Editor's note: This article, which is a selection of quotes from "Advice to a Young Scientist" coupled with commentary from Farnam Street staff, originally ran in May 2021 on the Farnam Street blog. It is reprinted here in its entirety with the gracious permission of Farnam Street.
The Nobel Prize-winning biologist Peter Medawar (1915–1987) is best known for work that made the first organ transplants and skin grafts possible. Medawar was also a lively, witty writer who penned numerous books on science and philosophy.
In 1979, he published Advice to a Young Scientist, a book brimming with both practical advice and philosophical guidance for anyone “engaged in exploratory activities.” Here, we summarize some of Medawar’s key insights from the book.
Application, diligence, a sense of purpose
“There is no certain way of telling in advance if the daydreams of a life dedicated to the pursuit of truth will carry a novice through the frustration of seeing experiments fail and of making the dismaying discovery that some of one’s favourite ideas are groundless.”
If you want to make progress in any area, you need to be willing to give up your best ideas from time to time.
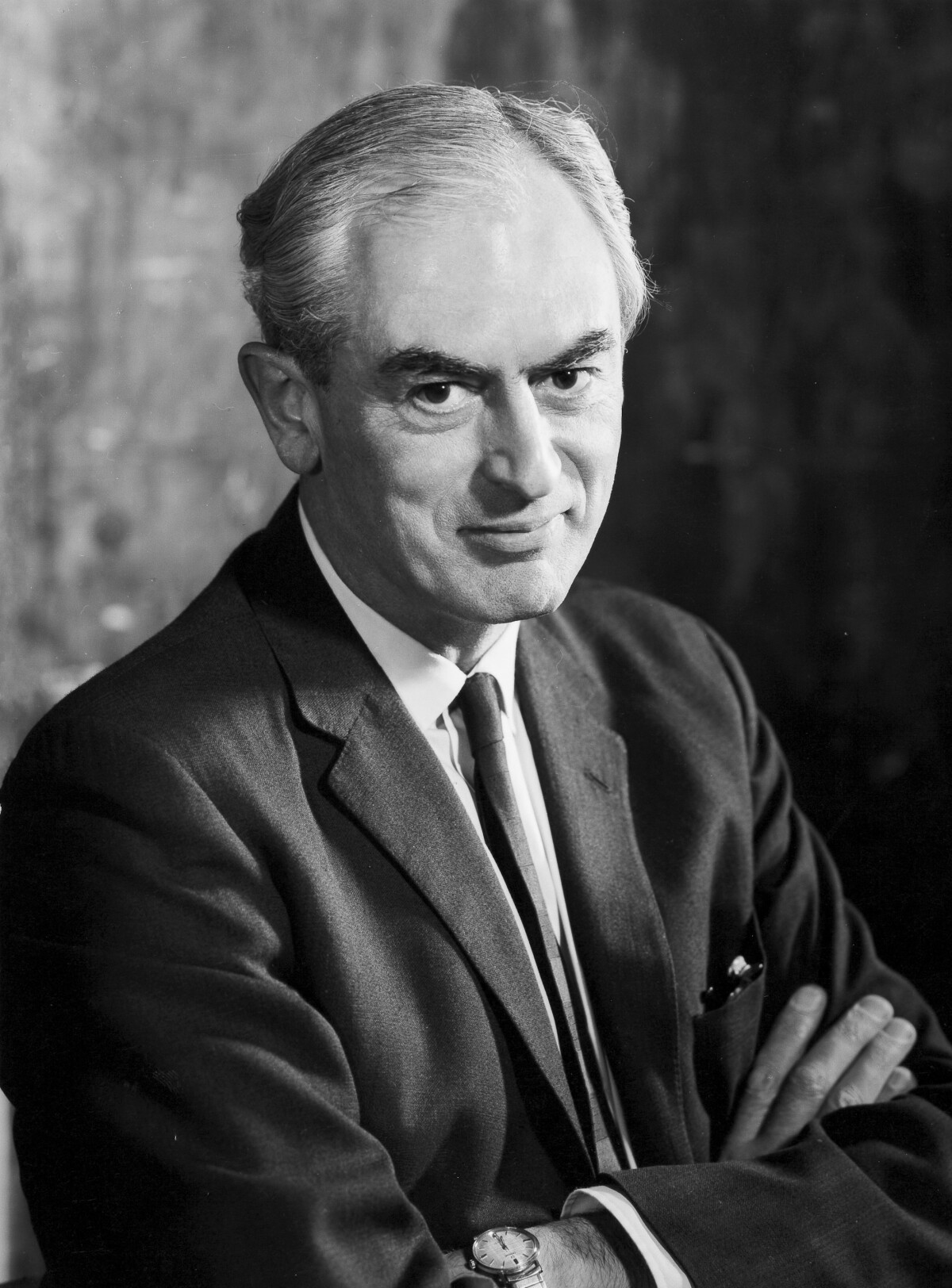
Science proceeds because researchers do all they can to disprove their hypotheses rather than prove them right. Medawar notes that he twice spent two whole years trying to corroborate groundless hypotheses. The key to being a good scientist is the capacity to take no for an answer— when necessary. Additionally:
“…one does not need to be terrifically brainy to be a good scientist…there is nothing in experimental science that calls for great feats of ratiocination or a preternatural gift for deductive reasoning. Common sense one cannot do without, and one would be the better for owning some of those old-fashioned virtues which have fallen into disrepute. I mean application, diligence, a sense of purpose, the power to concentrate, to persevere and not be cast down by adversity—by finding out after long and weary inquiry, for example, that a dearly loved hypothesis is in large measure mistaken.”
