The Conference on Neural Information Processing Systems (NeurIPS) remains the highest-profile conference in AI, and as such, it draws paper submissions from across Amazon’s business lines. Some of those papers concern specific application areas, like computer vision and recommender systems, but many of them address more general problems, such as continual learning, federated learning, and privacy. And some of them investigate ways to improve popular machine learning methods, such as contrastive learning or variational autoencoders.
Below is a quick guide to the main-conference papers from Amazon researchers at this year’s NeurIPS.
Algorithmic fairness
Are two heads the same as one? Identifying disparate treatment in fair neural networks
Michael Lohaus, Matthaus Kleindessner, Krishnaram Kenthapadi, Francesco Locatello, Chris Russell
Computer vision
An in-depth study of stochastic backpropagation
Jun Fang, Mingze Xu, Hao Chen, Bing Shuai, Zhuowen Tu, Joseph Tighe
Self supervised amodal video object segmentation
Jian Yao, Yuxin Hong, Chiyu Wang, Tianjun Xiao, Tong He, Francesco Locatello, David Wipf, Yanwei Fu, Zheng Zhang
Self-supervised pretraining for large-scale point clouds
Zaiwei Zhang, Min Bai, Erran Li
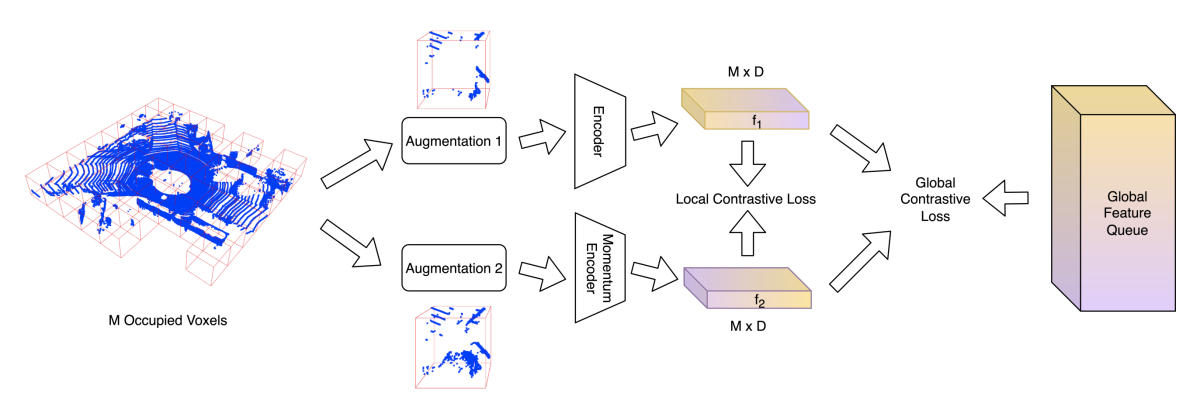
Semi-supervised vision transformers at scale
Zhaowei Cai, Avinash Ravichandran, Paolo Favaro, Manchen Wang, Davide Modolo, Rahul Bhotika, Zhuowen Tu, Stefano Soatto
Continual learning
Measuring and reducing model update regression in structured prediction for NLP
Deng Cai, Elman Mansimov, Yi-An Lai, Yixuan Su, Lei Shu, Yi Zhang
Memory efficient continual learning with transformers
Beyza Ermis, Giovanni Zappella, Martin Wistuba, Cédric Archambeau
Distribution shifts
Assaying out-of-distribution generalization in transfer learning
Florian Wenzel, Andrea Dittadi, Peter Gehler, Carl-Johann Simon-Gabriel, Max Horn, Dominik Zietlow, David Kernert, Chris Russell, Thomas Brox, Bernt Schiele, Bernhard Schölkopf, Francesco Locatello
Neural attentive circuits
Martin Weiss, Nasim Rahaman, Francesco Locatello, Chris Pal, Yoshua Bengio, Nicolas Ballas, Erran Li
