As usual at the International Conference on Acoustics, Speech, and Signal Processing (ICASSP), a plurality of Amazon’s accepted papers concentrate on automatic speech recognition — with, this year, a particular emphasis on personalized speech recognition. The topics of acoustic-event detection, keyword spotting, and signal processing are also well represented.
But as is also usual, some of the Amazon papers wander farther afield, to topics like commonsense reasoning, self-learning, query rewriting, and general machine learning techniques. Below is a quick guide to Amazon’s more than 40 papers at the conference.
Acoustic-event classification
FedRPO: Federated relaxed Pareto optimization for acoustic event classification
Meng Feng, Chieh-Chi Kao, Qingming Tang, Amit Solomon, Viktor Rozgic, Chao Wang
Multiscale audio spectrogram transformer for efficient audio classification
Wentao Zhu, Mohamed Omar
Transformer-based bioacoustic sound event detection on few-shot learning tasks
Liwen You, Erika Pelaez Coyotl, Suren Gunturu, Maarten Van Segbroeck
Weight-sharing supernet for searching specialized acoustic event classification networks across device constraints
Guan-Ting Lin, Qingming Tang, Chieh-Chi Kao, Viktor Rozgic, Chao Wang
Automatic speech recognition
Cross-utterance ASR rescoring with graph-based label propagation
Srinath Tankasala, Long Chen, Andreas Stolcke, Anirudh Raju, Shally Deng, Chander Chandak, Aparna Khare, Roland Maas, Venkatesh Ravichandran
Dynamic chunk convolution for unified streaming and non-streaming Conformer ASR
Xilai Li, Goeric Huybrechts, Srikanth Ronanki, Jeff Farris, Sravan Bodapati
Domain adaptation with external off-policy acoustic catalogs for scalable contextual end-to-end automated speech recognition
David M. Chan, Shalini Ghosh, Ariya Rastrow, Björn Hoffmeister
Gated contextual adapters for selective contextual biasing in neural transducers
Anastasios Alexandridis, Kanthashree Mysore Sathyendra, Grant Strimel, Feng-Ju (Claire) Chang, Ariya Rastrow, Nathan Susanj, Athanasios Mouchtaris
