With services like Alexa, which use synthesized speech for output, text normalization (TN) is usually the first step in the process of text-to-speech conversion. TN takes raw text as input— say, the string 6-21-21 — and expands it into a verbalized form that a text-to-speech model can use to produce the final speech — “twenty first of June twenty twenty one”.
Historically, TN algorithms relied on hard-coded rules, which didn’t generalize across languages and were hard to maintain: a typical rule-based TN system for a single language might have thousands of rules, which evolve over time and whose development requires linguistic expertise.
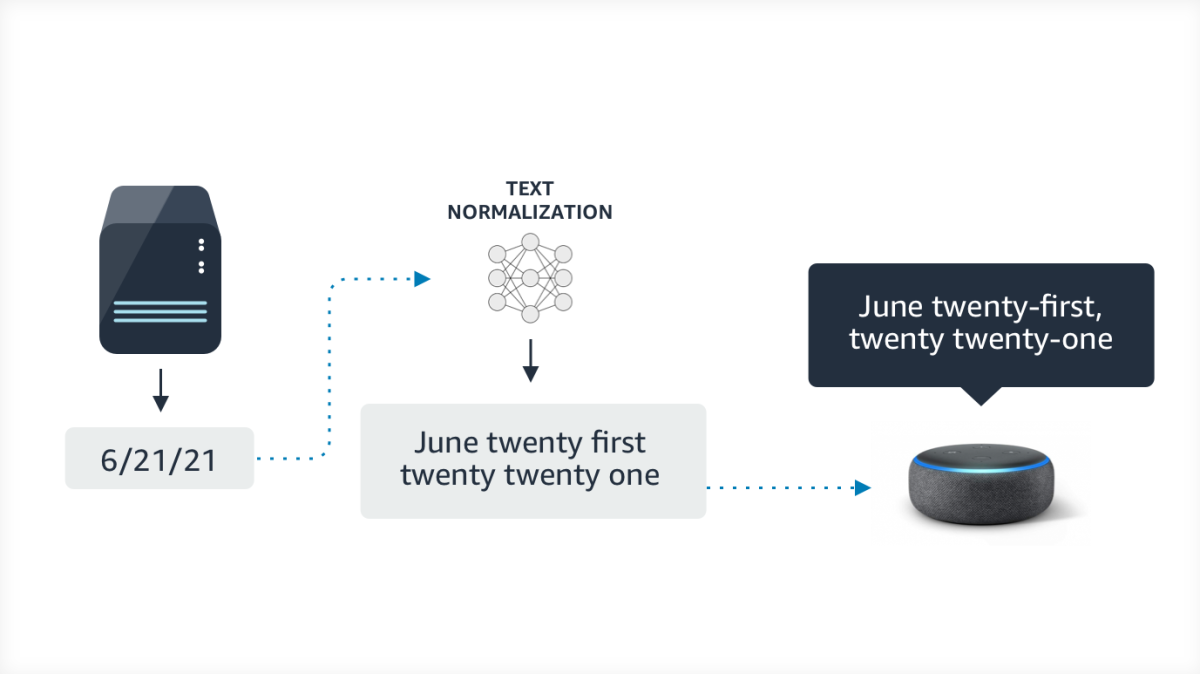
More recently, academic and industry researchers have begun developing machine-learning-based TN models. But these have drawbacks, too.
Sequence-to-sequence models occasionally make unacceptable errors, such as converting “$5” to “five pounds”. Semiotic-classification models require domain-specific information classes created by linguistic experts — classes such as emoticonor telephone number — which limits their generalizability. And both types of models require large amounts of training data, which makes it difficult to scale them across languages.
At this year’s meeting of the North American Chapter of the Association for Computational Linguistics (NAACL), my colleagues and I are presenting a new text normalization model, called Proteno, that addresses these challenges.
We evaluated Proteno on three languages, English, Spanish, and Tamil. There’s a large body of research on TN in English, but no TN datasets existed for Spanish and Tamil. Consequently, we created our own datasets, which we have publicly released for use by other TN researchers.
Proteno specifies only a few, low-level normalization classes — such as ordinal number, cardinal number, or Roman numeral — which generalize well across languages. From the data, Proteno then learns a huge variety of additional, fine-grained classes.
In our experiments on English, for instance, we used eight predefined classes, and Proteno automatically generated another 2,658. By contrast, semiotic-classification models typically have about 20 classes.
Proteno also uses a simple but effective scheme for tokenization, or splitting texts up into smaller chunks. Prior tokenization techniques required linguistic knowledge or data-heavy training; Proteno’s tokenization technique, by contrast, simply breaks text up at spaces and at transitions between Unicode classes, such as letter, numeral, or punctuation mark. Consequently, it can generalize across languages, it enables the majority of normalizations to be learned from the data, and it reduces the incidence of unacceptable errors.
Together, these techniques also allow Proteno to make do with much less training data than previous machine learning approaches. In our experiments, Proteno offered comparable performance with the previous state of the art in English — while requiring only 3% as much training data.
