Using Alexa, customers can control thousands of different smart-home devices — such as lights, plugs, switches, door locks, cameras, and thermostats — with just their voices. But the Alexa smart-home interface is more than just a voice-based remote control.
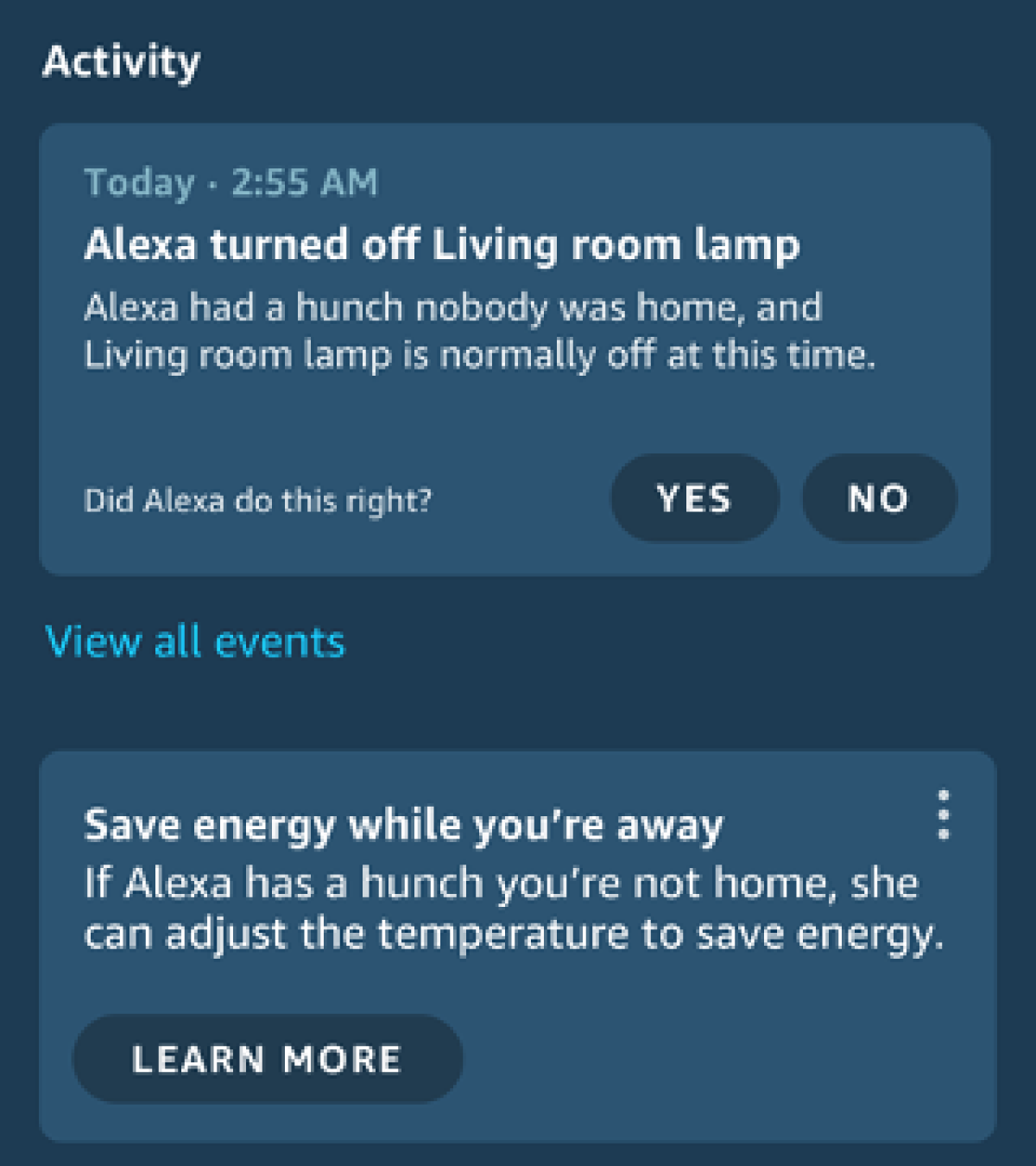
In 2018, we launched Hunches, an Alexa setting that offers reminders when Alexa thinks you forgot to turn off a light or to lock a door. Since then, we have expanded Hunches to include a proactive experience called Automatic Actions: we turn off lights and adjust thermostats when customers are asleep or leave the home. Today, thanks to predictive and proactive features like Hunches and Routines, one in four smart-home interactions is initiated by Alexa — without customers’ having to say anything.
