Amazon Alexa currently has more than 40,000 third-party skills, which customers use to get information, perform tasks, play games, and more. To make it easier for customers to find and engage with skills, we are moving toward skill invocation that doesn’t require mentioning a skill by name (as highlighted in a recent post).
To enable name-free skill interaction, Alexa currently uses a two-step, scalable, and efficient neural shortlisting-reranking approach. (I described our approach to shortlisting in a post yesterday). The shortlisting step uses a scalable neural model to efficiently find the optimal (k-best) candidate skills for handling a particular utterance; the re-ranking step uses rich contextual signals to find the most relevant of those skills. We use the term “re-ranking” since we improve upon the initial confidence score provided by the shortlisting step.
This week, at the Human Language Technologies conference of the North American chapter of the Association for Computational Linguistics (NAACL 2018), my colleagues and I presented a paper, “A Scalable Neural Shortlisting-Reranking Approach for Large-Scale Domain Classification in Natural Language Understanding,” that describes our approach.
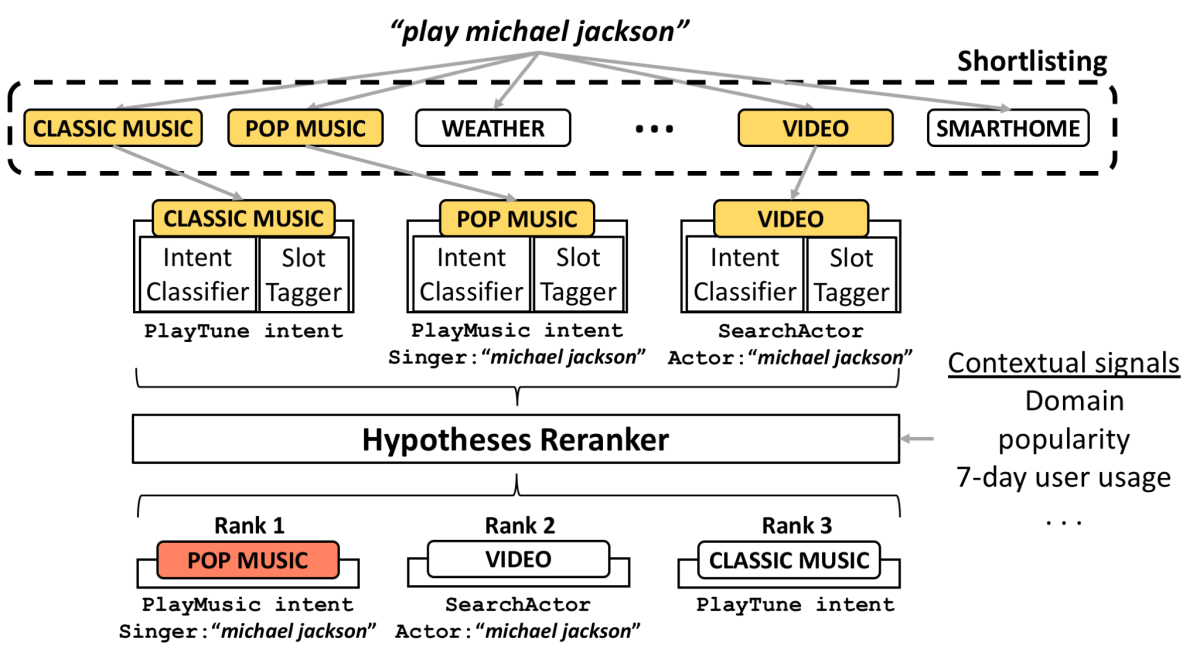
The Challenge
The problem here is essentially a domain classification problem over the k-best candidate skills returned by the shortlisting system, which we call Shortlister. The goal of Shortlister is to achieve high recall — to identify as many pertinent skills as possible — with maximum efficiency. On the other hand, the goal of the reranking network, HypRank, is to use rich contextual signals to achieve high precision — to select the most pertinent skills. Designing HypRank comes with its own challenges:
- Hypothesis representation: It needs to use available contextual signals to produce an effective hypothesis representation for each skill in the k-best list;
- Cross-hypothesis feature representation: It needs to efficiently and automatically compare features, such as a skill’s intent confidence, to those of other candidate skills in the k-best list;
- Generalization: It needs to be language-agnostic; and
- Robustness: It needs to be able to accommodate changes, such as independent modifications to Shortlister or to the natural-language-understanding models that provide skill-specific semantic interpretation of utterances.
