In the past few years, advances in artificial intelligence have captured our imaginations and led to the widespread use of voice services on our phones and in our homes. This shift in human-computer interaction represents a significant departure from the on-screen way we’ve interacted with our computing devices since the beginning of the modern computing era.
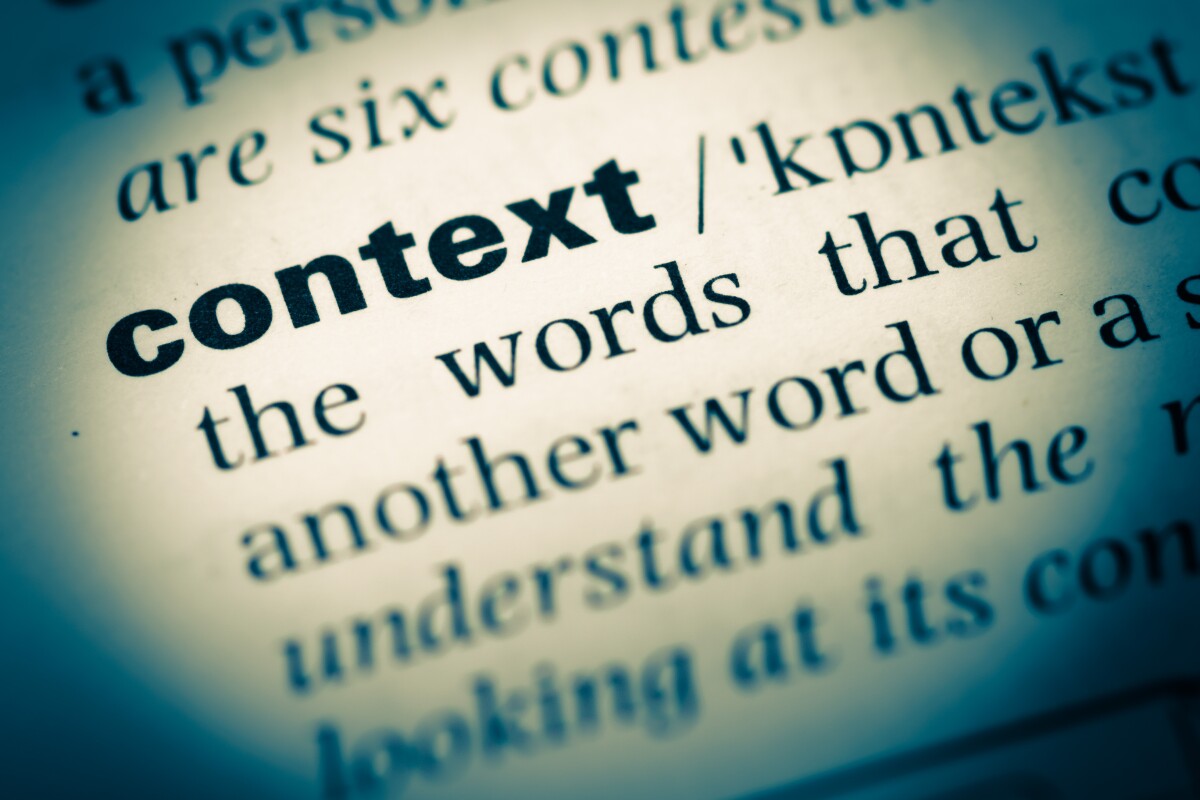
Substantial advances in machine learning technologies have enabled this, allowing systems like Alexa to act on customer requests by translating speech to text, and then translating that text into actions. In an invited talk at the second NeurIPS workshop on Conversational AI later this morning, I’ll focus on the role of context in redefining human-computer interaction through natural language, and discuss how we use context of various kinds to improve the accuracy of Alexa’s deep-learning systems to reduce friction and provide customers with the most relevant responses. I’ll also provide an update on how we’ve expanded the geographic reach of several interconnected capabilities (some new) that use context to improve customer experiences.
