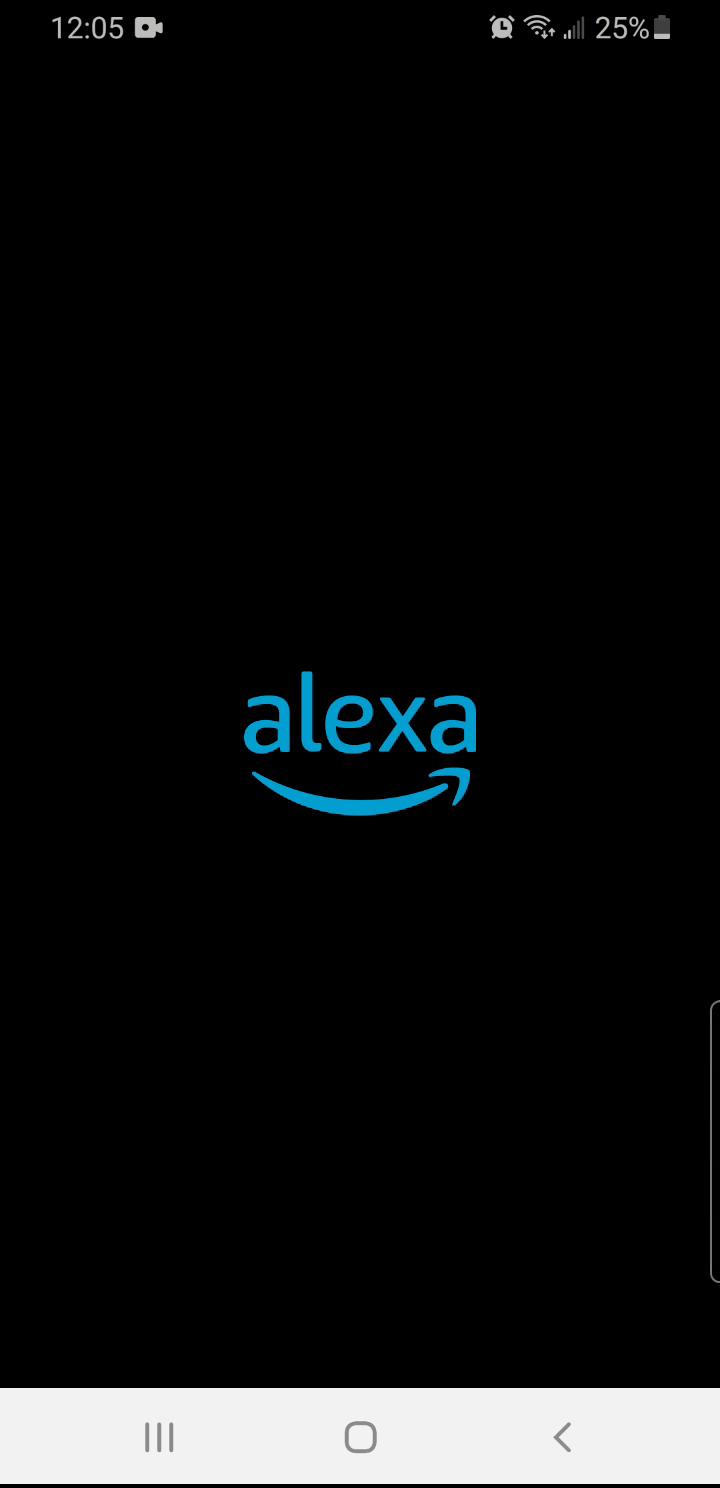
Last fall, Amazon introduced ultrasound-based motion detection, to enable Alexa customers to initiate Routines, or prespecified sequences of actions, when certain types of motion are detected (or not detected). For instance, Routines could be configured to automatically turn the lights on, play music, or announce weather or traffic when motion is detected near a customer’s Echo device, indicating that someone has entered the room.
There are many different motion detection technologies, but we selected ultrasound because it works in low-light conditions or even in the dark and, unlike radio waves, ultrasound waves do not travel through drywall, so there's less risk of detecting motion in other rooms.
