Entity linking (EL) is the process of automatically linking entity mentions in text to the corresponding entries in a knowledge base (a database of facts that relate entities), such as Wikidata. For example, in the diagram below, we would aim to link the mention “England” to the entity “England Football Team” as opposed to the entity “England” the country.
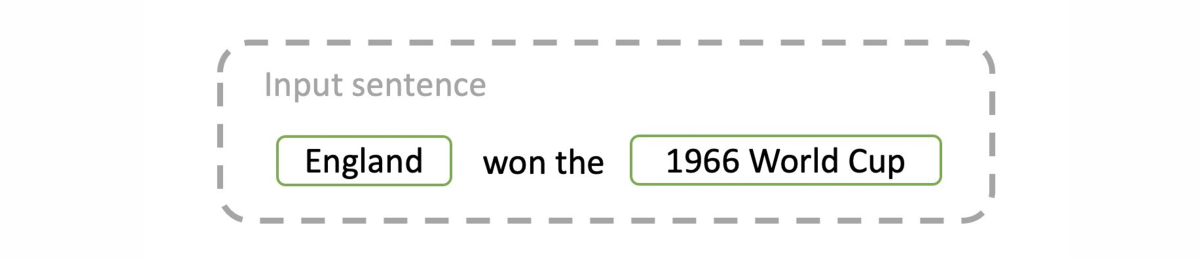
Entity linking is a common first step in natural-language-processing (NLP) applications such as question answering, information extraction, and natural-language understanding. It is critical for bridging unstructured text with knowledge bases, which enables access to vast amounts of curated data.
Current EL systems exhibit great performance on standard datasets, but they have several limitations when deployed in real-world applications. First, they are computationally intensive, which makes large-scale processing expensive.
Secondly, most EL systems are designed to link to specific knowledge bases (typically Wikipedia) and cannot be easily adapted to other knowledge bases. Lastly, the most efficient existing methods cannot link texts to entities that were introduced into the knowledge base after training (a task known as zero-shot EL), meaning they must be frequently retrained to be kept up-to-date.
In the NAACL 2022 industry track, we introduced a new EL system called ReFinED, which addresses all three issues. We built on this work in a second paper in the main conference, which introduces a novel method to incorporate additional knowledge base information into the model, further improving its accuracy.
ReFinED surpasses state-of-the-art performance on standard EL datasets by an average of 3.7 points in F1 score, a measure that factors in both false positives and false negatives, and it is 60 times faster than existing approaches with competitive performance.
ReFinED is capable of generalizing to large-scale knowledge bases such as Wikidata (which has 15 times as many entities as Wikipedia) and of zero-shot entity linking. The combination of speed, accuracy, and scale makes ReFinED an effective and cost-efficient system for extracting entities from web-scale datasets, for which the model has been successfully deployed within Amazon.
Entity linking with fine-grained types and descriptions
EL is challenging because entity mentions are often ambiguous. Therefore, EL systems must make effective use of context (surrounding words) to reliably disambiguate entity mentions.
Recent EL systems use deep-learning methods to match mentions, not with entities directly, but with information stored in the knowledge base, such as textual entity descriptions or fine-grained entity types. This is advantageous for linking to entities not seen in the training data (zero-shot EL), because the information used to describe them will have properties the model has seen during training.
However, such zero-shot-capable approaches are an order of magnitude more computationally expensive than non-zero-shot models, as they require numerous entity types and/or multiple forward passes through the model to encode mentions and descriptions. This makes large-scale processing prohibitively expensive for some applications.
Like earlier zero-shot-capable models, ReFinED uses fine-grained entity types and entity descriptions to perform EL. But we use a simple Transformer-based encoder that yields better performance than that of more complex architectures, surpassing the state of the art on five EL datasets.
