Machine learning models are usually tested on held-out data from their training sets. But when data distributions in the real world diverge from those in the test set, such tests can overstate model performance. And models can still fail in surprising ways — for instance, systematically mishandling inputs that don’t seem any more challenging than others that the model handles smoothly.
At the NeurIPS 2021 Workshop on Explainable AI Approaches for Debugging and Diagnosis (XAI4Debugging), my colleagues and I will present Defuse, a method that automatically discovers model errors on particular types of input and provides a way to correct them.
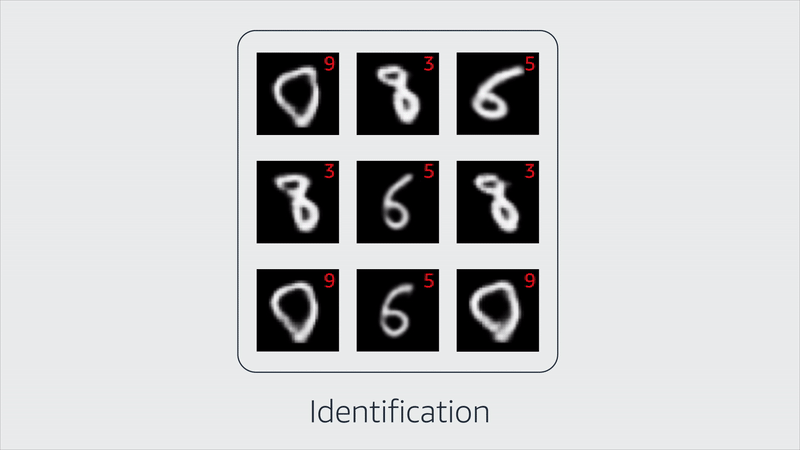
Given a trained image classification model (a classifier), Defuse generates realistic-looking new images that are variations on test-set inputs that the classifier mishandles. Then Defuse sorts misclassified images into high-level “model bugs” — groups of similar images that consistently cause errors. Users can then identify scenarios under which their models would fail and train more-robust models.
During retraining, we mix the artificially generated error-causing data with the model’s original training data, in a ratio controlled by a parameter, λ. In experiments, we show that for each of three sample datasets, there is a value of λ that enables consistently high performance on the input classes identified by Defuse while largely preserving performance on the original data.
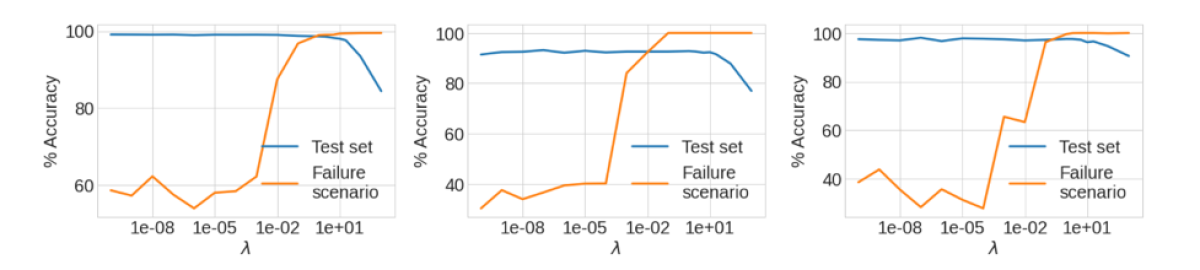
To spur further research on this problem, we have publicly released the code for Defuse on GitHub.
Data augmentation
To generate our synthetic images, we train a variational autoencoder (VAE) on the classifier’s training data. A VAE is a model trained to output the same data that it takes as input, but in-between, it produces a vector representation that captures salient properties of the input. That vector representation defines a latent space in which proximity indicates similarity of data.
Once the VAE is trained, we use its latent space to generate new image data. In the image below, we show how the VAE can interpolate between two test images — which define points in the latent space — to generate new related images.
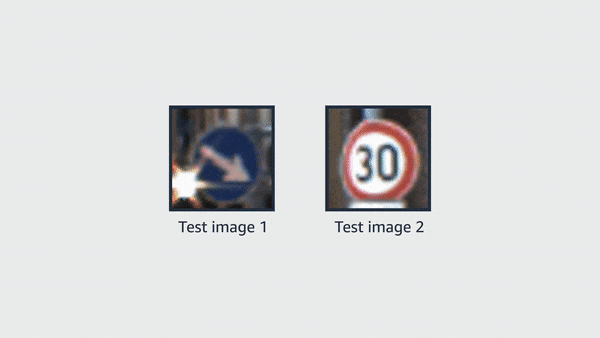
The VAE’s latent representations are the basis of Defuse’s three main steps: identification, distillation, and correction.