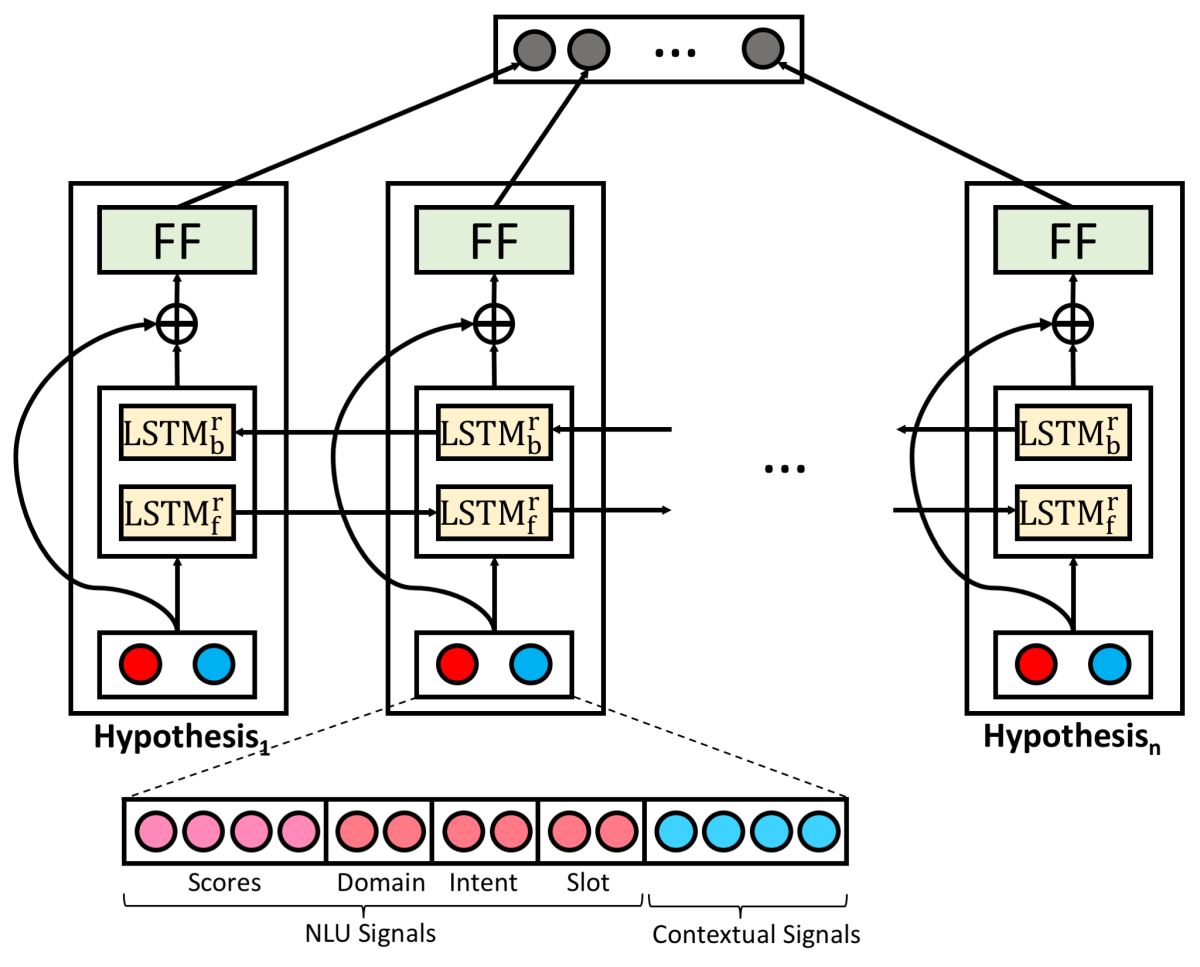
The HypRank Neural Model
HypRank comprises two components:
1. Hypothesis representation for each skill; and 2. A bidirectional long short-term memory (bi-LSTM) model for re-ranking a list of hypotheses.
For each skill in the k-best list, we form a hypothesis based on additional semantic and contextual signals. For example, we perform intent-slot semantic analysis for a skill. If a user says “play Michael Jackson,” the Pop Music skill might infer the intent PlayMusic, while the Classic Music skill might infer the intent PlayTune. But the confidence scores that the skills assign their inferences could be useful for skill re-ranking. The hypothesis generator is constantly being updated, re-weighting signals and accommodating new functionality and changes in usage patterns.
HypRank is unique because of its list-wise ranking approach using a bi-LSTM layer. LSTM models are common in natural-language processing because they factor in the order in which data are received: if you’re trying to understand the sixth word in an utterance, it helps to know what the previous five were. Bidirectional LSTM models consider data sequences both forward and backward.
By leveraging the bi-LSTM layer, HypRank can evaluate an entire list of skill hypotheses before providing a re-ranking score for each hypothesis. This is distinct from point-wise approaches that look at each hypothesis in isolation or pair-wise approaches that look at pairs of hypotheses in a series of tournament-like competitions.
Whereas past re-ranking approaches relied on manually crafted cross-hypothesis features, our approach uses the bi-LSTM layer to automatically learn and encode appropriate cross-hypothesis features for improved re-ranking. The encoded cross-hypothesis vector then passes through a conventional feed-forward network, which determines the final score for each hypothesis.
HypRank is agnostic about both language and locale. The contextual information used to form a hypothesis is designed to be independent of whether the language spoken is English or French and whether the locale is the U.S. or France. Research is ongoing on making HypRank as generalizable as possible and also as robust as possible to changes in the upstream signals.
Acknowledgments: Sunghyun Park, Ameen Patel, Jihwan Lee, Joo-Kyung Kim, Dongchan Kim, Hammil Kerry, Ruhi Sarikaya, and all engineers in the Fan Sun, Bo Cao, and Yan Weng teams.