There has been remarkable progress in conversational AI systems this decade, thanks in large part to the power of cloud computing, the abundance of the data required to train AI systems, and improvements in foundational AI algorithms. Increasingly, though, as customers expand their conversational-AI horizons, they expect Alexa to interpret their requests contextually; provide more personal, contextually relevant responses; expand her knowledge and reasoning capabilities; and learn from her mistakes.
As conversational AI systems expand to more use cases within and outside the home, to the car, the workplace and beyond, the challenges posed by ambiguous expressions are magnified. Understanding the user’s context is key to interpreting a customer’s utterance and providing the most relevant response. Alexa is using an expanding number of contextual signals to resolve ambiguity, from personal customer context (historical activity, preferences, memory, etc.), skill context (skill ratings, categories, usage), and existing session context, to physical context (is the device in a home, car, hotel, office?) and device context (does the device have a screen? what other devices does it control, and what is their operational state?).
Earlier this fall, Rohit Prasad, Alexa AI vice president and head scientist, announced we would be implementing new Alexa self-learning techniques to help her learn at a faster pace. Earlier this week we launched in the U.S. a new self-learning system that detects the defects in Alexa’s understanding and automatically recovers from these errors. This system is unsupervised, meaning that it doesn’t involve any manual human annotation; instead, it takes advantage of customers’ implicit or explicit contextual signals to detect unsatisfactory interactions or failures of understanding. The system learns how to address these issues and automatically deploys fixes to our production systems shortly after.
For example, during our beta phase, the system automatically learned to associate the utterance “Play ‘Good for What’” to “Play ‘Nice for What’”, correcting a customer’s error and leading to a successful outcome in requesting a song by Drake. This system is currently applying corrections to a large number of music-related utterances each day, helping decrease customer interaction friction for the most popular use of Alexa-compatible devices. We’ll be looking to expand the use of this self-learning capability in the months ahead.
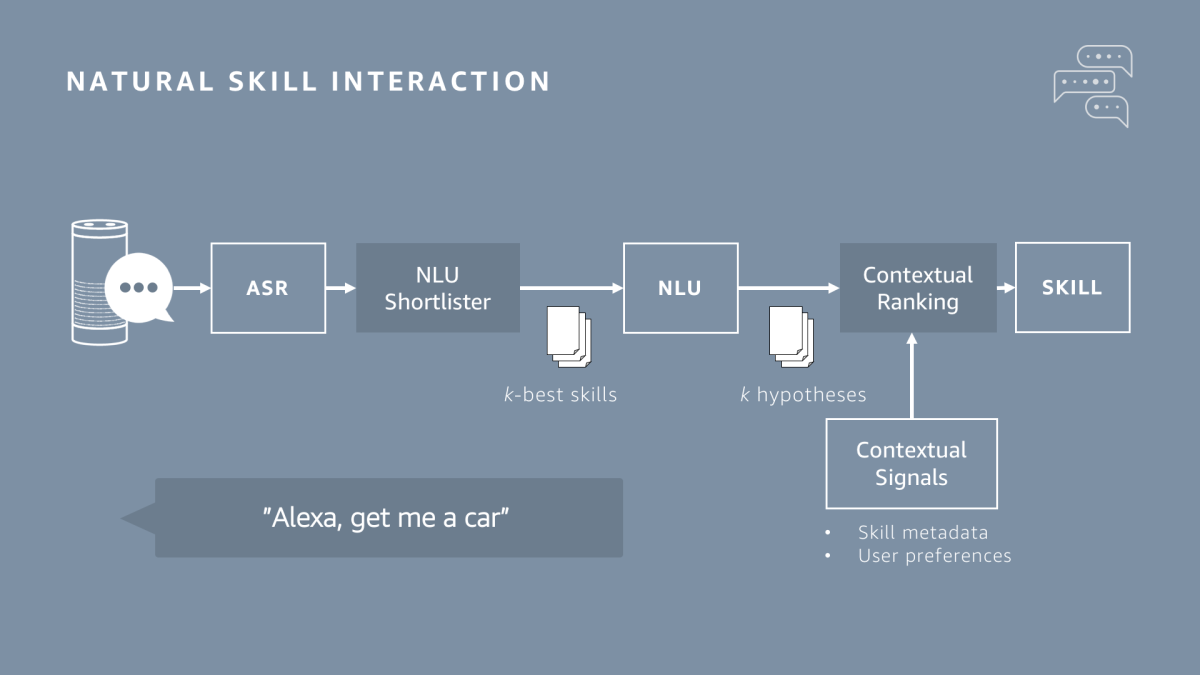
Our vision is for Alexa to help you with whatever you need. Alexa skills and the developers who build them are incredibly important to that vision. There are now hundreds of thousands of developers and device makers building Alexa experiences, as evidenced by the more than 50,000 skills now available. In a post published earlier this year, my colleague Young-Bum Kim described the machine-learning system we’re using to perform name-free skill interaction, which lets customers more naturally discover, enable, and launch Alexa skills. For example, to order a car, a customer can just say, “Alexa, get me a car”, instead of having to specify the name of the ride-sharing service. This requires a system that can process many contextual signals to automatically select the best skill to handle a particular request.