Getting the technology to work on existing Echo hardware required innovation on a number of fronts — among other things, reducing false alarms by adequately sampling long-tail data; devising a self-calibration feature to adjust to variations in commodity hardware; and filtering out distortion during concurrent ultrasound detection and music playback. We describe the details below.
Ultrasound-based presence detection
With ultrasound-based presence detection (USPD), an ultrasonic signal (>=32 kHz) is transmitted via onboard loudspeakers, and changes in the signal received at the microphones are monitored to detect motion.
Ultrasound sensors can be broadly categorized as using Doppler sensing or time-of-flight sensing. In Doppler sensing, once the signal is transmitted, the system detects motion by looking for frequency shifts in the recorded spectrum of the signal, which are caused by its reflection from moving objects. This frequency shift is similar to the shift in sound frequencies you hear in a police car siren it is approaching you or moving away from you.
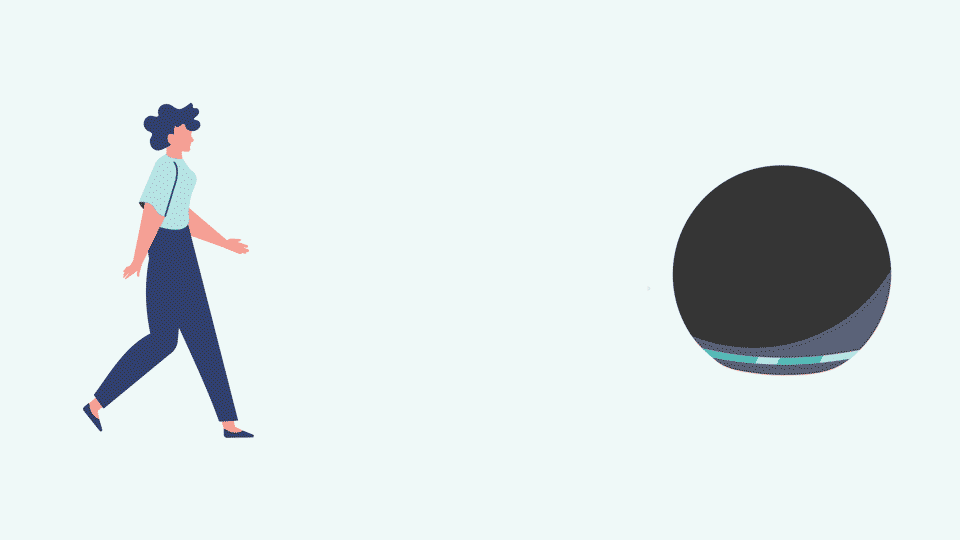
In time-of-flight sensing, variations in the arrival time of the reflected signal are monitored to detect changes in the environment. We use Doppler sensing due to the robustness of its motion detection signal and because it generalizes well across the cases when Alexa is or is not playing audio simultaneously.
The magnitude of the Doppler-shifted signal depends on factors such as distance from target to source, the size and absorption coefficient of the target, the absorption coefficient of the room, and even the humidity and temperature in the room. In addition, when a person moves through a closed space, not only do we observe multiple Doppler components due to various parts of the body moving in different directions with different speeds, but we also observe repetitions of those components due to reflections.