Because there were no prior TN models trained on Spanish and Tamil, we had no benchmarks for our experiments. But on comparable amounts of training data, the Proteno models trained on Tamil and Spanish achieved accuracies comparable to that of the one trained on English (99.1% for Spanish, 96.7% for Tamil, and 97.4% for English).
Methods
Proteno treats TN as a sequence classification problem, where most of the classes are learned. The figure below illustrates Proteno’s training and run-time processing pipelines, which have slightly different orders.
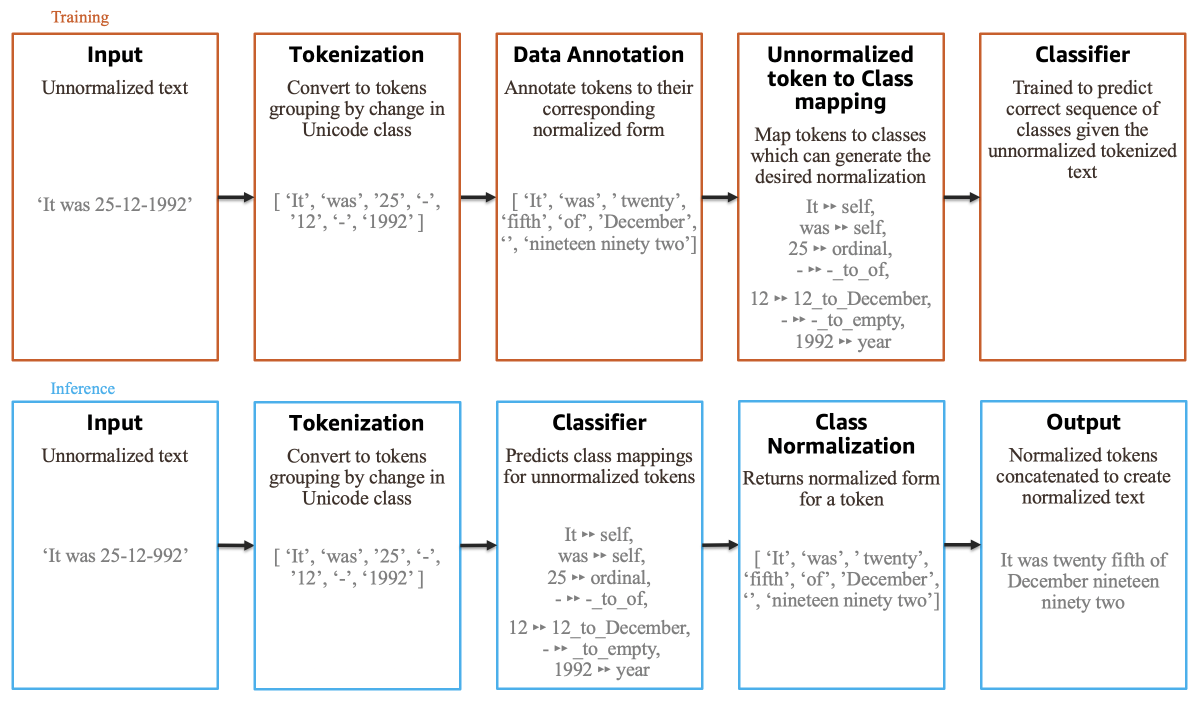
The training pipeline consists of the following steps:
- Tokenization: Previous tokenization methods relied on language-specific rules devised by linguists. For instance, the string 6-21-21 would be treated as a single token of the type date. We propose a granular tokenization mechanism that is language independent and applicable to any space-separated language. The text to be normalized is first split at its spaces and then further split wherever there is a change in the Unicodeclass. The string 6-21-21 thus becomes five tokens, and we count on Proteno to learn how to handle them properly.
- Annotation: The tokenized, unnormalized text is annotated token by token, which gives us a one-to-one mapping between each unnormalized token and its ground-truth normalization. This data will be used to train the model.
Class generation: Each token is then mapped to a class. A class may receive tokens only of a particular type; so, for instance, the class corresponding to dollars will not accept the type pounds, and vice versa. This prevents the model from making unacceptable errors. Each class also has an associated normalization function.
There are two kinds of classes:
- Predefined: We define a limited number of classes (around 8-10) containing basic normalization rules. A small subset of these (3-5) contain language-specific rules, such as how to distinguish cardinal and ordinal uses of a number. Other classes — such as self, digit, and Roman numerals — remain similar across many languages.
- Auto-generated (AG): The model also generates classes automatically by analyzing the unnormalized-to-normalized token mappings in the dataset. If no existing class (pre-coded or AG) can generate the target normalization for a token in the training data, a new class is automatically generated. For instance, if the dataset includes the annotation “12→December", and if none of the existing classes can generate this normalization, then the class “12_to_December_AG" is created. This class accepts only “12", and its normalization function returns “December". AGs enable Proteno to learn the majority of normalizations automatically from data.