Earth system forecasting
Earthformer: Exploring space-time transformers for earth system forecasting
Zhihan Gao, Xingjian Shi, Hao Wang, Yi Zhu, Yuyang (Bernie) Wang, Mu Li, Dit-Yan Yeung

Federated learning
Self-aware personalized federated learning
Huili Chen, Jie Ding, Eric Tramel, Shuang Wu, Anit Kumar Sahu, Salman Avestimehr, Tao Zhang
Machine learning methods
Embrace the gap: VAEs perform independent mechanism analysis
Patrik Reizinger, Luigi Gresele, Jack Brady, Julius von Kuegelgen, Dominik Zietlow, Bernhard Schölkopf, Georg Martius, Wieland Brendel, Michel Besserve
Learning manifold dimensions with conditional variational autoencoders
Yijia Zheng, Tong He, Yixuan Qiu, David Wipf
On the detrimental effect of invariances in the likelihood for variational inference
Richard Kurle, Ralf Herbrich, Tim Januschowski, Yuyang (Bernie) Wang, Jan Gasthaus
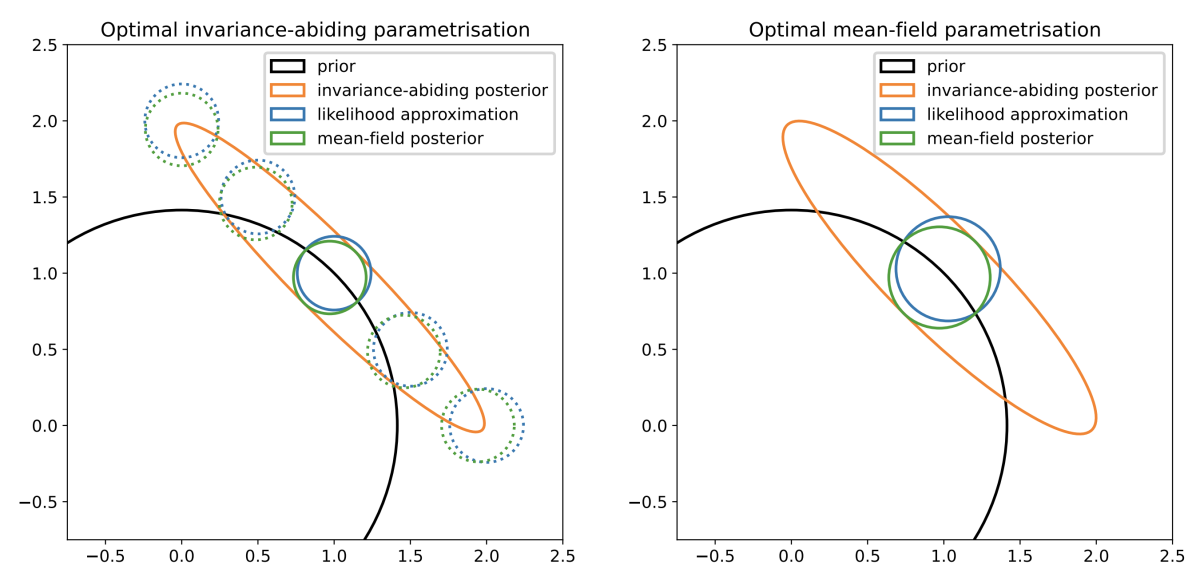
Why do we need large batch sizes in contrastive learning? A gradient-bias perspective
Changyou Chen, Jianyi Zhang, Yi Xu, Liqun Chen, Jiali Duan, Yiran Chen, Son Tran, Belinda Zeng, Trishul Chilimbi
Privacy
Private synthetic data for multitask learning and marginal queries
Giuseppe Vietri, Cédric Archambeau, Sergul Aydore, William Brown, Michael Kearns, Aaron Roth, Ankit Siva, Shuai Tang, Steven Wu
Recommender systems
Toward understanding privileged features distillation in learning-to-rank
Shuo Yang, Sujay Sanghavi, Holakou Rahmanian, Jan Bakus, S. V. N. Vishwanathan
Uplifting bandits
Yu-Guan Hsieh, Shiva Kasiviswanathan, Branislav Kveton
Reinforcement learning
Adaptive interest for emphatic reinforcement learning
Martin Klissarov, Rasool Fakoor, Jonas Mueller, Kavosh Asadi, Taesup Kim, Alex Smola
Faster deep reinforcement learning with slower online network
Kavosh Asadi, Rasool Fakoor, Omer Gottesman, Taesup Kim, Michael L. Littman, Alex Smola
Tabular data
Learning enhanced representations for tabular data via neighborhood propagation
Kounianhua Du, Weinan Zhang, Ruiwen Zhou, Yangkun Wang, Xilong Zhao, Jiarui Jin, Quan Gan, Zheng Zhang, David Paul Wipf
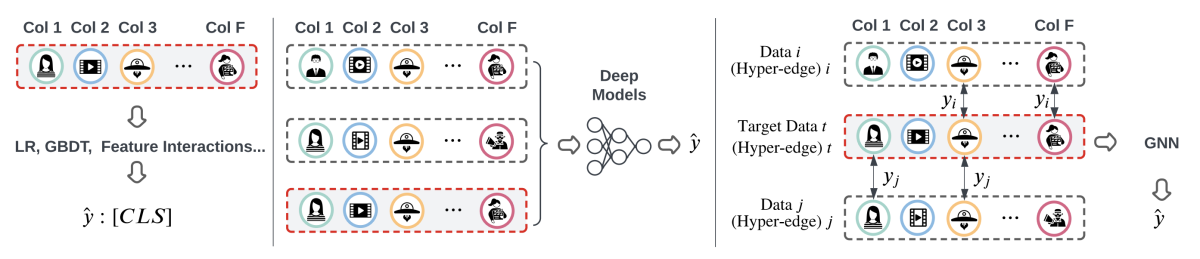