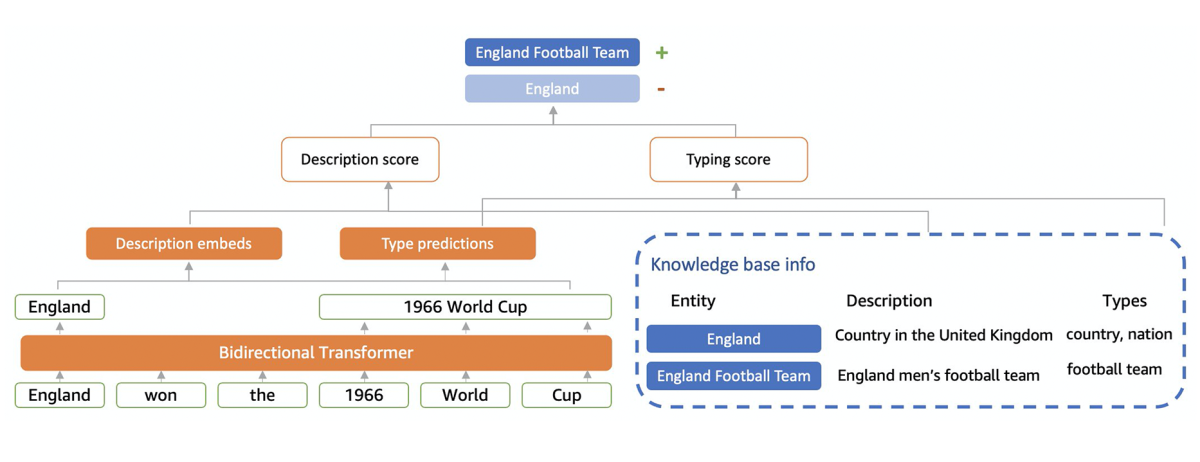
Unlike previous work, ReFinED performs mention detection (identifying entity mention spans), fine-grained entity typing (predicting entity types), and entity disambiguation (scoring entities) for all mentions within a document in a single forward pass, making it 60 times as fast as comparable models and therefore approximately 60 times more resource efficient to run.
Under the hood, ReFinED is a Transformer-based neural network that computes two scores, a description score and an entity typing score, to indicate how suitable an entity is for a mention.
Incorporating relationship data
One shortcoming of this approach is that there may be some mentions whose candidate entities cannot be disambiguated with knowledge base entity description and types. As illustration, consider the following sentence, with the entity descriptions and types for two entities that “Clinton” could refer to:
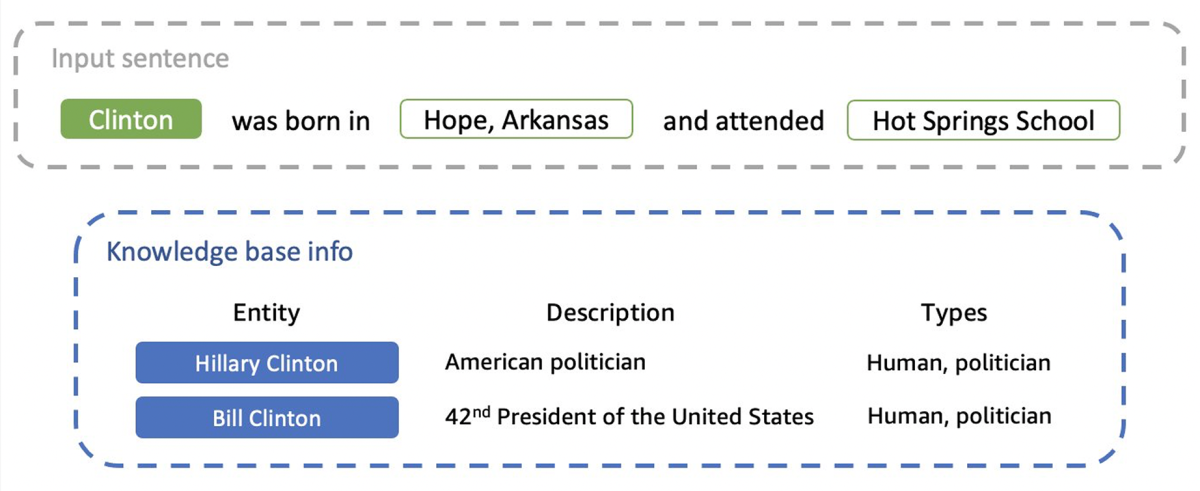
Given only the context of the sentence and the knowledge base descriptions and types, it is not possible to correctly decide whether the sentence is referring to Hillary Clinton or Bill Clinton.
Our second NAACL paper, “Improving entity disambiguation by reasoning over a knowledge base”, addresses this drawback. We propose an approach that uses additional knowledge base facts associated with the candidate entities.