Because of all these complexities, the signal received at the source is not at all as clean as a single tone with a frequency shift. In practice, what we observe looks more like this:
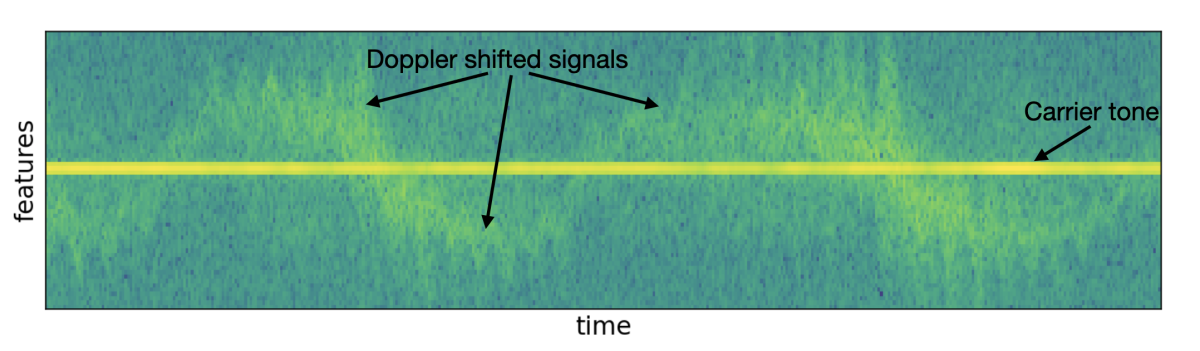
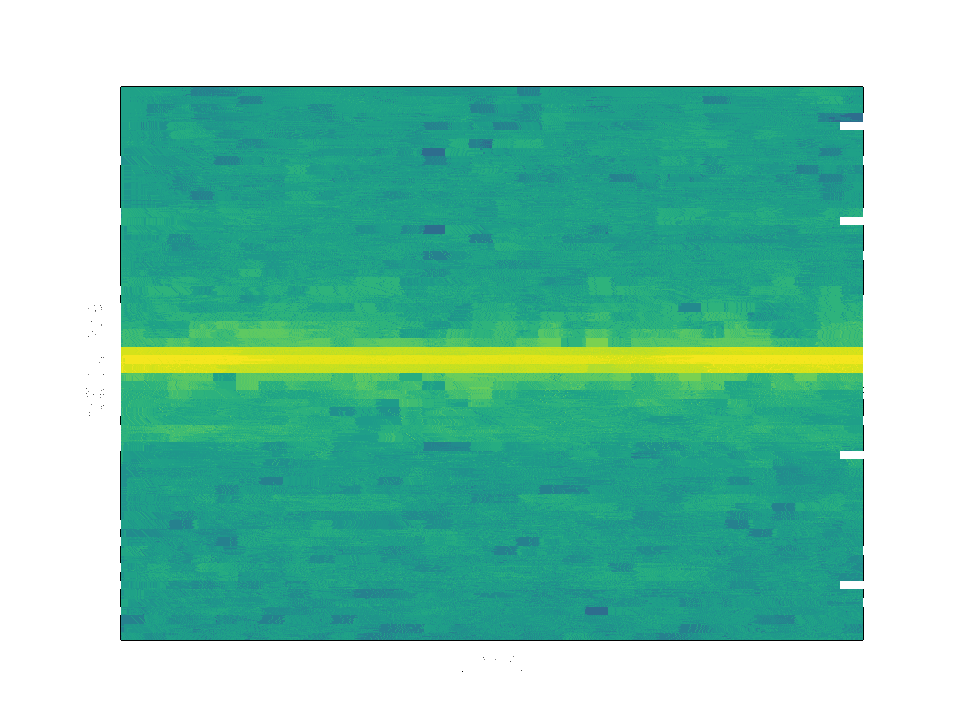
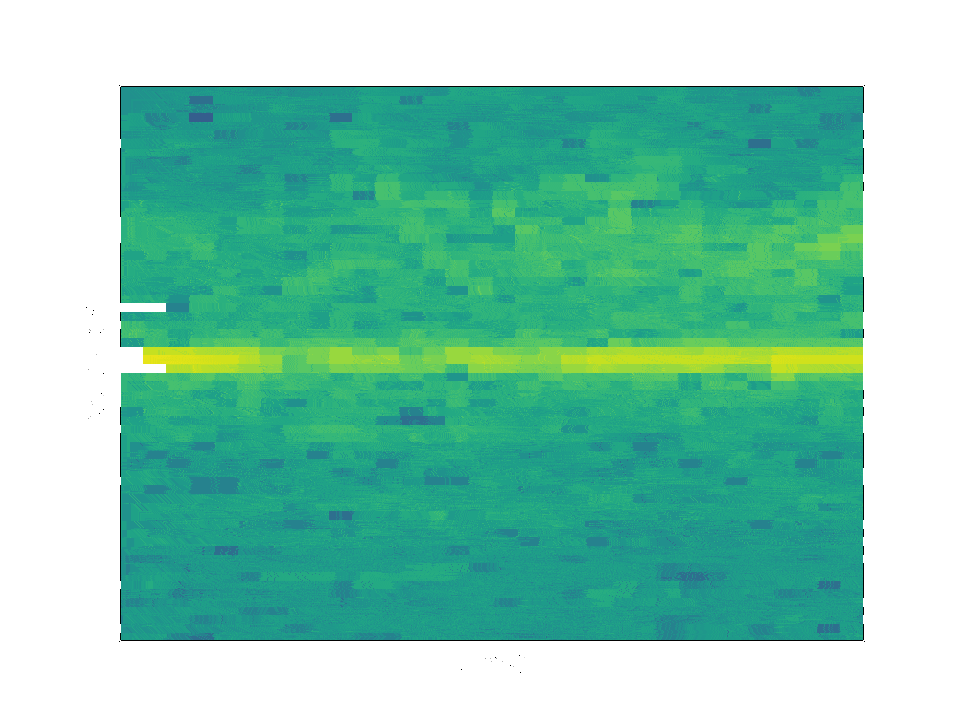
Further, moving objects such as fans and curtains introduce their own Doppler shifts, which have to be rejected since they do not necessarily indicate people’s presence. Below are two spectrograms, one of a room with no motion other than a rotating floor fan and another with both a fan and human motion near a device. As can be seen, they are difficult to tell apart.
These complications mean that conventional signal processing is insufficient to recognize human motion from Doppler-shifted signals. So we instead use deep learning, which should be able to recognize more heterogeneous patterns in the signal.
Below is a high-level block diagram of our USPD algorithm. On the signal transmitter side, a device- and environment-dependent optimal ultrasound signal is transmitted through the onboard