With every new Echo device and upgrade, we challenge ourselves to bring the best audio experience to our customers at an affordable price. This year, we’re introducing Amazon’s own custom-built spatial audio-processing technology, designed to enhance stereo sound on compatible Echo devices.
The version of the technology on Echo Studio, for instance, is customized to the specific acoustic design of the speakers and employs digital-processing methods — such as upmixing and virtualization — so stereo audio, television shows, and movie soundtracks feel closer to the listener, with greater width, clarity, and presence. It turns the Echo Studio into a hi-fi audio system that mirrors that of a stereo reference arrangement. Vocal performances are more present in the center soundstage, and stereo panned instruments are better defined on the sides, thereby creating a more immersive sound experience that reproduces the artist's intent.
In this blog post, we break down how we built this spatial audio-processing technology with an emphasis on the way humans perceive sound — or psychoacoustics — by using a combination of crosstalk cancellation, speaker beamforming, and upmixing to create a room-filling, spatial audio experience.
Psychoacoustics: Width, depth, and listening zones
Throughout development, we characterize the stereo image by its psychoacoustic qualities, including width, depth, and listening zones. We then investigate how sound waves interact with listeners in various room shapes and sizes and how signal-processing methods affect the listener’s experience.
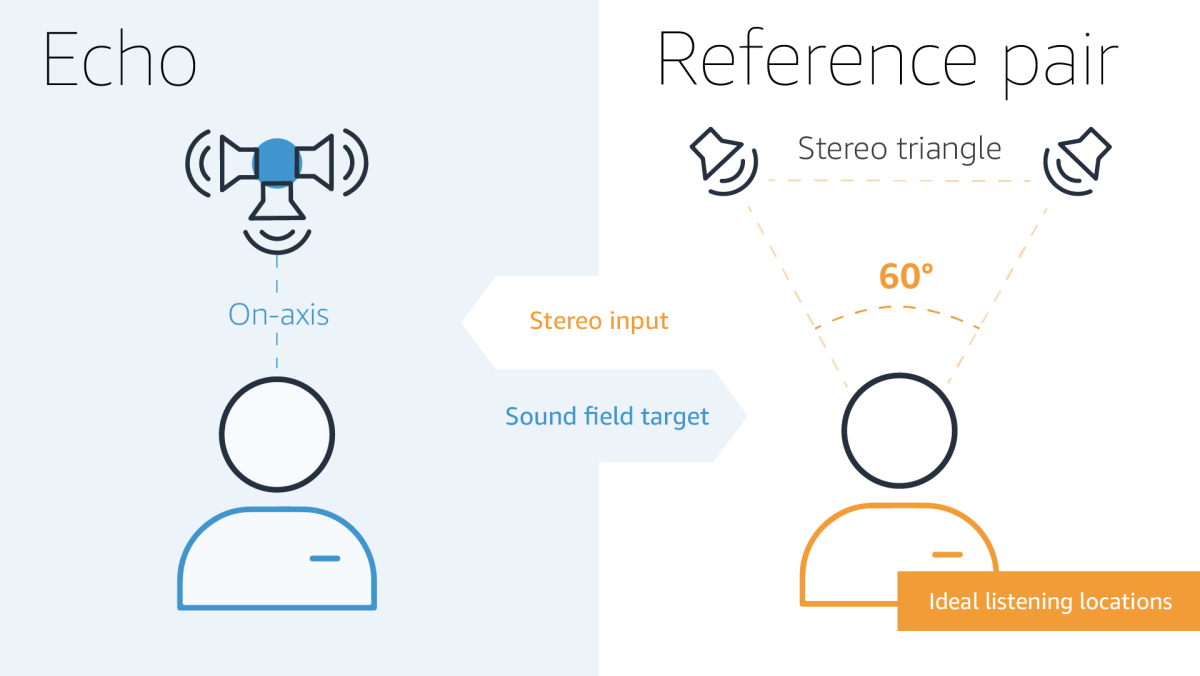
Width
Width: The angular extent (wide vs. narrow) of localizable elements in the stereo image along the horizontal — or azimuth — plane.
When determining the width of a sound field, we first consider localizable elements such as a point-source that would induce time and level differences in the acoustic responses at the listener’s two ears. To model this phenomenon, it is helpful to compare the listening experiences on headphones vs. a loudspeaker in terms of the separation of left and right ear responses.
