Emine Yilmaz, an Amazon Scholar and a professor of computer science at University College London, has for years been intimately involved with the European Conference on Information Retrieval (ECIR), which starts next week. She was the conference’s Program Committee cochair last year and Doctoral Consortium cochair in 2017, and this year, she’s on the committee selecting the winner of the conference’s test-of-time award.
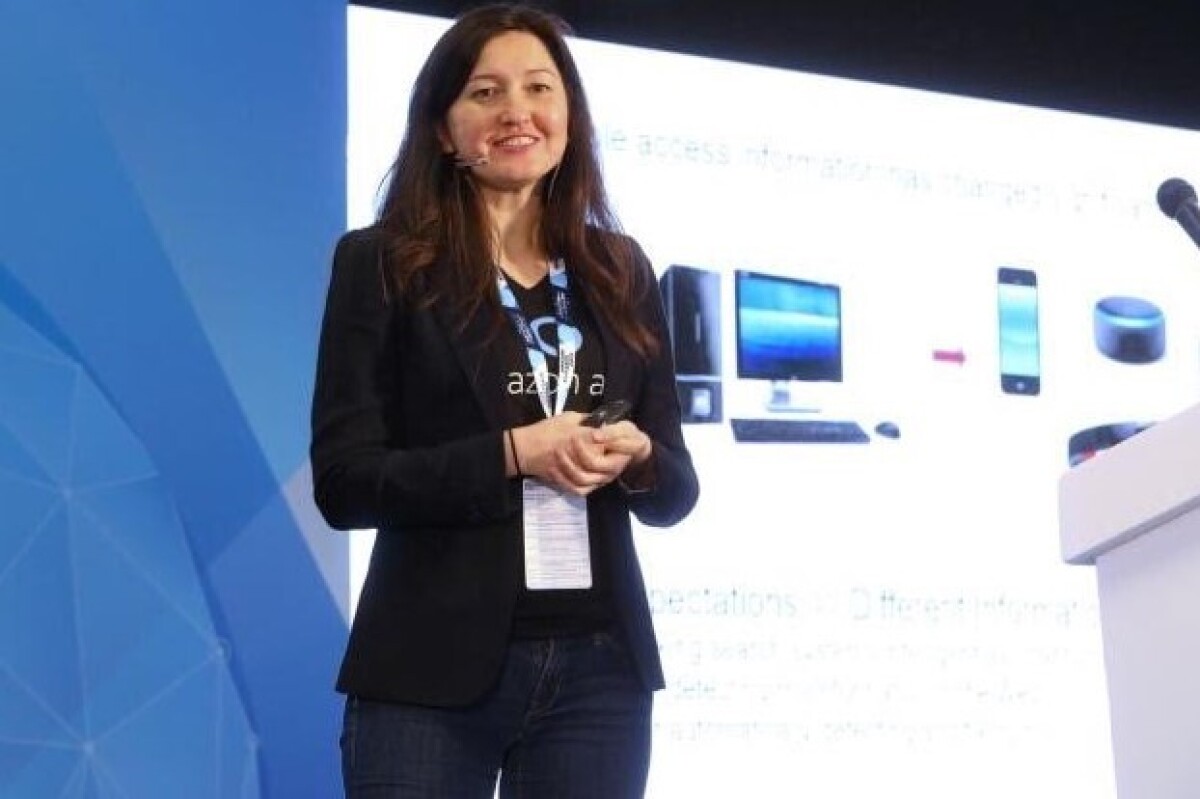
Within the ECIR community, she says, she’s recently noticed a growing interest in conversational information retrieval (IR), or using multiturn dialogues to refine queries.
“Conversational IR is an area that's kind of slowly emerging,” Yilmaz says. “How do you build an interactive system that works together with the user? And how do you make such systems potentially proactive? I think that's now gaining more and more and more importance.”
At Amazon, Yilmaz is working with the Alexa Shopping team, where conversational IR is a central research topic. A conventional, web-based search engine will typically return a list of results, and the customer simply selects the one or two of greatest interest. But few voice service customers want to sit through a recitation of 10 or 20 results, so the ability to interactively refine queries is crucial.
Read more about Amazon's involvement in ECIR, including some of the papers our scientists will be presenting there.
In the near term, Yilmaz explains, “the main focus is to predict user satisfaction. We look at the user interactions with Alexa, and we look at how the behavior evolves. Based on that, we try to detect or predict if the user interaction was satisfactory or not.”
One reason to try to predict user satisfaction is that voice interactions generate less data than web-based interactions. Someone who clicks two links among the 20 returned by a conventional search engine conveys information about not only those two links but also the remaining 18. If, on the other hand, a voice-based query returns a single result, the customer’s decision about whether or not to engage with that result is not nearly as informative. Predicting customers’ satisfaction with query results they weren’t exposed to helps fill in the gaps.
Explore, exploit, evaluate
But predicting customer satisfaction has other uses, Yilmaz explains. “Let's say you’re beta-testing a new feature, and you have to decide whether to show it to users or not,” she says. “There is a dilemma there. You don't want to show it to many users, because maybe it's a bad feature, and you don't want to affect user satisfaction and user experience. On the other hand, you need to show it to enough users to get a reliable indication of its quality. So you should show it to a targeted, small set of users and ideally identify if users would be satisfied with it by using very limited datasets.”
Predicting customer satisfaction can also help in the evaluation of conversational IR systems, Yilmaz explains. “As a user, you don't think about the importance of evaluation of such systems,” she says. “But at the end of the day, if your goal is to build a better conversational IR system, you need to be able to quantify what a better system means. At the moment, there is no good metric that is highly correlated with user satisfaction and is designed specifically for conversational IR that people can optimize for.”
And of course, the goal is to build a better conversational IR system.
“I think that's where the field is going,” Yilmaz says. “The system does whatever it can, given its understanding of what the user needs, and whenever it’s uncertain, it asks questions.” In the field of information retrieval, Yilmaz says, “there is a lot of work on systems that can ask clarification questions in order to improve the support they can provide. And also systems that can provide explanations together with the response. The model may say, ‘I'm recommending this restaurant because I think you like Sichuan cuisine.’ The user may say, ‘Well, now I'm not in the mood for Sichuan. I feel like pasta’. During the last few years, a lot of research has been devoted to building such systems, but we are still at the very beginning phases.”