loudspeaker. This signal gets reflected from a moving object and is then captured by the onboard microphone array. The signal is preprocessed and then passed to a neural-network-based classifier to detect motion.
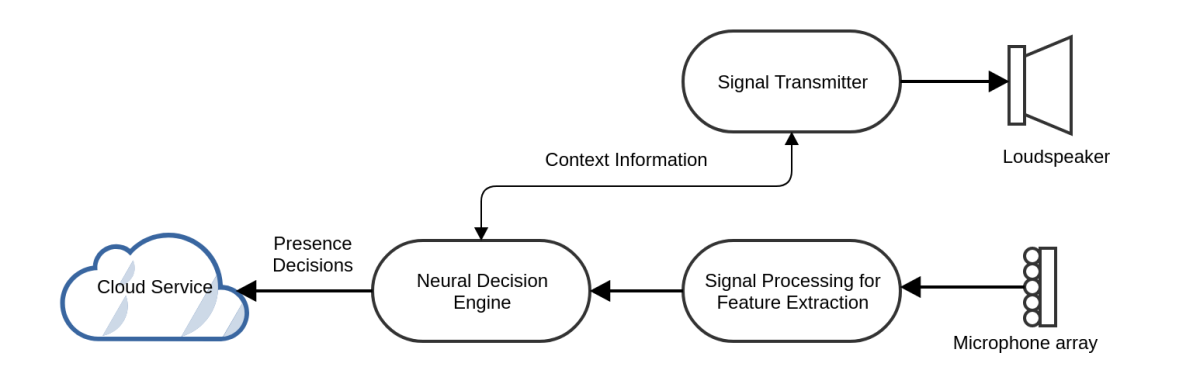
False alarms
The biggest algorithmic challenge we faced was achieving high detection accuracy while keeping the false-alarm rate low. Reducing false-alarm rates is especially challenging because of the well-known long-tail problem in AI: there are a multitude of rare events that could fool a detector, but their rarity means that they’re usually underrepresented in training data.
To address this problem, we started by training a seed model on a relatively small amount of data. First, we used the seed model to sort through large amounts of data and extract infrequent events. Second, we used a model trained on that rare-event data to automatically capture infrequent events during our internal data collection process. Data captured by these methods eventually helped us address the long-tail problem and achieve extremely low false-alarm rates.