Mask the bias: Improving domain-adaptive generalization of CTC-based ASR with internal language model estimation
Nilaksh Das, Monica Sunkara, Sravan Bodapati, Jason Cai, Devang Kulshreshtha, Jeff Farris, Katrin Kirchhoff
On-the-fly text retrieval for end-to-end ASR adaptation
Bolaji Yusuf, Aditya Gourav, Ankur Gandhe, Ivan Bulyko
Robust acoustic and semantic contextual biasing in neural transducers for speech recognition
Xuandi Fu, Kanthashree Mysore Sathyendra, Ankur Gandhe, Jing Liu, Grant Strimel, Ross McGowan, Athanasios Mouchtaris
Code generation
Conversational text-to-SQL: An odyssey into state-of-the-art and challenges ahead
Sree Hari Krishnan Parthasarathi, Lu Zeng, Dilek Hakkani-Tür
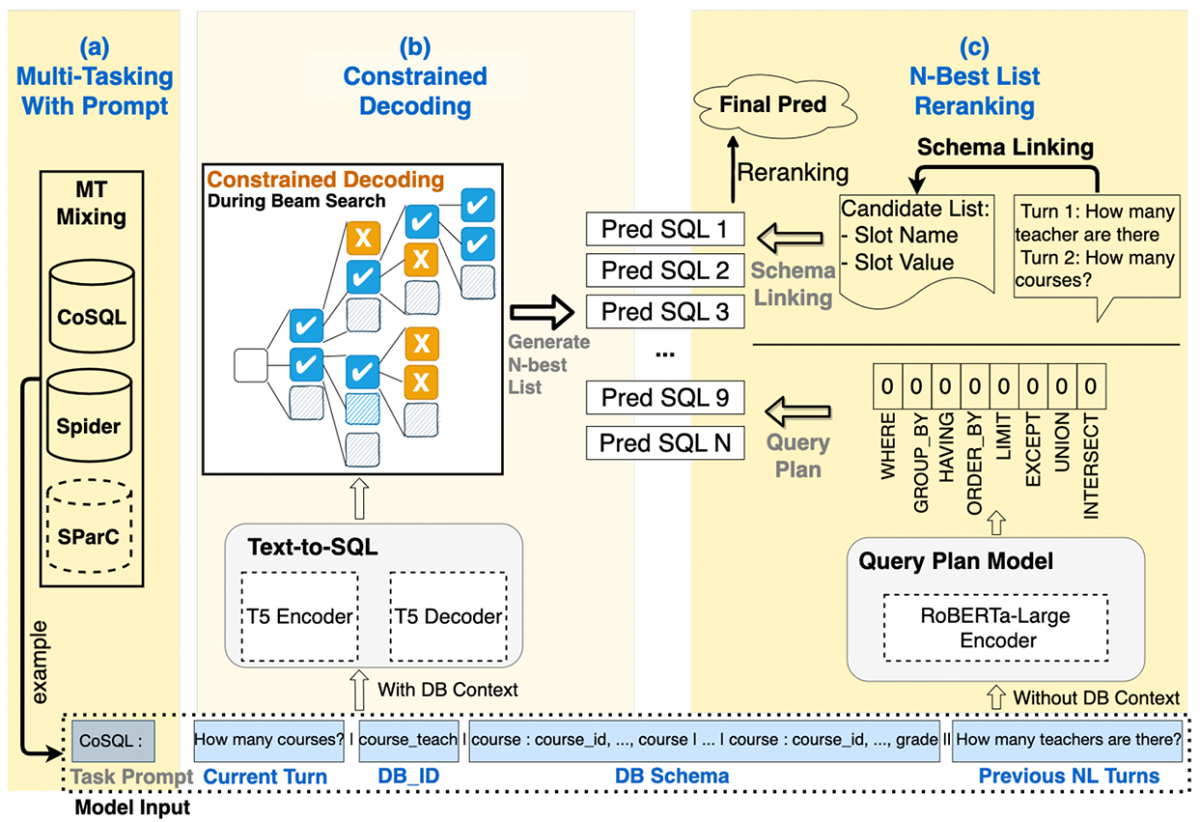
Commonsense reasoning
CLICKER: Attention-based cross-lingual commonsense knowledge transfer
Ruolin Su, Zhongkai Sun, Sixing Lu, Chengyuan Ma, Chenlei Guo
Continual learning
Quantifying catastrophic forgetting in continual federated learning
Christophe Dupuy, Jimit Majmudar, Jixuan Wang, Tanya Roosta, Rahul Gupta, Clement Chung, Jie Ding, Salman Avestimehr
Endpoint detection
Adaptive endpointing with deep contextual multi-armed bandits
Do June Min, Andreas Stolcke, Anirudh Raju, Colin Vaz, Di He, Venkatesh Ravichandran, Viet Anh Trinh
Towards accurate and real-time end-of-speech estimation
Yifeng Fan, Colin Vaz, Di He, Jahn Heymann, Viet Anh Trinh, Zhe Zhang, Venkatesh Ravichandran
Keyword spotting
Dual-attention neural transducers for efficient wake word spotting in speech recognition
Saumya Sahai, Jing Liu, Thejaswi Muniyappa, Kanthashree Mysore Sathyendra, Anastasios Alexandridis, Grant Strimel, Ross McGowan, Ariya Rastrow, Feng-Ju Chang, Athanasios Mouchtaris, Siegfried Kunzmann
Fixed-point quantization aware training for on-device keyword-spotting
Sashank Macha, Om Oza, Alex Escott, Francesco Caliva, Robbie Armitano, Santosh Kumar Cheekatmalla, Sree Hari Krishnan Parthasarathi, Yuzong Liu
Self-supervised speech representation learning for keyword-spotting with light-weight transformers