Unlike loudspeaker listening, headphone listening lacks a crosstalk path, as illustrated in the image below. In order to make headphone listening realistic, we can model crosstalk from the point-source to the two ears using an all-pass signal-processing filter for one ear and a delayed low-pass filter for the other ear. The two filters approximate and parameterize the listener’s ear responses with respect to their relative head-related transfer functions (HRTFs), which contain important cues that the human ear uses to localize sound. Moreover, the filter design ensures that there’s minimal modification to the signal spectra — or tonal balance — and therefore preserves the original playback content.
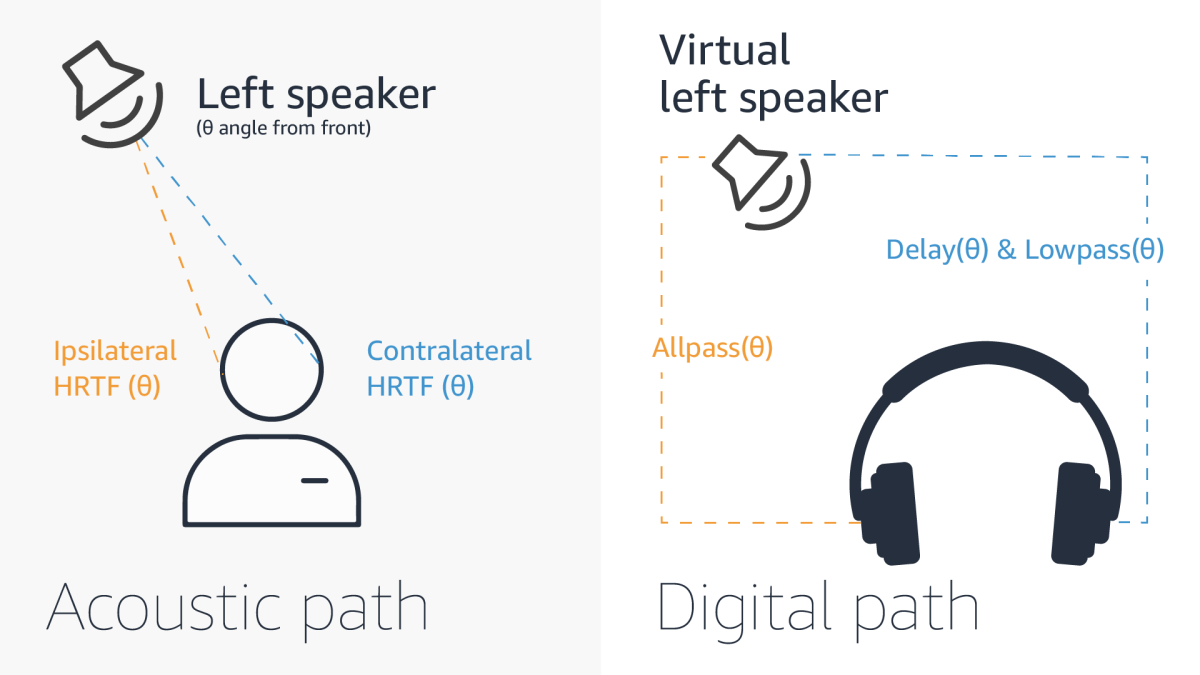
However, unlike headphones, an external speaker can create its own crosstalk for the listener, depending on its placement. For example, the left and right speaker transducers, or drivers, on the Echo Studio are narrowly spaced within the device, whereas the speakers in a standard stereo pair are 60 degrees apart relative to the listener.
With the spatial audio-processing technology on Echo Studio, we decouple the crosstalk of the driver pair by modeling and then inverting the system of equations between each driver and the listener’s ears, via crosstalk cancellation (CTC) methods. If we have more than two drivers, then the more general formulation is called null-steering, where filters are designed for all the drivers so that their acoustic responses cancel at one ear.
In both cases, we can normalize the filter design to satisfy a target cancellation gain curve defined by the power ratio of the acoustic energy at the ipsilateral (same side of the body) and contralateral (opposite side of the body) ears across frequencies. This prevents overfitting the cancellation to an exact location, since a listener may be at varying distances or not perfectly centered to the device.
