Customer-obsessed science
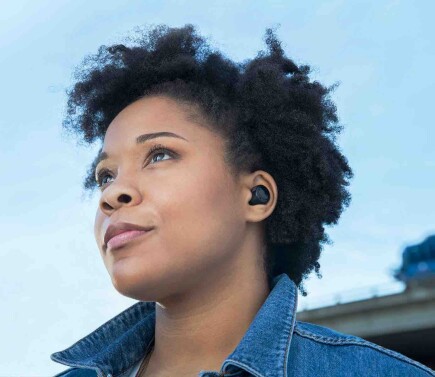
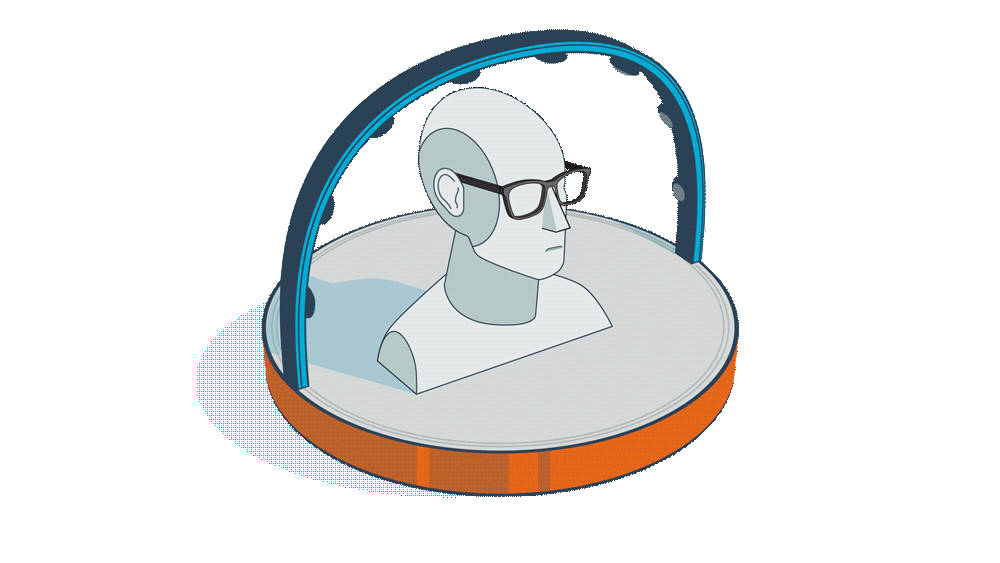
Research areas
-
April 11, 2025Novel three-pronged approach combines claim-level evaluations, chain-of-thought reasoning, and classification of hallucination error types.
-
-
Featured news
-
2025The effectiveness of automatic evaluation of generative models is typically measured by comparing the labels generated via automation with human labels using correlation metrics. However, metrics like Krippendorff’s α and Randolph’s κ were originally designed to measure the reliability of human labeling, thus make assumptions about typical human labeling behavior, and these assumptions may not be applicable
-
2025Retrieval-augmented generation (RAG) enhances the question answering (QA) abilities of large language models (LLMs) by integrating external knowledge. However, adapting general-purpose RAG systems to specialized fields such as science and medicine poses unique challenges due to distribution shifts and limited access to domain-specific data. To tackle this, we propose SimRAG, a self-training approach that
-
2025Foundation models, such as large language models, have achieved remarkable success in natural language processing and are evolving into models capable of handling multiple modalities. Listening ability, in particular, is crucial for many applications, leading to research on building speech foundation models. However, the high computational cost of these large models presents a significant challenge for
-
QID: Efficient query-informed ViTs in data-scarce regimes for OCR-free visual document understanding2025In Visual Document Understanding (VDU) tasks, fine-tuning a pre-trained Vision-Language Model (VLM) with new datasets often falls short in optimizing the vision en-coder to identify query-specific regions in text-rich document images. Existing methods that directly inject queries into model layers by modifying the network architecture often struggle to adapt to new datasets with limited annotations. To
-
2025Recent advances in Multi-Modal Large Language Models (M-LLMs) show promising results in video reasoning. Popular Multi-Modal Large Language Model (M-LLM) frameworks usually apply naive uniform sampling to reduce the number of video frames that are fed into an M-LLM, particularly for long context videos. However, it could lose crucial context in certain periods of a video, so that the downstream M-LLM may
Academia
View allWhether you're a faculty member or student, there are number of ways you can engage with Amazon.
View all