Chenyang Gao, Yue Gu, Francesco Caliva, Yuzong Liu
Small-footprint slimmable networks for keyword spotting
Zuhaib Akhtar, Mohammad Omar Khursheed, Dongsu Du, Yuzong Liu
Language learning
Phonetic RNN-transducer for mispronunciation diagnosis
Daniel Zhang, Soumya Saha, Sarah Campbell
Machine learning
Prune then distill: Dataset distillation with importance sampling
Anirudh Sundar, Gokce Keskin, Chander Chandak, I-Fan Chen, Pegah Ghahremani, Shalini Ghosh
Role of bias terms in dot-product attention
Mahdi Namazifar, Devamanyu Hazarika, Dilek Hakkani-Tür
Natural-language understanding
Distill-quantize-tune: Leveraging large teachers for low-footprint efficient multilingual NLU on edge
Pegah Kharazmi, Zhewei Zhao, Clement Chung, Samridhi Choudhary
Pyramid dynamic inference: Encouraging faster inference via early exit boosting
Ershad Banijamali, Pegah Kharazmi, Sepehr Eghbali, Jixuan Wang, Clement Chung, Samridhi Choudhary
Personalized speech recognition
Dialog act guided contextual adapter for personalized speech recognition
Feng-Ju (Claire) Chang, Thejaswi Muniyappa, Kanthashree Mysore Sathyendra, Kai Wei, Grant Strimel, Ross McGowan
PROCTER: Pronunciation-aware contextual adapter for personalized speech recognition in neural transducers
Rahul Pandey, Roger Ren, Qi Luo, Jing Liu, Ariya Rastrow, Ankur Gandhe, Denis Filimonov, Grant Strimel, Andreas Stolcke, Ivan Bulyko
Slot-triggered contextual biasing for personalized speech recognition using neural transducers
Sibo Tong, Philip Harding, Simon Wiesler
Query rewriting
KG-ECO: Knowledge graph enhanced entity correction for query rewriting
Jason Cai, Mingda Li, Ziyan Jiang, Eunah Cho, Zheng Chen, Yang Liu, Xing Fan, Chenlei Guo
Self-learning
Federated self-learning with weak supervision for speech recognition
Milind Rao, Gopinath Chennupati, Gautam Tiwari, Anit Kumar Sahu, Anirudh Raju, Ariya Rastrow, Jasha Droppo
Self-healing through error detection, attribution, and retraining
Ansel MacLaughlin, Anna Rumshisky, Rinat Khaziev, Anil Ramakrishna, Yuval Merhav, Rahul Gupta
Signal processing