Once the driver’s CTC filters are designed for stereo inputs, they can be combined with the approximated HRTF filters that introduce the amount of crosstalk consistent with a stereo reference system.
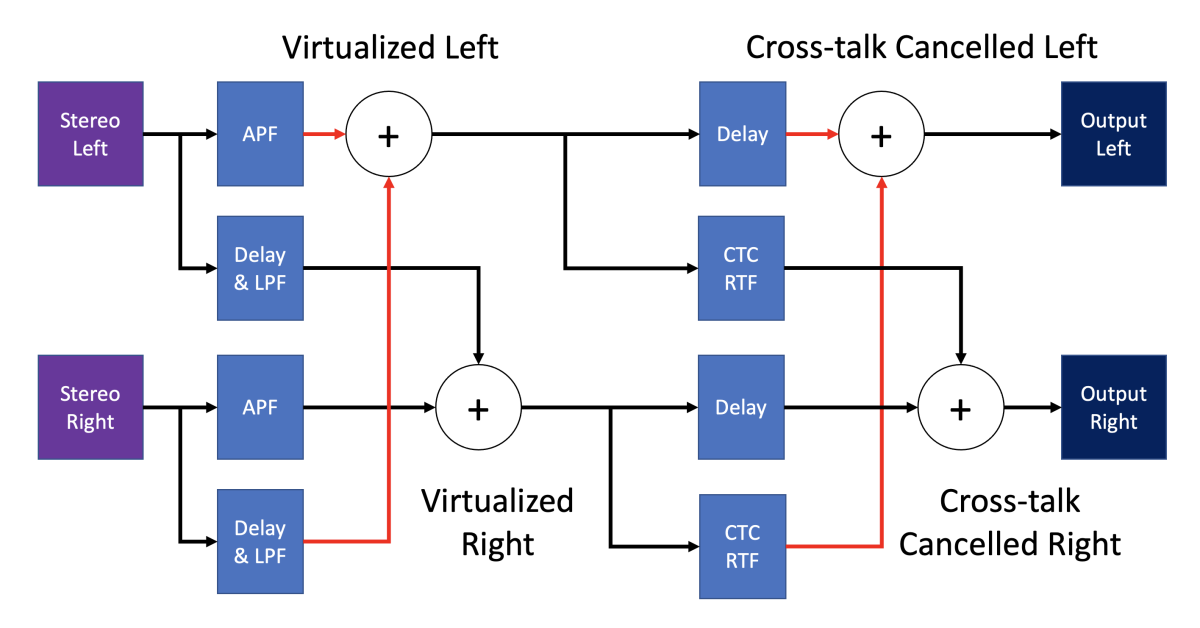
Depth
Depth: The distance (frontal vs. recessed) of the perceived sound field from the listener.
The distance at which sound elements in an audio track localize correlates with the relationship — or coherence — of the two signals between the sound source and the listener’s ears. For example, a simple left or right signal from a speaker is easy to understand, but if the audio mixes with the room’s reverberation, the audio clarity decreases, and the audio sounds recessed.
In speaker playback, however, we contend with the speaker directivity and its interaction with the room environment. For example, a direct acoustic path between a speaker and a listener preserves the desired clarity of the original content. But when the acoustic signal reflects off of walls, the loss in coherence recesses the perceived sound field and causes elements to smear spatially. This is why tracks heard anechoically or on headphones appear closer — or even inside the listener’s head — and clearer than tracks heard over external speakers in a reverberant room. In the first case, the acoustic response is direct from the driver to the listener’s ears, while external speakers must contend with the effects of the room environment.