Identification: First, Defuse encodes all the images from the training data using the trained VAE. Perturbing a latent code with small amounts of noise should cause the decoded instances to have small but semantically meaningful differences from the original instance. The perturbations are assigned the same label as the original instance, and Defuse saves instances that the classifier misclassifies. In the figure above, the red number in the upper right-hand corner of each input image is the classifier’s prediction.
Distillation: Next, a clustering model groups together the latent codes of the images from the previous step to diagnose misclassification regions. In the example above, Defuse groups together generated images of the digit 8 that are incorrectly classified as 3. Defuse uses a Gaussian mixture model with a Dirichlet process prior because the number of clusters is unknown ahead of time.
Below is a low-dimensional (t-distributed stochastic neighbor embedding, or t-SNE) visualization of latent codes obtained from one of the three datasets we used in our experiments. The blue circles are the latent codes of images in the training set; the black dots are the latent codes of generated images that were identified as classifier mistakes. The red dots are the three decoded latent codes, where the red number in the upper left-hand corner is the classifier label.
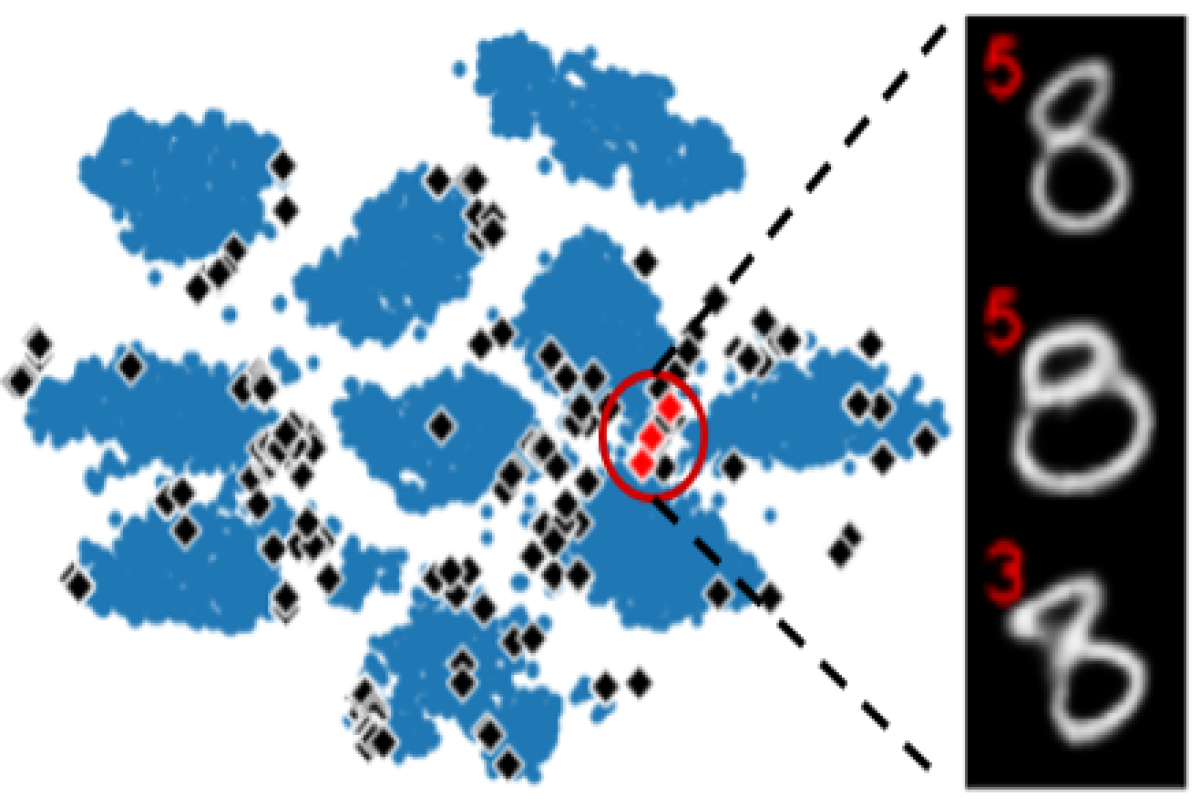
Correction: The clusters created in the distillation step are then annotated by labelers. Defuse runs the correction step using both the annotator-labeled data and the original training data, mixed according the parameter λ.
Experiments
We conducted experiments on three public benchmark datasets, assessing accuracy on both the misclassification region test data and the original test set after performing correction. We compared Defuse to finetuning only on the images from the identification step that are labeled as classifier mistakes by annotators. We expect this baseline to be reasonable because related works that focus on robustness to classic adversarial attacks demonstrate the effectiveness of tuning directly on the adversarial examples. Overall, these results indicate that the correction step in Defuse is highly effective at correcting the errors discovered during identification and distillation.