A framework for unified real-time personalized and non-personalized speech enhancement
Zhepei Wang, Ritwik Giri, Devansh Shah, Jean-Marc Valin, Michael M. Goodwin, Paris Smaragdis
Augmentation robust self-supervised learning for human activity recognition
Cong Xu, Yuhang Li, Dae Lee, Andrew Park, Hongda Mao, Huyen Do, Jonathan Chung, Dinesh Nair
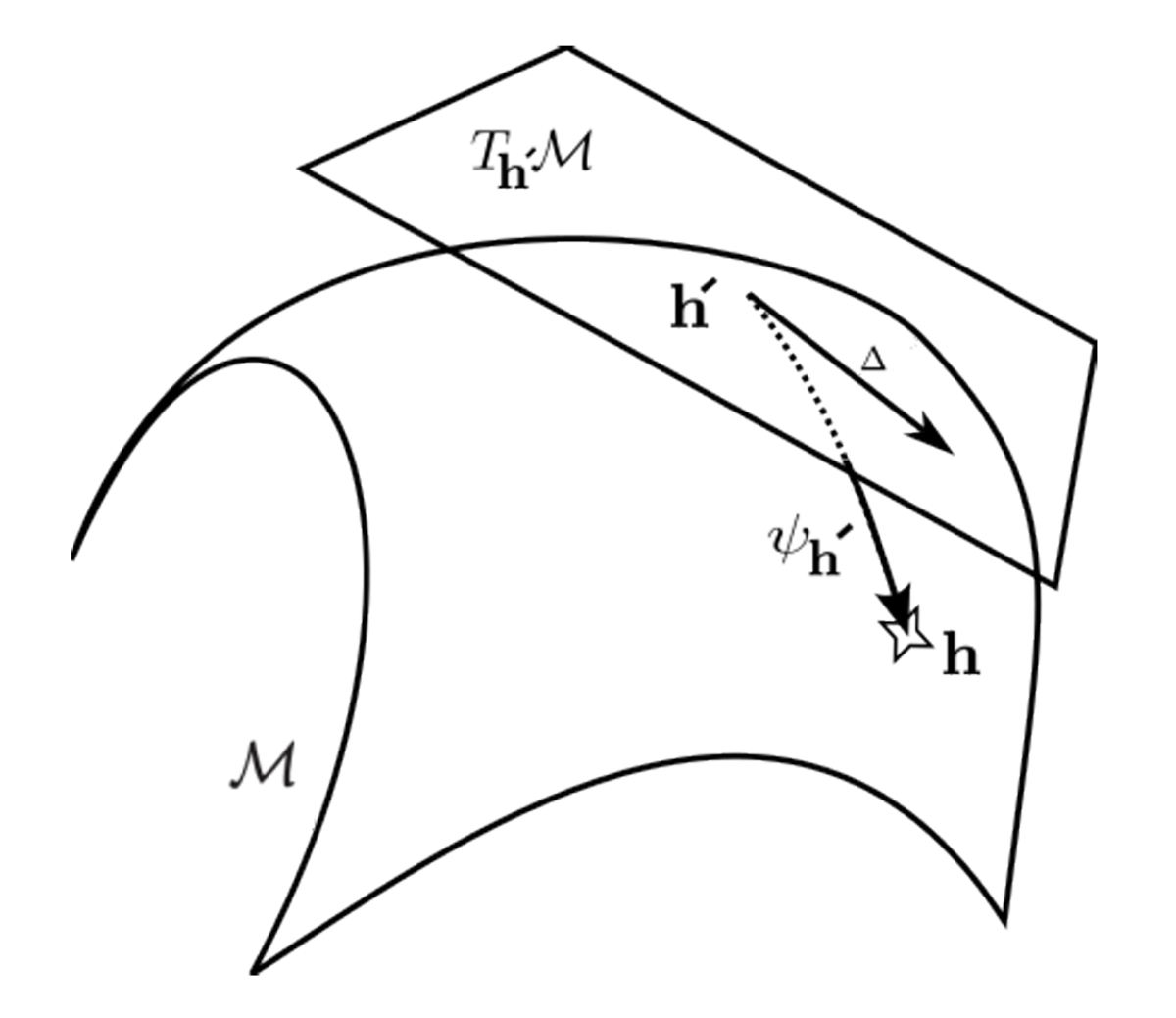
Generative modeling based manifold learning for adaptive filtering guidance
Karim Helwani, Paris Smaragdis, Michael M. Goodwin
SPADE: Self-supervised pretraining for acoustic disentanglement
John Harvill, Jarred Barber, Arun Nair, Ramin Pishehvar
Spoken-language understanding
End-to-end spoken language understanding using joint CTC loss and self-supervised, pretrained acoustic encoders
Jixuan Wang, Martin Radfar, Kai Wei, Clement Chung
Exploring subgroup performance in end-to-end speech models
Alkis Koudounas, Eliana Pastor, Giuseppe Attanasio, Vittorio Mazzia, Manuel Giollo, Thomas Gueudre, Luca Cagliero, Luca de Alfaro, Elena Baralis, Daniele Amberti
Multilingual end-to-end spoken language understanding for ultra-low footprint applications
Markus Mueller, Anastasios Alexandridis, Zach Trozenski, Joel Whiteman, Grant Strimel, Nathan Susanj, Athanasios Mouchtaris, Siegfried Kunzmann
Text-to-speech
Framewise WaveGAN: High speed adversarial vocoder in time domain with very low computational complexity
Ahmed Mustafa, Jean-Marc Valin, Jan Buethe, Paris Smaragdis, Mike Goodwin
Modelling low-resource accents without accent-specific TTS frontend
Georgi Tinchev, Marta Czarnowska, Kamil Deja, Kayoko Yanagisawa, Marius Cotescu
Video
ModEFormer: Modality-preserving embedding for audio-video synchronization using transformers
Akash Gupta, Rohun Tripathi, Wondong Jang
Multi-scale compositional constraints for representation learning on videos
Georgios Paraskevopoulos, Chandrashekhar Lavania, Lovish Chum, Shiva Sundaram
Voice communication
Low-bitrate redundancy coding of speech using a rate-distortion-optimized variational autoencoder
Jean-Marc Valin, Jan Buethe, Ahmed Mustafa