The Association for the Advancement of Artificial Intelligence (AAAI), whose annual conference begins this week, had its first meeting in 1980. But its AI lineage goes back even farther: two of its first presidents were John McCarthy and Marvin Minsky, both participants in the 1956 Dartmouth Summer Research Project on Artificial Intelligence, which launched AI as an independent field of study.
Like all AI conferences, AAAI was transformed by the deep-learning revolution, which many people date to 2012, when Alex Krizhevsky, Ilya Sutskever, and Geoff Hinton’s deep network AlexNet won the ImageNet object recognition challenge with a 40% lower error rate than the second-place finisher.
Given the 10-year anniversary of that paper, and given that, in its long history, AAAI has seen AI research trends come and go, Amazon Science thought it might be a good time to contemplate what comes after the deep-learning revolution. So we asked Nikko Ström, a vice president and distinguished scientist in the Alexa AI organization, for his thoughts.
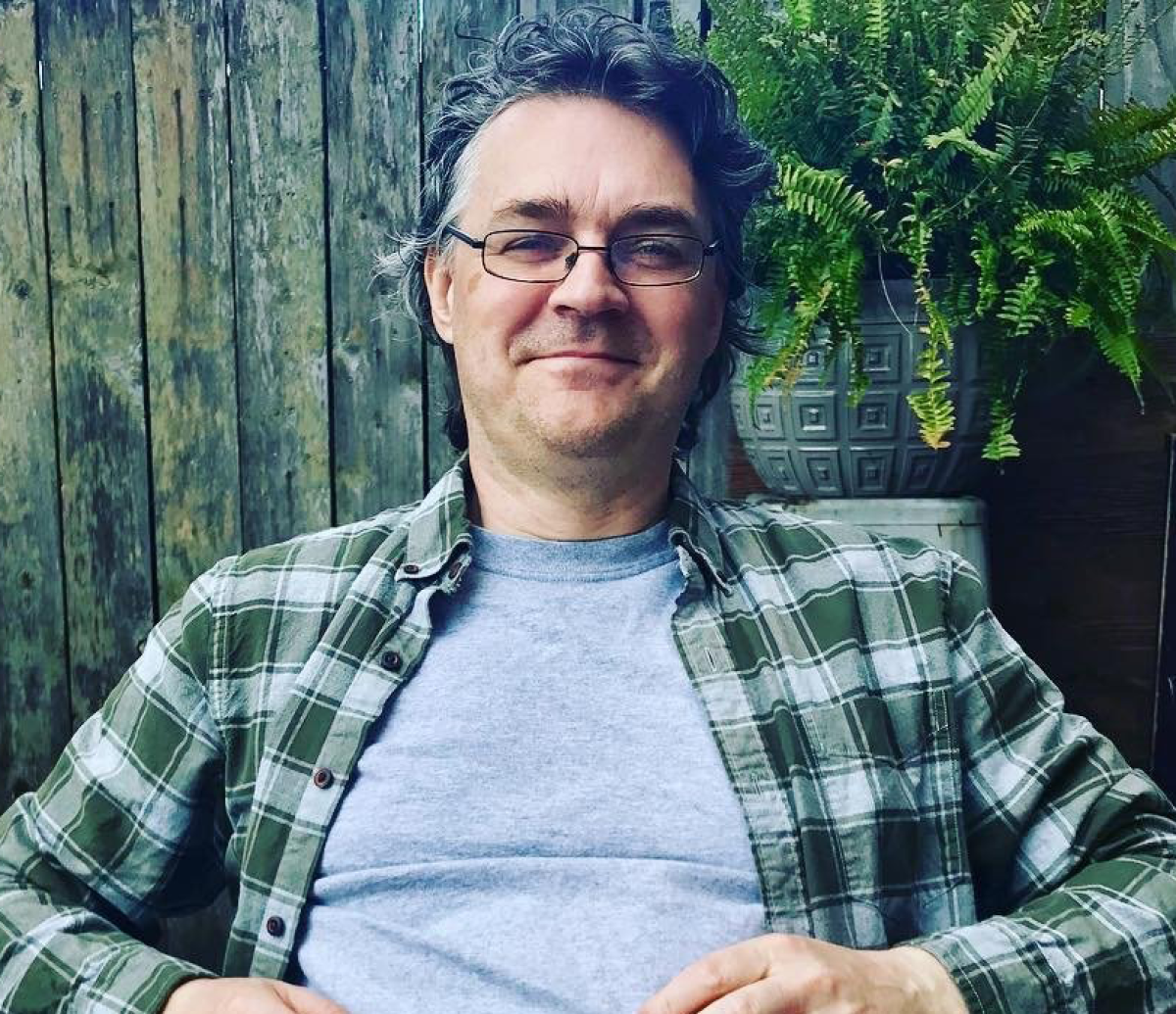
To begin with, Ström contests the dating of the revolution’s inception.
