The European Conference on Computer Vision (ECCV), which began on Sunday, is held every other year, alternating with the International Conference on Computer Vision (ICCV). Scheduled for Glasgow this year, ECCV has, like most of the summer’s major computer science conferences, gone virtual.
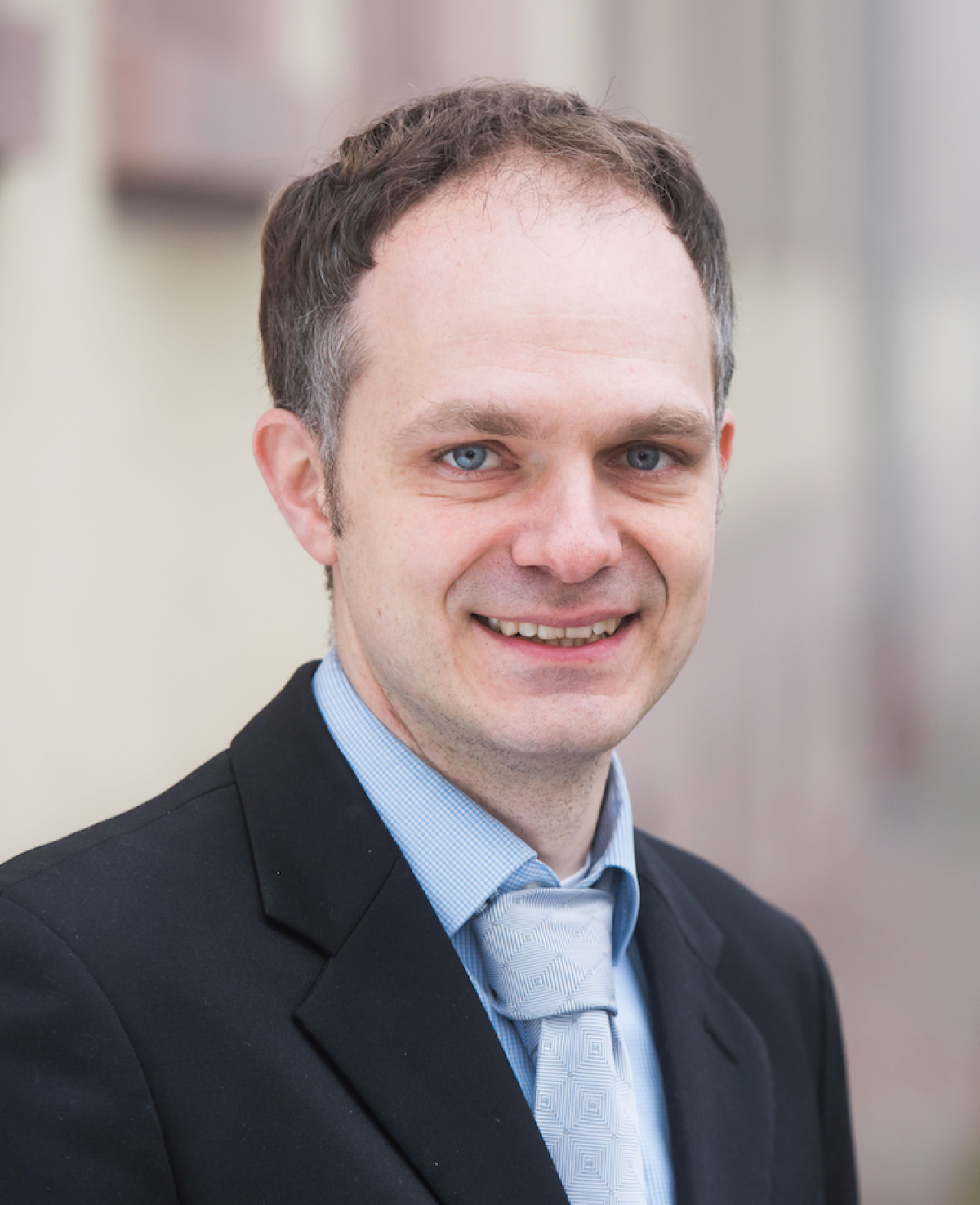
Together with CVPR (the IEEE Conference on Computer Vision and Pattern Recognition), ICCV and ECCV round out the big three of computer vision conferences.