- Predefined: We define a limited number of classes (around 8-10) containing basic normalization rules. A small subset of these (3-5) contain language-specific rules, such as how to distinguish cardinal and ordinal uses of a number. Other classes — such as self, digit, and Roman numerals — remain similar across many languages.
- Classification: We model TN as a sequence-tagging problem, where the input is a sequence of unnormalized tokens and the output is the sequence of classes that can generate the normalized text. We experimented with four different types of classifiers: conditional random fields (CRFs), bi-directional long-short-term-memory models (bi-LSTMs), bi-LSTM-CRF combinations, and Transformers.
Datasets
As the goal of Proteno is to be applicable to multiple languages, we evaluated it across three languages, English, Spanish, and Tamil. English had significantly more auto-generated classes than Tamil or Spanish, as written English tends to use many more abbreviations than the other two languages.
Language | Total predefined classes | Language-specific predefined classes | Auto-generated classes |
Spanish | 10 | 5 | 279 |
Tamil | 8 | 3 | 74 |
English | 8 | 4 | 2,658 |
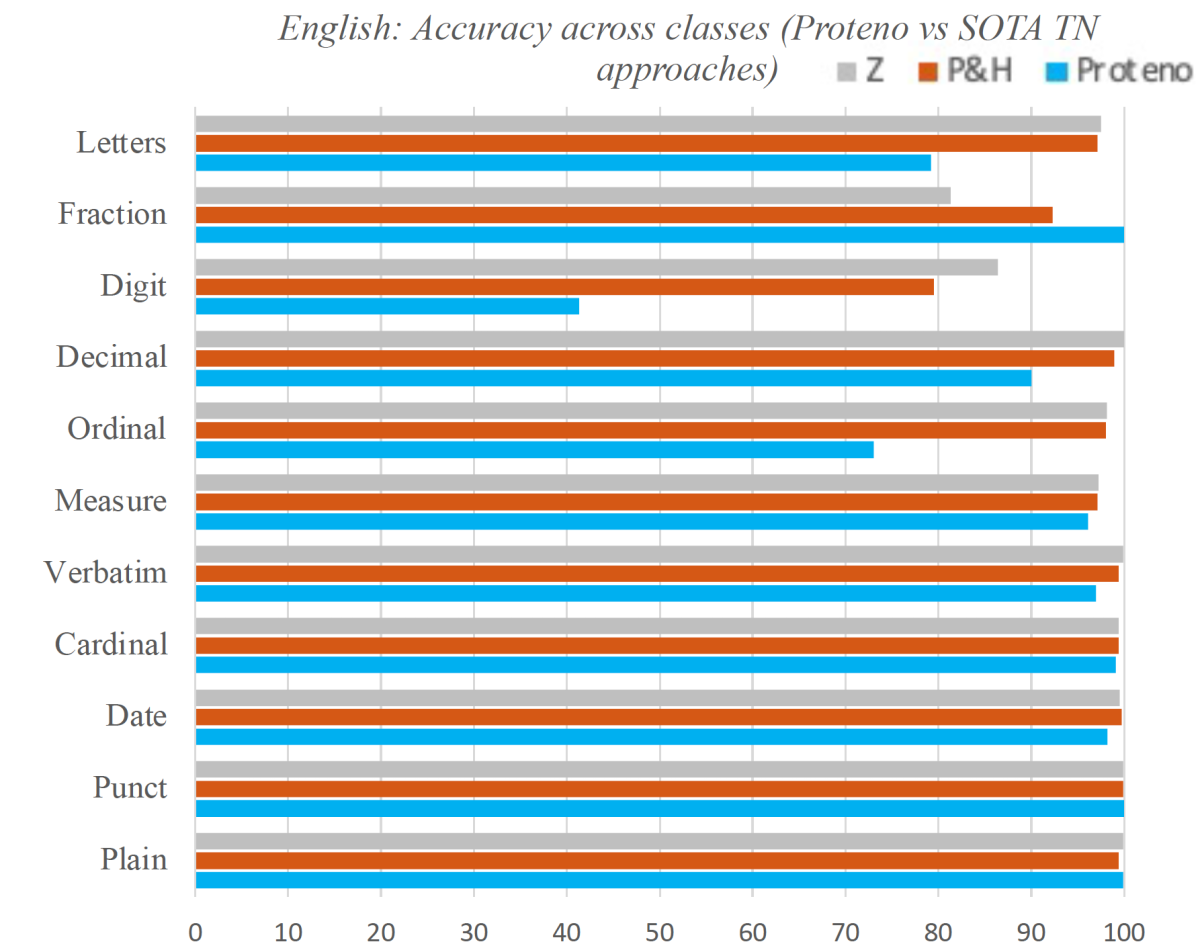
To benchmark Proteno’s performance in English, we could compare it to earlier models on only 11 of the 13 predefined classes found in existing datasets; differences in tokenization schemes meant that there were no logical mappings for the other two classes.
These results indicate that Proteno is a strong candidate for doing TN with low data annotation requirements while curbing unacceptable errors, which would make it a robust and scalable solution for production text-to-speech models.