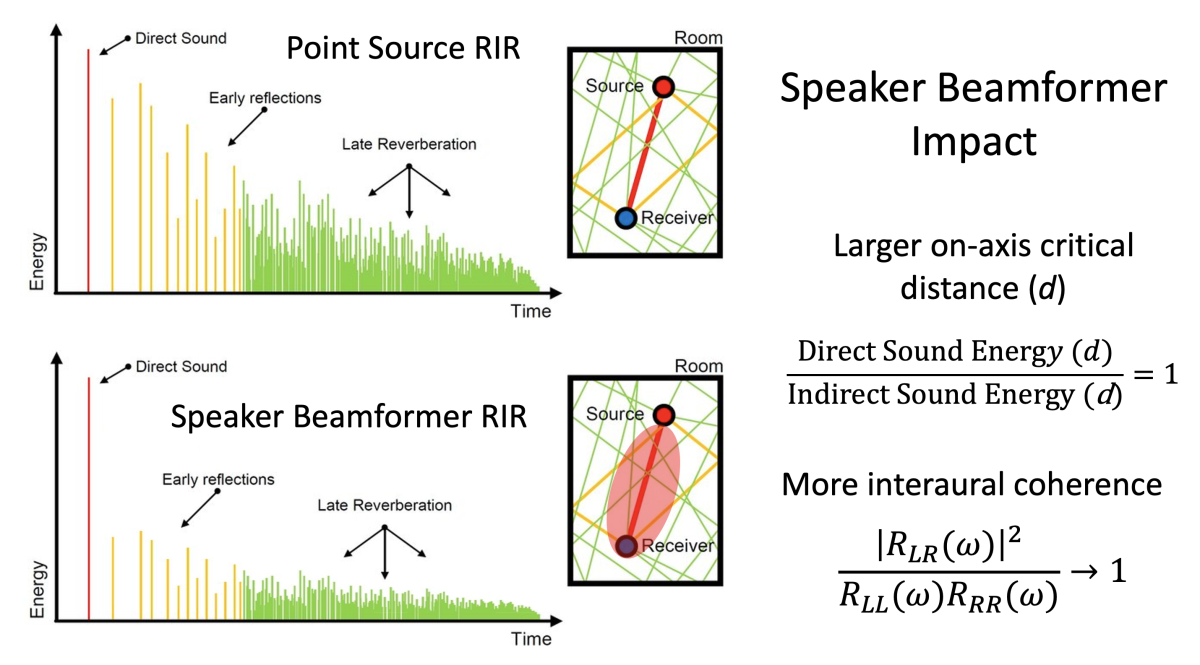
As part of our custom-built spatial audio technology, we can control the speaker directivity via careful beamforming. The speaker drivers can be filtered to produce a sound field with a directivity that sums coherently on-axis and cancels off-axis. That is, the acoustic response is greatest when the listener is lined up in front of the speaker and, conversely, weakest when the listener is to the side at +/- 90 degrees.
Therefore, one way to design with such directivity is to place two nulls at +/- 90-degree angles and either control for the cancellation gain between on-/off-axis power responses or the shape of the nulls as a function of azimuth. The resulting beam pattern is one with a main lobe that is wide enough for the direct path to be strong, at up to a +/- 45-degree azimuth listening window, before quickly tapering off to minimize the acoustic energy further off-axis, which would reflect off the walls.
This has the intended effect of making stereo audio feel closer to the listener, with greater clarity than is typical in an acoustically untreated listening environment like a living room. The effect is similar to how theaters reproduce a frontal soundstage over different seating areas, despite the speakers’ being far away.