“In the past, ECCV tended to be a bit more on math and 3-D geometry than CVPR, which was always a bit more in the direction of pattern recognition,” says Thomas Brox, an Amazon Scholar and professor of computer science at the University of Freiburg, who is a program chair at this year’s ECCV. “But since, these days, everything is pattern recognition and deep learning, they’re more similar.”
Brox’s first ECCV was in 2004, when he was still a graduate student, so he had been attending for 10 years when the deep-learning revolution in computer vision began.
“I like it if things get simpler,” Brox says. “So I liked that time a lot — 2014, 2015, this was when many computer vision problems simplified a lot suddenly. You took a network — it didn’t matter much what — and you always got a much better performance than everyone else got before.
Check out our #ECCV2020 Conference Opening Session here https://t.co/fcvF6YgQZG Congratulations to the Award winners :-)
— European Conference on Computer Vision (ECCV) (@eccvconf) August 24, 2020
“Of course, now everyone has done that, and it’s getting pretty complicated again. It’s about changing a few details in your network, how you train, how you collect the data, how you present it, and then you get your little incremental improvements.
“Progress on benchmarks is still relatively fast, but progress on concepts is relatively slow. In the past, when that’s been the case, then at some point progress on the benchmarks has stopped, too. During my postdoc in 2010, it was the same situation with object detection. There was a lot of progress before that, and then it became slower and slower, and no one had a good idea of what to do. And then deep learning came and solved the problem, more or less.”
Ten years later, with deep learning, too, “there’s very little conceptual novelty,” Brox says. “I think we’re hitting a wall.”
Getting a foothold
Of course, no one knows what the next conceptual breakthrough will be: “If anyone knew, we would all be doing it,” Brox says with a laugh. But he’s willing to hazard a few guesses.
“One bet might be that you want to go a bit away from these label annotations, because they might actually limit you more than they help,” Brox says. Today, most machine learning is supervised, meaning that it involves labeled training examples, and the machine learning model learns to predict the labels on the basis of input features. Training with unlabeled data is known as unsupervised.
Amazon Scholars
The Scholars program is designed for academics who want to apply research methods in practice and help us solve hard technical challenges without leaving their academic institutions. Learn more about the program here.
“As soon as you work on unsupervised losses, then you’re back in the old days, in a way,” Brox says. “We formulated the same kinds of losses. But there was no deep network, and the optimization techniques worked directly on the output variables rather than the network parameters. It needs something else on top, but something in this direction can be interesting.”
Another technique that intrigues Brox is the use of generative models, rather than the discriminative models that prevail today. Given two variables — say, visual features of images and possible labels of objects in those images — discriminative models, such as today’s neural nets, estimate the value of one variable given a specific value of the other: if the image feature is a pointy ear, the label is likely to be “cat”.
A generative model, by contrast, attempts to learn a probability distribution that relates all possible values of one variable to all possible values of the other. As such, it offers a statistical model of the world, rather than a bag of tricks for performing classifications.
“Discriminative models just try to find features that separate two different classes, and you’re happy if you can discriminate them,” Brox says. “Whereas with generative models, you also want to explain what you see. If you can explain what you see, then you have potentially much more robust models that also generalize better.
“That is a direction that is very interesting, but it is not currently competitive. When you come with a new concept, it will typically not be as good as all these well-optimized methods that optimize all the details. It’s similar to what the deep-learning people faced for many years, when they were already convinced that they had the right tool, but no one in the computer vision community wanted to believe them, because their numbers were much worse. You really have to believe in your strategy to go on with it and make it better until you hit the state of the art.”
Geometry returns
In his own work, Brox is also investigating the possibility of integrating deep learning with the “math and 3-D geometry” that used to be a priority at ECCV. In particular, he’s looking at using the motion of objects to infer information about their 3-D shape, an approach that would seem to benefit from rigorous computational methods for correlating points on an object’s surface under different rotations.
“In the motion signal, there’s a good deal of information, and it’s not well used in the current techniques,” Brox says. “Especially when you think of unsupervised learning, this might be quite useful. Making better use of 3-D structure has also been one of my interests.
“At the beginning, nobody believed that 3-D vision could be captured by deep learning. Everybody thought, ‘Okay, these two fields are incompatible, so if you work on 3-D vision, you’re safe.’ You don’t need to shift to deep learning.
“Actually, that’s not true. There are also benefits if you use deep learning for 3-D vision. But you can’t do everything with deep learning there. It’s more a mixture of classical geometry, classical math, and using deep learning for the pattern recognition parts of it. The combination of both is quite promising.”