Knowledge base facts encode the relations between pairs of entities, as in the following examples:
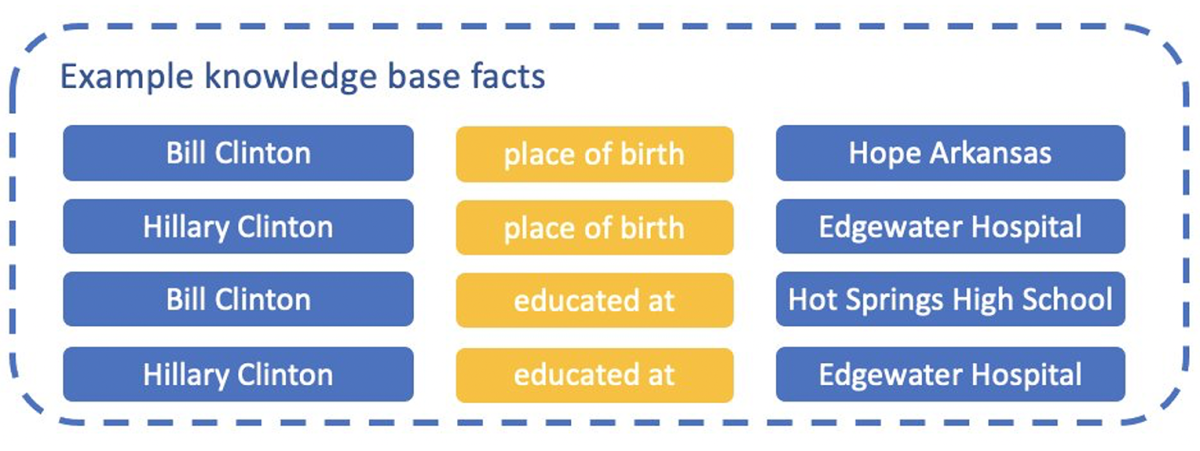
In order to use this type of information, we gave our model an additional mechanism, which allows it to predict the relationships connecting pairs of mentions in the text. For example, the model would infer from the sentence context that “Clinton”’s place of birth and place of education are “Hope, Arkansas” and “Hot Springs High School”. We can then match these inferences against facts in the knowledge base.
In this case, as the diagram below shows, we would find that the two predictions match the knowledge base facts for Bill Clinton but not for Hillary Clinton. As a result, our model would boost the score for Bill Clinton and, hopefully, make the correct prediction.
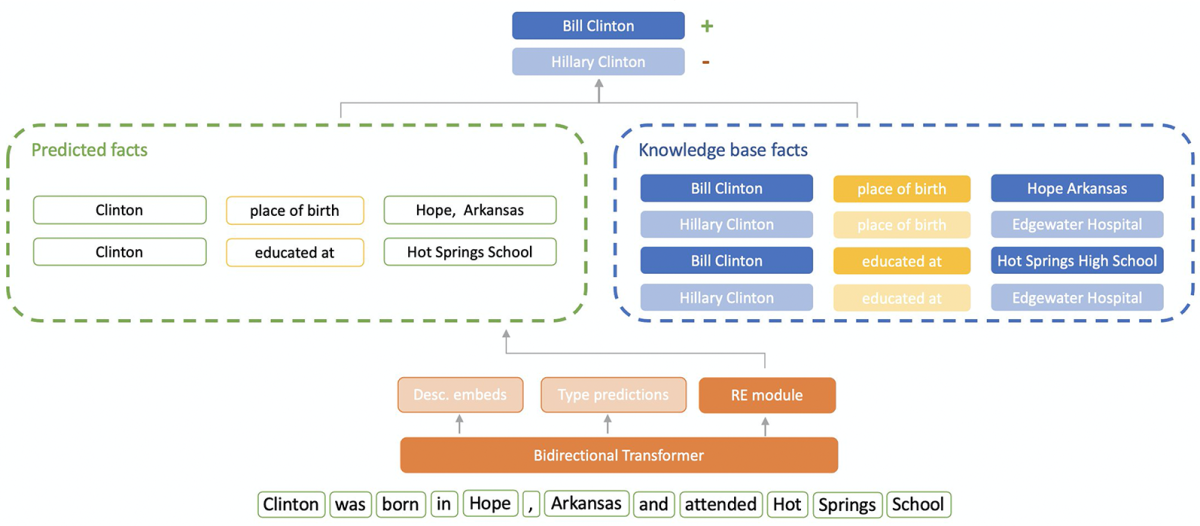
By adding this mechanism to the model, we were able to increase the state-of-the-art performance by 1.3 F1 points on average across six commonly used datasets in the literature and by 12.7 F1 points on the “ShadowLink” dataset, which focuses on particularly challenging examples.