The truth is, any measure of risk-taking comes with the possibility of failure. Learning from failure to continue exploring the unknown is a broadly useful mindset.
How to make important discoveries
“It can be said with marked confidence that any scientist of any age who wants to make important discoveries must study important problems. Dull or piffling problems yield dull or piffling answers.”
A common piece of advice for people early on in their careers is to pursue what they find most interesting. Medawar disagrees, explaining that “almost any problem is interesting if it is studied in sufficient depth.” He advises scientists to look for important problems, meaning ones with answers that matter to humankind.
When choosing an area of research, Medawar cautions against mistaking a fashion (“some new histochemical procedure or technical gimmick”) for a movement (“such as molecular genetics or cellular immunology”). Movements lead somewhere; fashions generally don’t.
Getting started
Whenever we begin some new endeavor, it can be tempting to think we need to know everything there is to know about it before we even begin. Often, this becomes a form of procrastination. Only once we try something and our plans make contact with reality can we know what we need to know. Medawar believes it’s unnecessary for scientists to spend an enormous amount of time learning techniques and supporting disciplines before beginning research:
“As there is no knowing in advance where a research enterprise may lead and what kind of skills it will require as it unfolds, this process of ‘equipping oneself’ has no predeterminable limits and is bad psychological policy….The great incentive to learning a new skill or supporting discipline is needing to use it.”
The best way to learn what we need to know is by getting started, then picking up new knowledge as it proves itself necessary. When there’s an urgent need, we learn faster and avoid unnecessary learning. The same can be true for too much reading:
“Too much book learning may crab and confine the imagination, and endless poring over the research of others is sometimes psychologically a research substitute, much as reading romantic fiction may be a substitute for real-life romance….The beginner must read, but intently and choosily and not too much.”
We don’t talk about this much at Farnam Street, but it is entirely possible to read too much. Reading becomes counterproductive when it serves as a substitute for doing the real thing, if that’s what someone is reading for. Medawar explains that it is “psychologically most important to get results, even if they are not original.” It’s important to build confidence by doing something concrete and seeing a visible manifestation of our labors. For Medawar, the best scientists begin with the understanding that they can never know anything and, besides, learning needs to be a lifelong process.
The secrets to effective collaboration
“Scientific collaboration is not at all like cooks elbowing each other from the pot of broth; nor is it like artists working on the same canvas, or engineers working out how to start a tunnel simultaneously from both sides of a mountain in such a way that the contractors do not miss each other in the middle and emerge independently at opposite ends.”
Instead, scientific collaboration is about researchers creating the right environment to develop and expand upon each other’s ideas. A good collaboration is greater than the sum of its parts and results in work that isn’t attributable to a single person.
For scientists who find their collaborators infuriating from time to time, Medawar advises being self-aware. We all have faults, and we too are probably almost intolerable to work with sometimes.
When collaboration becomes contentious, Medawar maintains that we should give away our best ideas.
Scientists sometimes face conflict over the matter of credit. If several researchers are working on the same problem, whichever one finds the solution (or a solution) first gets the credit, no matter how close the others were. This is a problem most creative fields don’t face: “The twenty years Wagner spent on composing the first three operas of The Ring were not clouded by the fear that someone else might nip ahead of him with Götterdämmerung.” Once a scientific idea becomes established, it becomes public property. So the only chance of ownership a researcher has comes by being the first.
However, Medawar advocates for being open about ideas and doing away with secrecy because “anyone who shuts his door keeps out more than he lets out.” He goes on to write, “The agreed house rule of the little group of close colleagues I have always worked with has always been ‘Tell everyone everything you know,’ and I don’t know anyone who came to any harm by falling in with it.”
How to handle moral dilemmas
“A scientist will normally have contractual obligations to his employer and has always a special and unconditionally binding obligation to the truth.”
Medawar writes that many scientists, at some point in their career, find themselves grappling with the conflict between a contractual obligation and their own conscience. However, the “time to grapple is before a moral dilemma arises.” If we think an enterprise might lead somewhere damaging, we shouldn’t start on it in the first place.
We should know our values and aim to do work in accordance with them.
The first rule is never to fool yourself
“I cannot give any scientist of any age better advice than this: the intensity of the conviction that a hypothesis is true has no bearing of whether it is true or not.”
Richard Feynman famously said, “The first principle is that you must not fool yourself—and you are the easiest person to fool.” All scientists make mistakes sometimes. Medawar advises, when this happens, to issue a swift correction. To do so is far more respectable and beneficial for the field than trying to cover it up. Echoing the previous advice to always be willing to take no for an answer, Medawar warns about falling in love with a hypothesis and believing it is true without evidence.
“A scientist who habitually deceives himself is well on the way toward deceiving others.”
The best creative environment
“To be creative, scientists need libraries and laboratories and the company of other scientists; certainly a quiet and untroubled life is a help. A scientist’s work is in no way deepened or made more cogent by privation, anxiety, distress, or emotional harassment. To be sure, the private lives of scientists may be strangely and comically mixed up, but not in ways that have any special bearing on the nature and quality of their work.”
Creativity rises from tranquility, not from disarray. Creativity is supported by a safe environment, one in which you can share and question openly and be heard with compassion and a desire to understand.
A final piece of advice
“A scientist who wishes to keep his friends and not add to the number of his enemies must not be forever scoffing and criticizing and so earn a reputation for habitual disbelief; but he owes it to his profession not to acquiesce in or appear to condone folly, superstition, or demonstrably unsound belief. The recognition and castigation of folly will not win him friends, but it may gain him some respect.”