Taking proactive actions in the home is a responsibility we take seriously, and our machine learning models need to clear a high bar in order to meet customer expectations. Before initiating an action, multiple models make decisions, including estimating the light level, predicting whether customers have gone to sleep or left the home, and predicting which devices should be controlled.
Each of these components is an active area of research and innovation, but in this post, I’m going to focus on how we decide which device to control. We do this by mapping customers' smart-home usage patterns to a set of archetypes.
Smart-home usage patterns
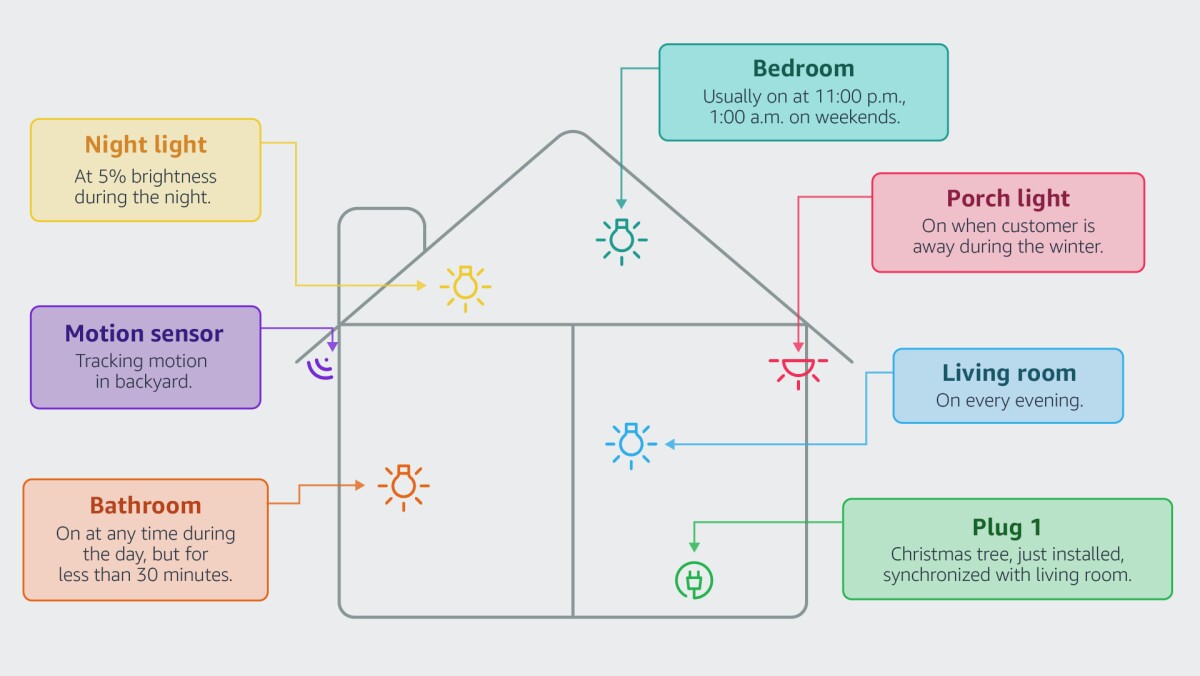
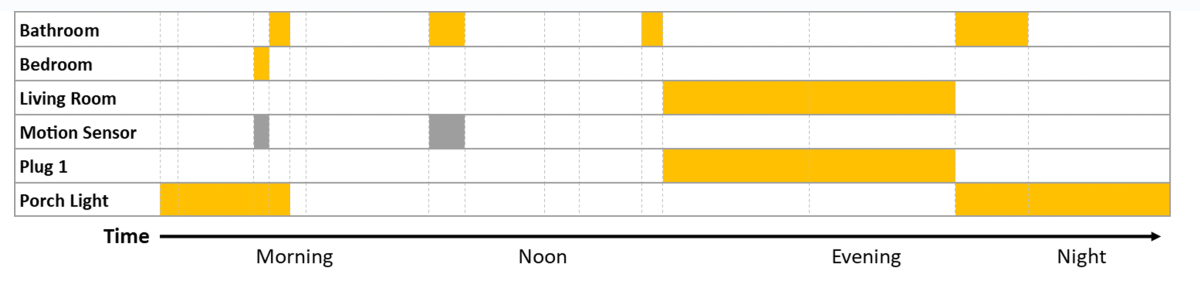
We derive insights about a device by how it is named and grouped, as well as by how it is used. Consider the time periods of the “on” states of the example devices in the image of state data timelines. We see the typical bathroom-light pattern of short spans of on-state over the day and longer spans in the evening.