We recently expanded the use of this system beyond the U.S.: customers in the U.K., Canada, Australia, India, Germany, and Japan can now discover and engage with select skills in a more natural way. For example, when customers in Germany say “Alexa, welche stationen kennst du?” (“Alexa, what stations do you know?”) Alexa will reply “Der Skill Radio Brocken kann dir dabei helfen. Möchtest du ihn aktivieren?” (“The skill Radio Brocken can help. Do you want to enable it?”).
With more than 20,000 smart-home devices from more than 3,500 unique brands now compatible with Alexa, smart home use cases especially benefit, as we combine customer, session, and device context to provide more-natural experiences for our customers. For example, if you own an Alexa-compatible iRobot Roomba robot vacuum and say “Alexa, start cleaning”, your Roomba will get to work. Previously, you would have to remember the skill by saying, “Alexa, ask Roomba to start cleaning.” We have enabled this more natural interaction style for a subset of smart home skills and will gradually make this available to more smart home skills and customers in the U.S
Additionally, my colleague Arpit Gupta described in a post earlier this year our solution to the problem of slot carryover, a crucial aspect of the context carryover capability we’ve phased into the Alexa experience this year. To engage in more natural spoken interactions, Alexa must track references through several rounds of conversation. For example, if a customer says “What’s the weather in Seattle?” and, after Alexa’s response, says “How about Boston?”, Alexa infers that the customer is asking about the weather in Boston. If, after Alexa’s response about the weather in Boston, the customer asks, “Any good restaurants there?”, Alexa infers that the customer is asking about restaurants in Boston.
We initially launched context carryover in the U.S. earlier this year. Recently we’ve extended this friction-reducing capability to customers in Canada, the U.K., Australia, New Zealand, India, and Germany.
Context carryover makes interactions with Alexa more natural, and Follow-Up Mode amplifies this experience by letting customers utter a series of requests without repeating the wake word “Alexa.” Follow-Up Mode depends on distinguishing the “signal” of follow-up requests from the “noise” of background conversations or TV audio. My colleague Harish Mallidi described the science behind Follow-Up Mode in a paper published this fall.
Earlier this year, we made Follow-Up Mode available in the U.S., and recently we’ve expanded its availability to Canada, the U.K., Australia, New Zealand, India, and Germany. Perhaps not surprisingly, we’ve found that customers who use Follow-Up Mode have more interactions with Alexa than those who don’t.
The road ahead
As I indicated in a previous post, we’re on a multiyear journey to fundamentally change human-computer interaction. It’s still Day 1, and not unlike the early days of the Internet, when some suggested that the metaphor of a market best described the technology’s future. Nearly a quarter-century later, a market segment is forming around Alexa, and it’s clear that for that market segment to thrive, we must expand our use of contextual signals to reduce ambiguity and friction and increase customer satisfaction.