On September 20, Amazon unveiled a host of new products and features, including Alexa Guard, a smart-home feature available on select Echo devices later this year. When activated, Alexa Guard can send a customer alerts if it detects the sound of glass breaking or of smoke or carbon monoxide alarms in the home.
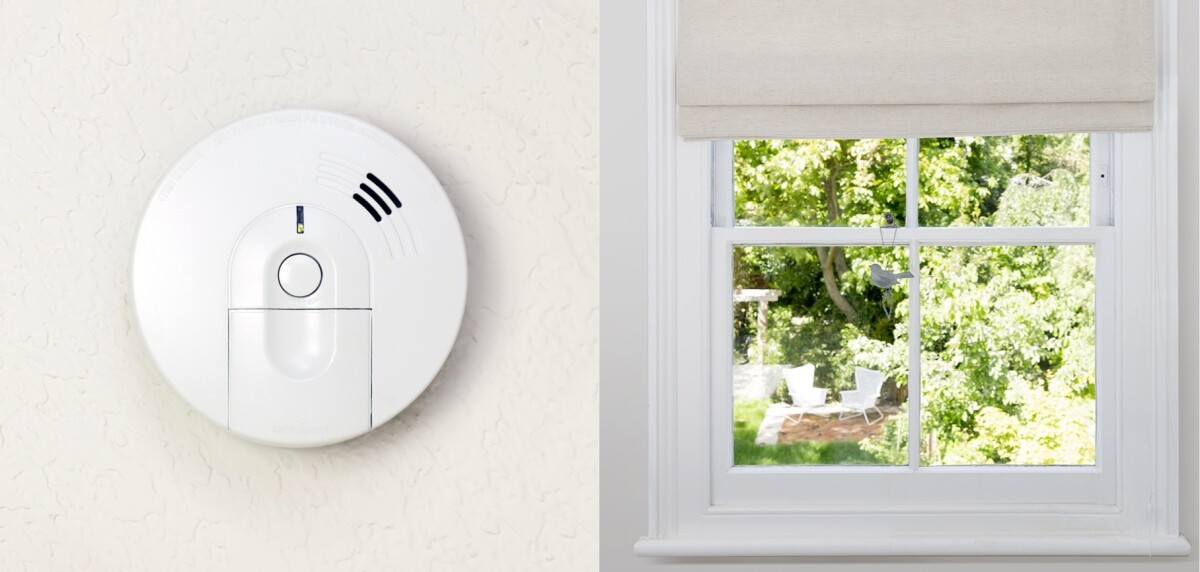
At this year’s Interspeech conference, in September, our team presented a pair of papers that describe two approaches we’ve taken to the problem of sound identification in our research. Both approaches use neural networks, but one of the networks — which we call R-CRNN — is larger and takes longer to train than the other.
We believe, however, that in the long run, it also promises greater accuracy. So the two systems might be used in conjunction: the smaller network would run locally on a sound detection device, uploading audio samples to the larger, cloud-based network only if they’re likely to indicate threats to home security.
We tested both systems using data that had been provided to contestants in the third annual IEEE AASP Challenge on Detection and Classification of Acoustic Scenes and Events (DCASE 2017). Both systems achieved higher scores than the third-place finisher in the competition, and we believe that they have advantages over the top two finishers, as well.
Systems entered in the competition had to analyze 30-second snippets of audio and determine, first, whether they contained particular sounds — such as glass breaking — and, second, where in the snippets the sounds occurred.
Most systems divided the snippets into 46-millisecond units — or “frames” — and then tried to assess whether individual frames contained the acoustic signatures of the target sounds.
When that task was complete, the systems had to stitch the frames back together into composite sounds. To do that, the top-performing systems used hand-coded rules that took advantage of the specific audio properties of the sounds in the contest dataset. Our systems don’t require this additional, content-specific step, so we believe they will generalize better to new sounds and settings.
Moreover, R-CRNN adapts a machine-learning mechanism that has already delivered state-of-the-art performance on several computer vision tasks. Computer vision research benefits from huge sets of labeled training data accumulated over decades; the data available to train our sound recognition systems was relatively meager. With more training data, R-CRNN’s performance should improve significantly.
The mechanism we adapt is called a region proposal network, which was developed to rapidly identify two-dimensional regions of images likely to contain objects sought by an object classifier. We instead use it to identify one-dimensional regions of an audio stream likely to contain sounds of interest.
This means that our classifier can act on the entire sound at once, rather than splitting it into frames that later must be pieced together again.
The region proposal network was designed to work with an object detector known as R-CNN, for region-based convolutional neural net. With R-CNN, images are fed to a convolutional neural net that learns to extract features useful for object detection, which then pass to the region proposal network.
In our network, R-CRNN, the extra “R” stands for “recurrent,” because our feature-extraction network is both convolutional and recurrent, meaning that it can factor in the order in which data arrive. That’s not necessary for image classification, but it usually improves audio processing, since it allows the network to learn systematic correlations between successive sounds.
Our feature extraction network is also a residual network, meaning that each of its layers receives not only the output of the layer beneath it but the input to the layer beneath that, too. That way, during training, each layer learns to elaborate on the computations performed by the preceding layers, rather than — at least occasionally — undoing them.
The feature summary vector produced by the extraction network passes to the region proposal network, and then both the summary vector and the region proposals pass to another network, which makes the final classification. In ongoing experiments, we’re evaluating whether this final classifier is necessary. If the region proposal network can draw reliable inferences itself, that will make the R-CRNN model both more compact and easier to train.
Like many of the contestants in the DCASE challenge, our other system splits input signals into 46-millisecond frames. And like them, it passes the frames through a network that learns to extract features of the signal useful for sound identification.
But our system also features an “attention mechanism,” a second network whose output is an array of values, one for each frame, in chronological order. Frames that appear to have characteristics of the target sound receive a high score in the output vector; frames that don’t receive a low score.
This array essentially demarcates the part of the input signal that contains the sound of interest, again dispensing with the need to stitch frames back together after the fact. Both the array and the feature vector pass to a classifier that makes the final assessment.
This simple architecture significantly reduces the model’s memory footprint and computational overhead, relative not only to R-CRNN but to the top two finishers in the DCASE challenge, too. (Those systems used “ensemble methods”, meaning they comprised multiple, separately trained models, which process data independently before having their results pooled.) It thus holds unusual promise for on-device use.
The model also has one other architectural feature that makes it more accurate. As the input signal passes through the feature extraction network, its time resolution is halved several times: we keep reducing the number of network nodes required to represent the signal. This ensures that the network’s output — the feature vector — will include information relevant to the final classification regardless of the sound’s duration. The feature vector for a half-second’s worth of breaking glass, for instance, will look roughly the same as the feature vector for three seconds.
In fact, this network fared slightly better than R-CRNN on the DCASE challenge test set. On the task of identifying whether a given 30-second input contained a sound of interest, it had an error rate of 20% and an F1 score (which measures both false positives and false negatives) of 90%; R-CRNN’s scores were 23% and 88%, respectively. (For comparison, the winner had scores of 13% and 93%, and the third-place finisher had scores of 28% and 85%.) But again, we believe that R-CRNN’s performance suffered more from lack of training data than the other models’. We consider it the most direct way to approach the problem of sound recognition.
Acknowledgments: Ming Sun, Chao Wang