Another device in the living room is mostly used in the evening. A third device is just called “Plug 1”, but it is highly correlated with the usage behavior in the living room. Alexa may infer that this plug is located in the living room. It could, for example, be attached to a Christmas tree. If our model knew this for certain, it could offer the customer a Routine that synchronizes the tree lights with holiday music.
Two factors make proactive routines challenging:
- Sparsity: While some smart homes are fully wired, other customers may own one Echo Dot and a single smart light bulb. We want to offer them Hunches, too.
- Diversity: There are hundreds of thousands of possible configurations for Echo devices, smart-home appliances, and their uses. Even if two homes are configured identically, customers may be using their devices very differently. For example, a device labeled “bedroom light” may be in the main bedroom or a guest bedroom, or it may just be a closet light.
Deep device embeddings model
Many of our services boil down to the question of what state customers would like their devices to be in: that’s what drives both Automatic Actions and Hunches. To answer that question, we train an encoder model that produces a device representation — an embedding — that encodes the device’s previous states and the configuration of the home, including device names, device types, and device groupings. From that embedding, a decoder model can predict the device’s future state.
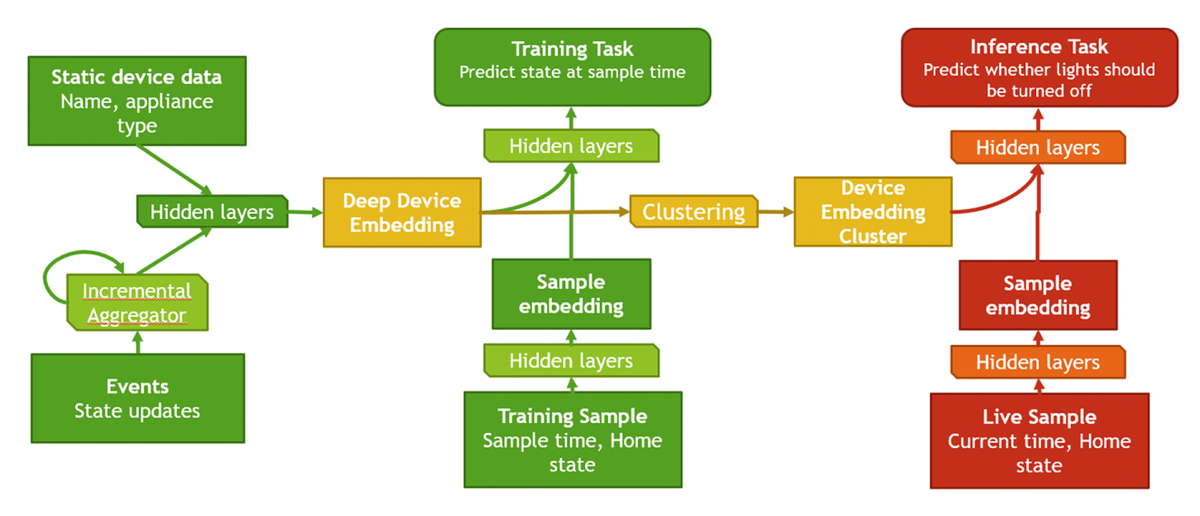
But predicted home state does not directly answer questions like whether a light should be turned off or a Hunch should be sent. For that purpose, we use a model trained to predict whether a given Hunch will be accepted or not. The Hunch acceptance model uses the home state prediction model’s encoder, which can compute per-home or per-device embeddings. We term the per-device embeddings the deep device embeddings.