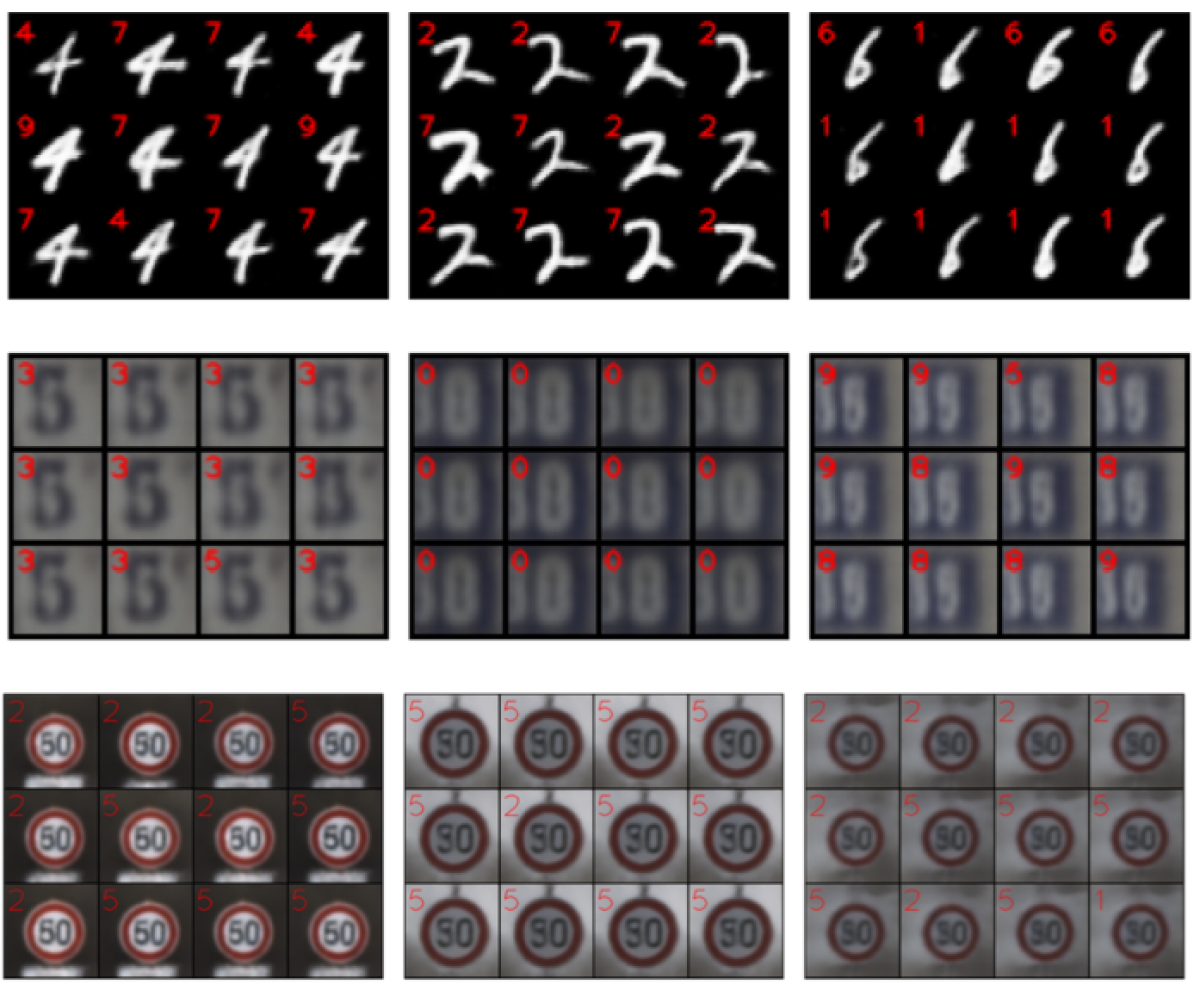
Novelty of the misclassified instances generated with Defuse: We expect Defuse to find novel model misclassifications beyond those revealed by the available data. To test this hypothesis, we compared the errors proposed by Defuse (the misclassification region data) and the misclassified training data.
We chose 10 images from the misclassification regions and found their nearest neighbors in the misclassified training data. In the figure below, we can see that the data in the misclassification regions reveal different types of errors than are found in the training set.

To learn more about Defuse check out the paper and the repository.
Acknowledgements: Krishnaram Kenthapadi