“Modern deep learning started around 2010 in Hinton’s lab,” Ström says. “Speech was the first application. There was a step function in accuracy, just like in image processing. Speech recognition systems around that time got 30% fewer errors from one year to the next because they started using these methods. Computer vision is a little bit of a bigger field than speech recognition, and visualizing problems is an easy way to understand them. So maybe that's why it's easier to get started with something like ImageNet or a vision task.”
Second, Ström thinks that the question of what will come after deep learning may be ill posed, because the definition of deep learning keeps evolving to incorporate new AI innovations.
“There’s a famous quote about Lisp in the 1970s by Joel Moses,” Ström says. “‘Lisp is like a ball of mud. Add more and it's still a ball of mud — it still looks like Lisp.’ The moniker ‘deep learning’ has been applied to many different types of models over time, it’s starting to resemble a ball of mud accumulating all of AI.
“In the beginning, when we worked on speech and computer vision classification tasks, no one had really thought about generative models like GANs, so that's one very different thing that we still call deep learning. The AlphaGo system combined deep learning with other things, like a probabilistic belief tree. The deep learning in chess or in go is really good at evaluating a board position, but there's also the looking forward: If I make this move, the board will look like that. Is that a good position? So it's not just deep learning; it's also evaluating all the branches of a tree.
“And then applying deep neural networks to reinforcement learning became important. So there are many different aspects of AI that have been brought in, and now we call it all deep learning.”
Symbolic reasoning
The history of AI research is sometimes characterized as a tug-of-war between two different approaches, symbolic reasoning and machine learning. In AAAI’s first decade, symbolic reasoning predominated, but machine learning began to make inroads in the 1990s, and with the deep-learning revolution, it took over the field.
But, Ström says, symbolic reasoning is just another set of methods that the expanding mudball of deep learning may end up consuming.
“Transformer networks have something called attention,” Ström says. “So you can have a vector in the network, and we can have the network attend to that vector more than all the other information. If you have a knowledge base of information, you can prepopulate that with vectors that represent truth in that knowledge base. And then you can have the network learn to attend to the right piece of knowledge depending on what the input is. That is how you can try to combine structured world knowledge with the deep-learning system.
“There are also graph neural networks, which can represent knowledge about the world. You have nodes, and you have edges between the nodes that are the relations between the nodes. So, for example, you can have entities represented in the nodes and then relations between the entities. We can use attention to zero in on the part of the knowledge graph that is important for the current context or question.
“In a very abstract sense, I think we know that we can represent all knowledge in a graph. It's just, how can we do it in an efficient way that's suitable for the task?
“Hinton had this idea a long time ago; he called it a thought vector. Any thought that you can have, we can represent with a vector. The reason that's interesting is that, we can represent anything in the graph, but to have that work well in unison with a deep-learning model, we also have to have, on the other side, something that we can represent anything with. And that happens to be vectors. So we can map between the two.”
Interactive learning
Assuming that the deep-learning paradigm will continue to absorb other computational approaches, the major drawback of the paradigm itself, Ström says, is the inefficiency of its learning. Human beings, after all, don’t need a million examples to learn to recognize a new animal.
That kind of inefficiency may be acceptable when the learning process involves a bank of computers churning away for days or weeks on data store on their own hard drives. But it’s totally impractical if an AI agent is trying to learn from direct interactions with the world. And that kind of interactive learning is, in Ström’s view, one of the major research challenges for AI today.
“The deep-learning system doesn't have all the prior knowledge that we have,” Ström explains. “It doesn't know that the dog in the image lives in a three-dimensional world that can spin, and we have an idea about what it looks like on the other side because we assume it's symmetrical, and things like that.
“Of course, networks are being trained specifically to be able to do these kind of things — rotate the dog so you can see the backside. But I think mostly it learns that from training on data. If you know the symmetries, you can generate that data using CGI: you have a model of a dog, and you spin it around and input that as training data and the system will learn the concept of the 3-D world and the spinning dog.
“There's probably some algorithmic innovation that's needed in that area. But I'm optimistic. It's evolutionary: there are so many people working on this all over the world now that, even if it's a bit random, someone will come up with some good ideas, and they’ll combine, and eventually we'll have something.”