In this model, the weights of the embedding encoder represent information collected across customers; devices with similar usage patterns yield representations that are close to one another in the embedding space. Some bathroom lights, for instance, may bear the label “bathroom light”, but others may be identified by device grouping or usage patterns. Using an embedding space allows us to abstract away these individual features.
From embeddings to semantics
While deep device embeddings are useful to predict Hunches, we also find that the embedding space has some interesting properties when visualized.
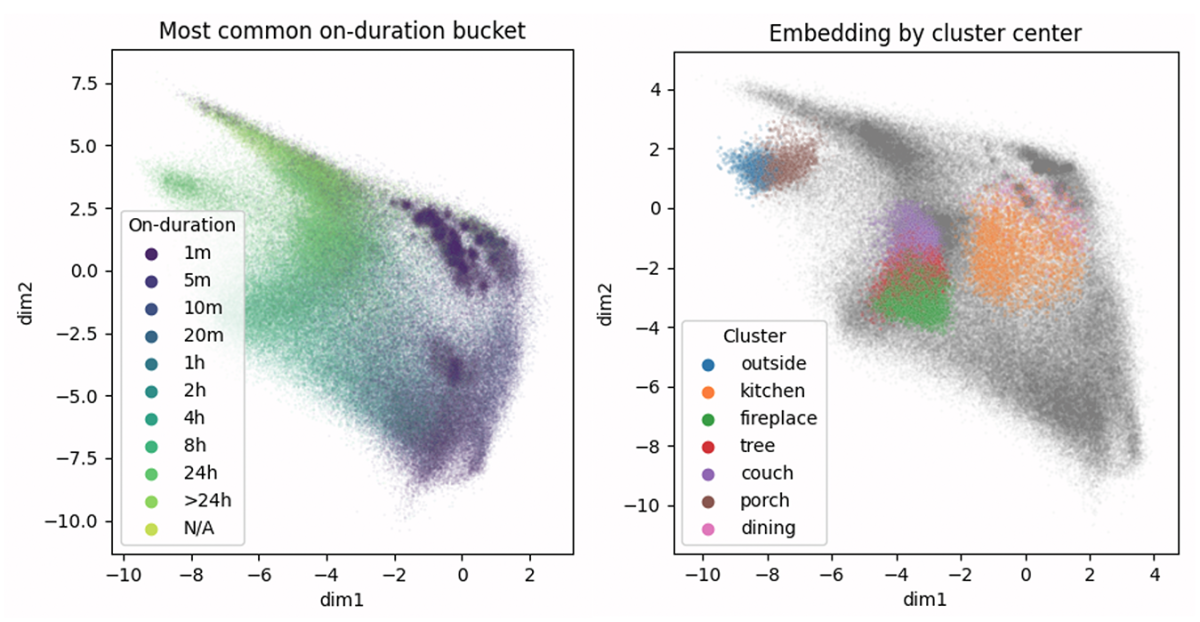
What’s interesting about this space is that there are clusters to which we can attach usage semantics. In our sample visualization, we define 60 clusters using k-means and color some of them. The title of each cluster is the name in it that is most frequent when normalized by its frequency in the complete dataset. We find that clusters carry semantic information; for example, the isolated island of devices consisting of the “outside” and “porch” (blue and brown) clusters is most likely outdoor devices that are left on for long durations at a time.
While the labels given to clusters are the dominant names, they are not the only names associated with the clusters. For instance, we also find devices labeled “First Light” in the outdoor cluster. The embeddings are able to tag devices not just by how they are named and grouped but also based on their usage.
The device embedding space thus provides an automated way to map device usage patterns in different homes into a common space that can be used to drive services such as Automatic Actions or Hunches.
Outlook
For all the convenience offered by Hunches and Automatic Actions, we still see opportunities to make the smart home smarter.
For example, we project all customer device usage into common roles. While this can be helpful when data is sparse, we would also like to offer customers more personalized smart-home services. We recently implemented a new representation called deep home embeddings, which aggregates information across all devices in the home and enables us to create proactive automations that consider the state of the home holistically.
Most smart homes start out with just one or two Internet-connected devices and add more over time. We are always trying to find ways to automate repetitive tasks, provide relevant reminders that increase customer convenience, and offer useful recommendations for all Alexa Smart Home customers.
Acknowledgments: Charles Brett, Maisie Wang, Michael Dillon, Paul Savastinuk, Usman Aleem