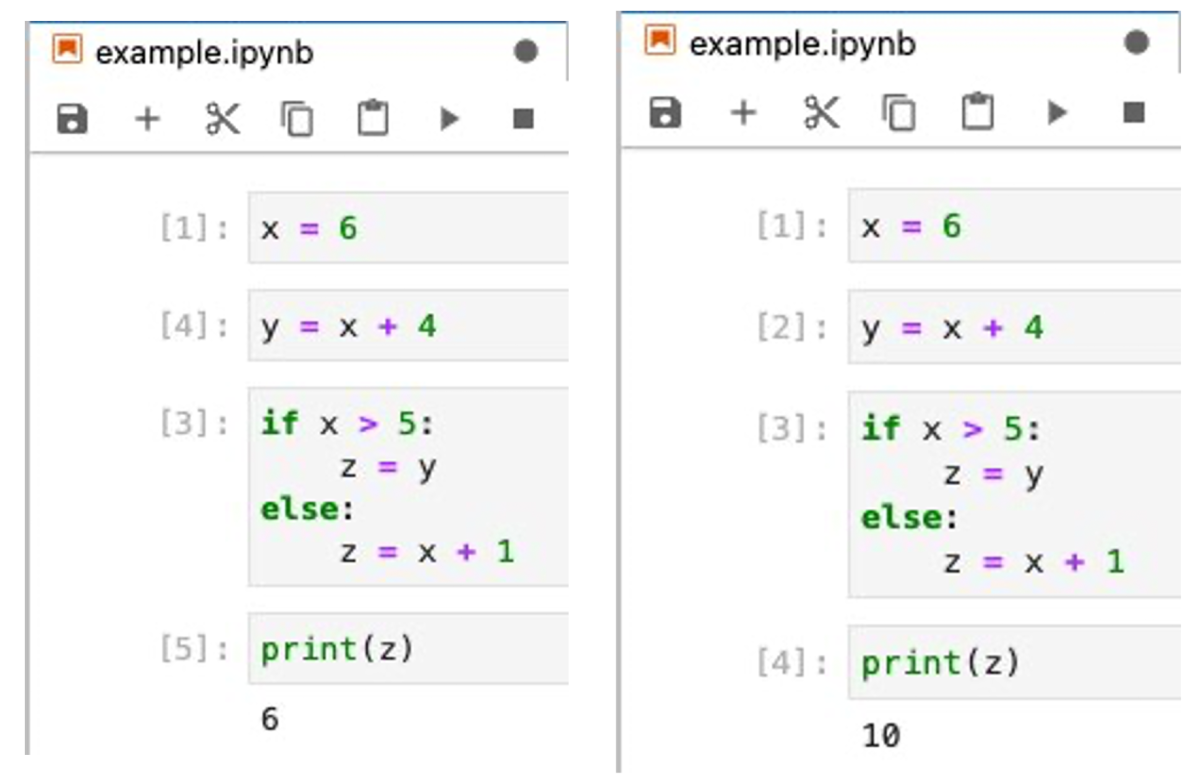
Once a code cell is executed, it is assigned an integer number in the square bracket on its left side. This number is called the execution count, and it indicates the cell’s position in the execution order. In the example above, when code cells are executed in nonlinear order, the variable z ends up with the value 6. However, execution count 2 is missing in the notebook file, which can happen for multiple reasons: perhaps the cell was executed and deleted afterwards, or perhaps one of the cells was executed twice. In any case, it would be hard for a second person to reproduce the same result.
To catch problems resulting from out-of-order execution in Jupyter notebooks, we developed a hybrid approach that combines dynamic information capture and static analysis. Our tool collects dynamic information during the execution of notebooks, then converts notebook files with Python code cells into a novel Python representation that models the execution order as well as the code cells as such. Based on this model, we are able to leverage our static-analysis engine for Python and design new static-analysis rules to catch issues in notebooks.
APIs
Another common problem for notebook users is misuse of machine-learning APIs. Popular machine learning libraries such as PyTorch, TensorFlow, and Keras greatly simplify the development of AI systems. However, due to the complexity of the field, the libraries’ high level of abstraction, and the sometimes obscure conventions governing library functions, library users often misuse these APIs and inject faults into their notebooks without even knowing it.
The code below shows such a misuse. Some layers of a neural network, such as dropout layers, may behave differently during the training and evaluation of the network. PyTorch mandates explicit calls to train() and eval() to denote the start of training and evaluation, respectively. The code example is intended to load a trained model from disk and evaluate it on some test data.
However, it misses the call to eval(), as by default, every model is in the training phase. In this case, some layers will indirectly change the architecture of the network, which would make all prediction unstable; i.e., for the same input, the predictions would be different at different times.
# noncompliant case model.load_state_dict(torch.load("model.pth")) predicted = model.evaluate_on(test_data) # compliant case model.load_state_dict(torch.load("model.pth")) model.eval() predicted = model.evaluate_on(test_data)
Instabilities caused by this bug can have a serious impact. Even when the bug is found (currently, through manual code review) and fixed, the model needs to be retrained. Depending on how large the model is and how late in the development process the bug is found, this could mean a waste of thousands of hours.
The best case would be to detect the bug directly after the developer writes the code. Static analysis can help with this. In our paper, we implemented a set of static-analysis rules that automatically analyze machine learning code in Jupyter notebooks and could detect such bugs with high precision.
In experiments involving a large set of notebook files, our rules found an average of one bug per seven notebooks. This result motivates us to dive deep into bug detection in Jupyter notebooks.
Our survey identified the following issues that notebook users care about:
- Reproducibility: People often find it difficult to reproduce results when moving notebooks between different environments. Notebook code cells are often executed in nonlinear order, which may be not reproducible. About 14% of the survey participants collaborate on notebooks with others only when models need to be pushed into production; reproducibility is even more crucial for production notebooks.
- Correctness: People introduce silent correctness bugs without knowing it when using machine learning libraries. Silent bugs affect model outputs but do not cause program crashes, which makes them extremely hard to find. In our survey, 23% of participants confirmed this.
- Readability: During data exploration, notebooks can easily get messy and hard to read. This hampers maintainability as well as collaboration. In our survey, 32% of participants mentioned that readability is one of the biggest difficulties in using notebooks.
- Performance: It is time- and memory-consuming to train big models. People want help to make both training and the runtime execution of their code more efficient.
- Security: In our survey, 34% of participants said that security awareness among ML practitioners is low and that there is a consequent need for security scanning. Because notebooks often rely on external code and data, they can be vulnerable to code injection and data-poisoning attacks (manipulating machine learning models).
These findings pointed us toward the kinds of issues that our new analysis rules should address. During the rule sourcing and specification phase, we asked ML experts for feedback on the usefulness of the rules as well as examples of compliant and noncompliant cases to illustrate the rules. After developing the rules, we invited a group of ML experts to evaluate our tools on real-world notebooks. We used their feedback to improve the accuracy of the rules.
The newly launched Amazon CodeGuru extension for JupyterLab and SageMaker Studio enables the enforcement of code quality and security in computational notebooks to “shift left”, or move earlier in the development process. Users can now detect security vulnerabilities — such as injection flaws, data leaks, weak cryptography, and missing encryption — within notebook cells, along with other common issues that affect the readability, reproducibility, and correctness of the computations performed by notebooks.
Acknowledgements: Martin Schäf, Omer Tripp