Deployment challenges
Deploying the trained model brought its own challenges. We wanted to enable USPD with the lowest possible emission level, while still retaining a sufficient detection range, and do all of this with no additional hardware costs (i.e., using the available microphones and loudspeakers on Echo devices instead of dedicated ultrasound transmitters). Further, we decided to support always-on motion detection. This meant being able to detect motion even when a user is playing music from the device speakers. Finally, we added algorithms to improve the user experience in the presence of only minor motion and spent a considerable amount of effort to support Amazon’s goal of reducing our devices’ power consumption. We describe these in more detail below.
Hardware variations and environmental conditions
Using onboard loudspeakers and microphones for ultrasound transmission and sensing meant that we had to manage variable acoustic characteristics. Mass-produced devices are known to have a certain variation in amplitude and phase response, and it is very difficult to control the response of loudspeakers in the ultrasonic frequency range without affecting yield rates. To manage these hardware variations and environmental variations, we designed automatic device calibration modules to tailor emission frequencies and levels to both the devices’ hardware idiosyncrasies and the acoustic properties of the rooms in which they are used. This helped us provide a consistent user experience across devices without increasing device costs.
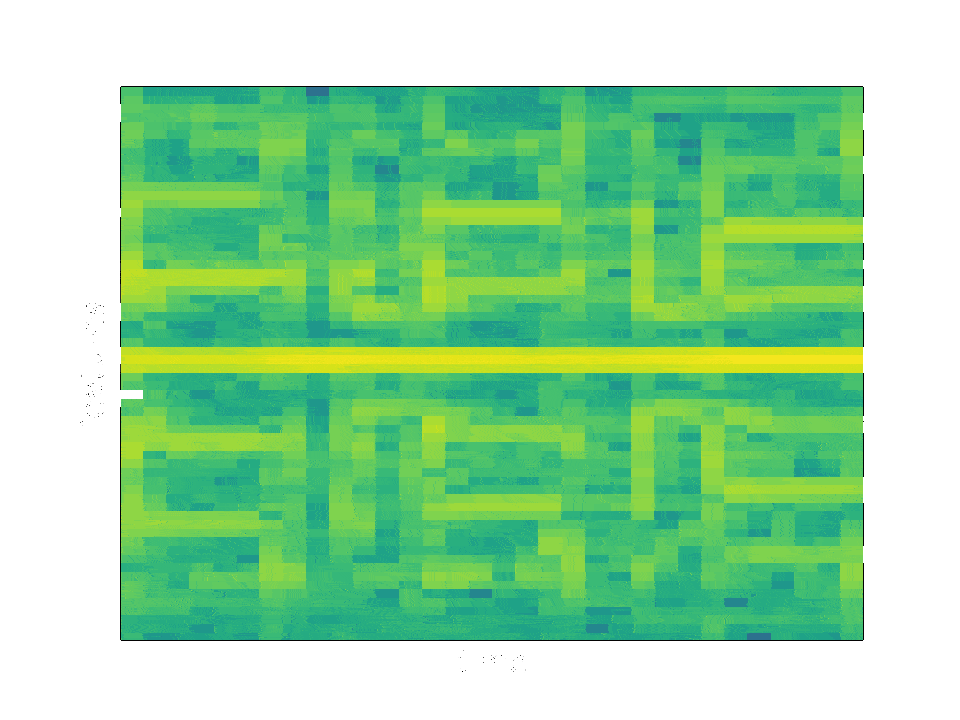
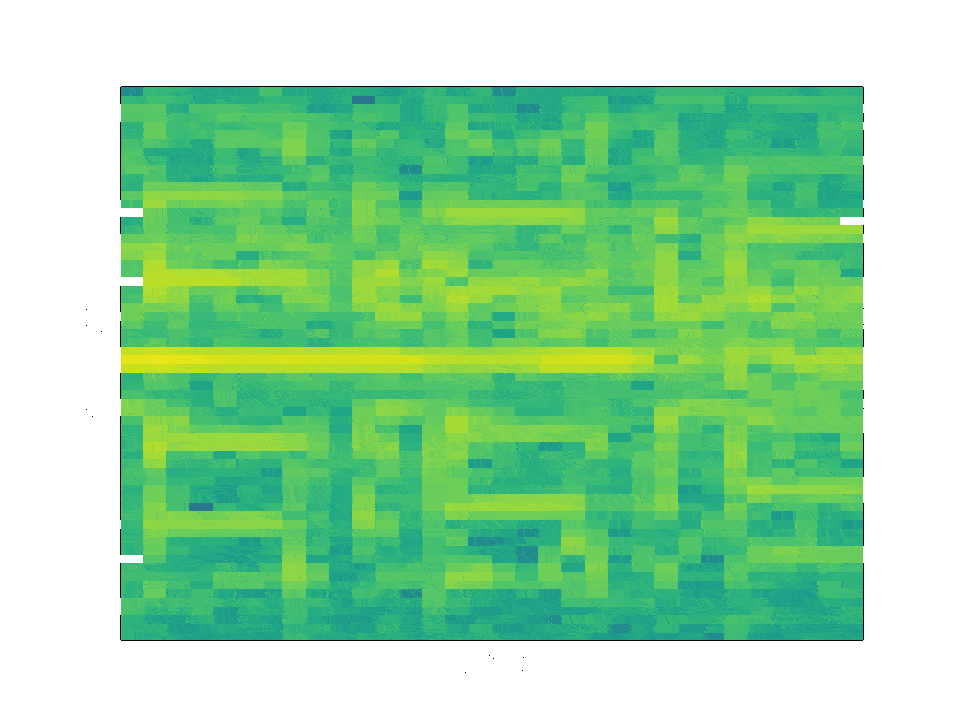
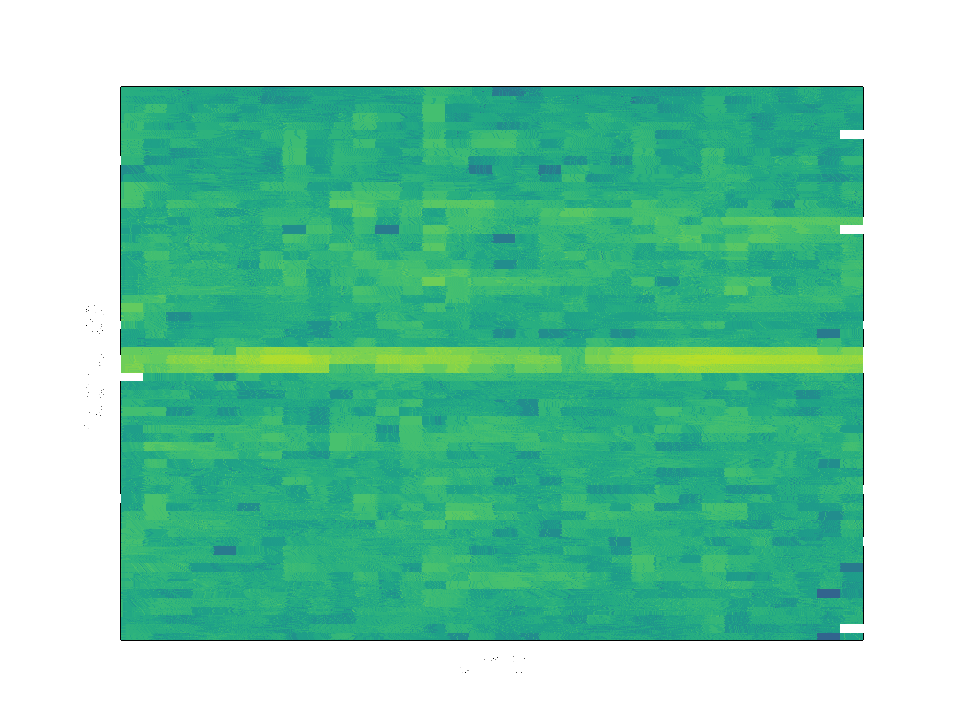
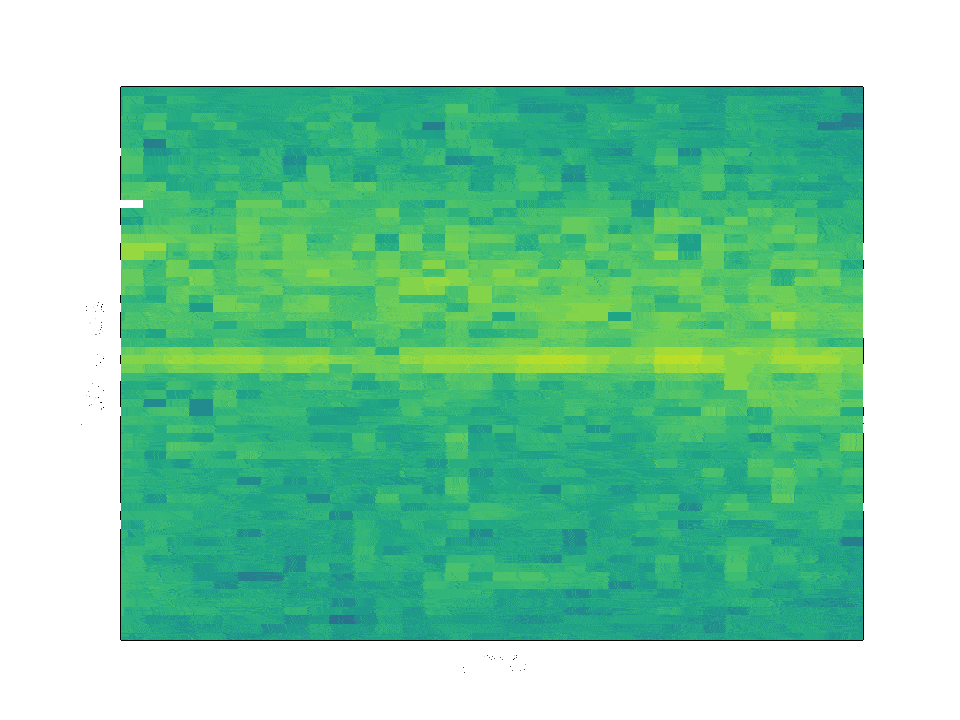
Sensing with concurrent music playback
Music playback is a key use case for Echo devices, which poses challenges, since we use device loudspeakers to simultaneously play music and emit ultrasound. Specifically, when low-frequency music content (such as bass sounds) is played together with an ultrasonic signal, the distortion shows up as noise in the ultrasound region. This noise is inaudible to listeners, but it interferes with the frequencies we use for sensing.
In order to enhance the ultrasound signal and get reasonable range performance in the presence of concurrent music, we developed an adaptive algorithm that uses the different magnitude and phase of distortion and motion features at different microphones to identify and remove distortion.
Major and minor motion
Human movements can be broadly categorized as either major or minor. Major movements include walking into or through an area, while minor movements include reaching for a telephone while seated, turning the pages in a book, opening a file folder, and picking up a coffee cup. Detecting minor movements is difficult, as their ultrasound spectra have very low signal-to-noise ratios (SNRs) compared to major movements, and detecting low-SNR events often means high false-positive rates. At the same time, detecting minor movements is extremely important for recognizing a user’s continued presence after walking into the room.
We developed an algorithm that changes the sensitivity of the detector based on context, such as time elapsed since the last major movement. After a customer walks into the room, the device operates at high sensitivity to detect minor movements for continued presence sensing, so we can provide the best of both worlds — high sensitivity to movements and low false-alarm rates.
Low-power mode
Reducing power consumption is an important goal at Amazon, so we implemented our solution on a low-power digital signal processor (DSP). This required a lot of code and optimizations of the neural-network architecture.
Specifically, as real-time systems, DSPs have strict computation schedules and budgets. This prevented us from deploying deeper neural network models, but we managed to trade off detection latency (on the order of 50 milliseconds) for higher accuracy by combining our neural models with custom DSP implementations. In addition, we disable ultrasonic emission when it is not essential; for example, we disable emissions for set periods of time after detecting presence.
The launch of far-field ultrasonic motion sensing on Echo devices is an exciting development, which will enable our customers to easily automate their day-to-day needs. We are looking forward to inventing more on behalf of our customers.
Acknowledgments: Special thanks to Tarun Pruthi for his contributions to this post.