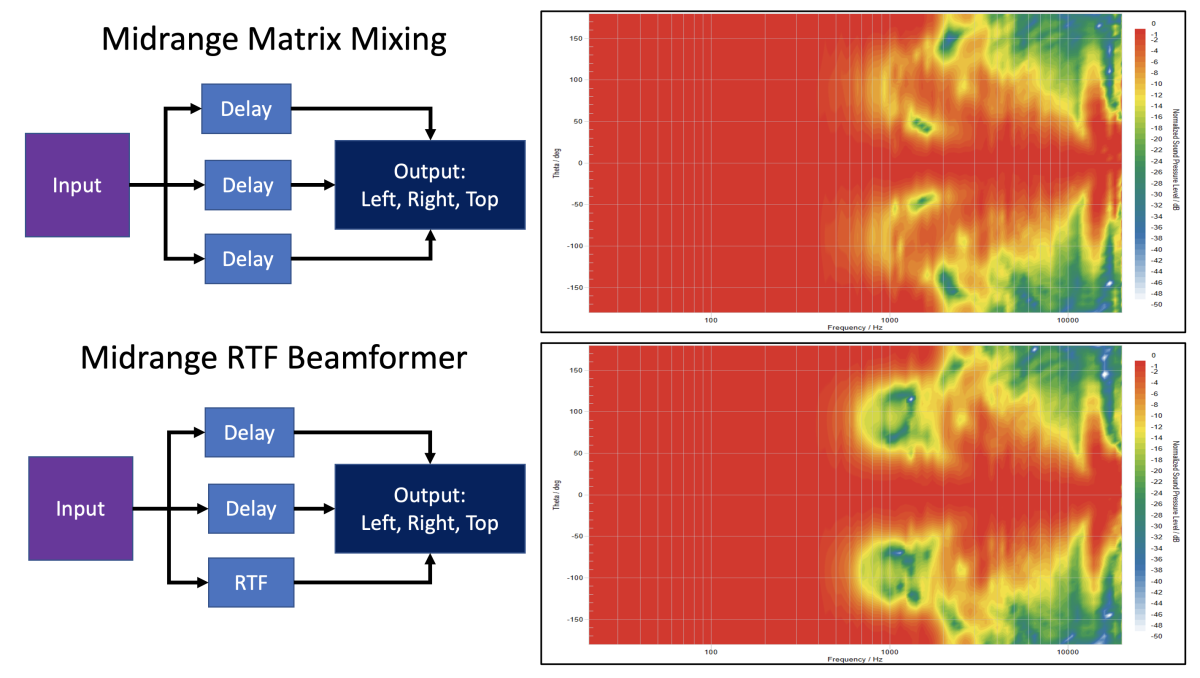
Listening zones
Listening zone: The mapping between the listening area and the stereo soundstage.
A listening “sweet spot” — the stereo image in a hi-fi audio system reference stereo pair — is best reproduced when the listener’s location forms an equilateral triangle with the stereo speaker pair. If the listener angle exceeds +/- 30 degrees, then a hole is created in the listener’s phantom center due to the loss of inter-speaker-to-ear coherence as room reflections grow stronger. Important elements of the audio mix, such as vocals, lose their presence. If the listener angle falls below +/- 30 degrees, then the stereo image narrows, as audio elements collapse toward the center. If the listener’s location is off-axis, then the stereo image biases towards one side or the other.
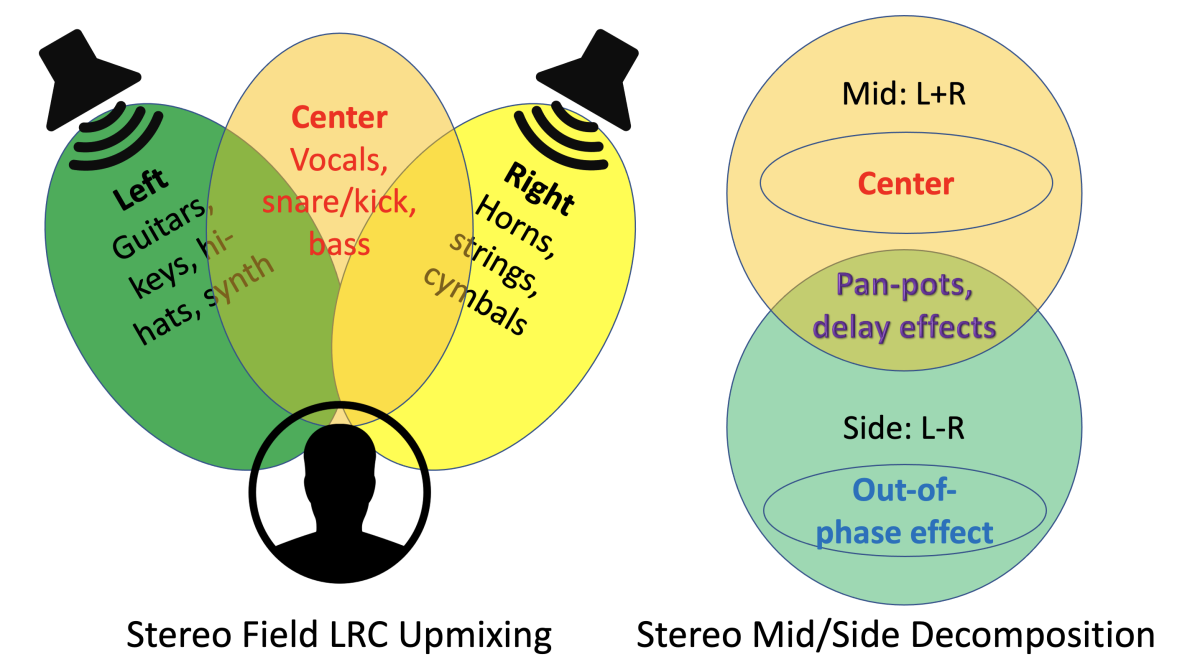
To combat this, our spatial audio technology aims to reproduce the stereo image over the largest listening area. In practice, the intended listening area of CTC-filtered playback conflicts with that of beamforming designs that control for speaker directivity. We can achieve a compromise by performing stereo upmixing and then applying different beamforming filters to each channel. For example, we can upmix into left, right, and center (LRC), where the center is minimally correlated with left-minus-right in the mid-/side decomposition.
The upmixed left channel is processed through the CTC filter that nulls the right ear after virtualization, the upmixed right channel nulls the left ear, and the center channel is beamformed with a wide main lobe. This means that vocal performances are more present in the center, while the stereo panned instruments are better defined on the side, creating a more immersive sound experience for the listener.
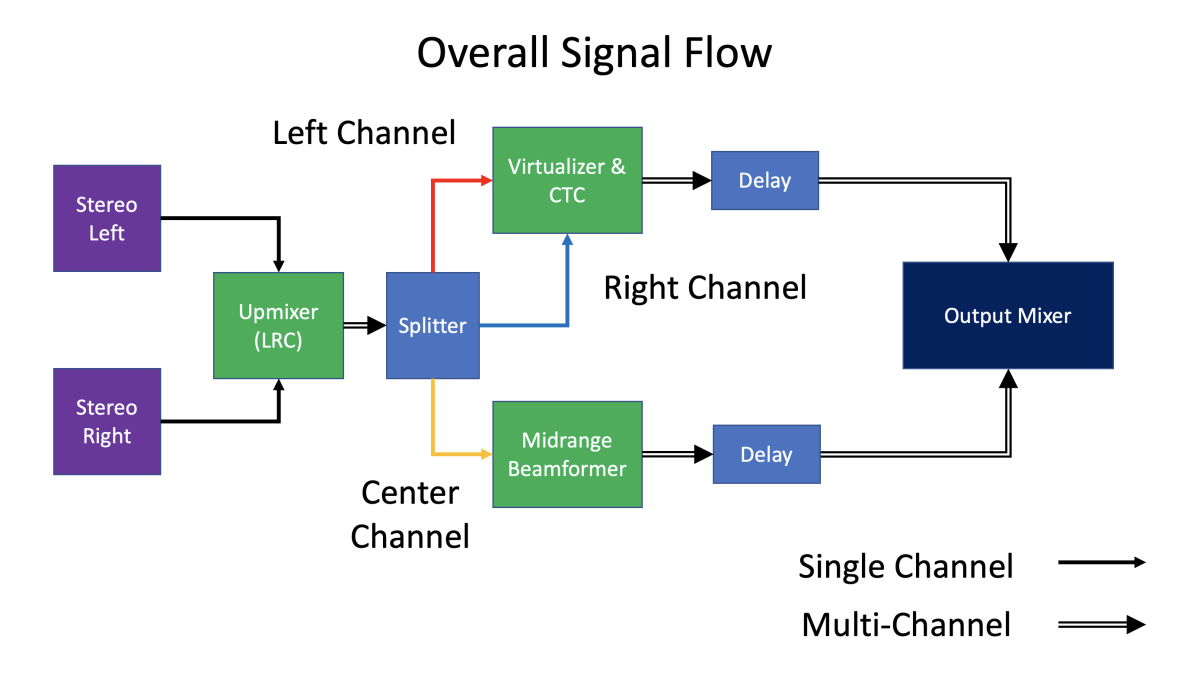
We’re continuing to iterate and refine technology across the Echo portfolio to bring the best audio experience to our customers. If you’d like to learn more about beamforming and speaker directivity in room acoustics, read papers published by our engineering team: “Fast source-room-receiver modeling”, in EUSIPCO 2020, and “Spherical harmonic beamformer designs", in EURASIP 2021.