At a recent press event on Alexa's latest features, Alexa’s head scientist, Rohit Prasad, mentioned multistep requests in one shot, a capability that allows you to ask Alexa to do multiple things at once. For example, you might say, “Alexa, add bananas, peanut butter, and paper towels to my shopping list.” Alexa should intelligently figure out that “peanut butter” and “paper towels” name two items, not four, and that bananas are a separate item.
Typical spoken-language understanding (SLU) systems map user utterances to intents (actions) and slots (entity names). For example, the utterance “What is the weather like in Boston?” has one intent, WeatherInfo, and one slot type, CityName, whose value is “Boston.” This is an example of a “narrow parse”.
Narrow parsers usually have rigid constraints, such as allowing only one intent to be associated with an utterance and only one value to be associated with a slot type. In a paper that we’re presenting at this year’s IEEE Spoken Language Technology conference (SLT 2018), we discuss a way to enable SLU systems to understand compound entities and intents.
We augment an SLU system with a domain-agnostic parser that can identify syntactic elements that establish relationships between different parts of an utterance, or “coordination structures”. In tests comparing our system to an off-the-shelf broad syntactic parser, we show a 26% increase in accuracy.
Off-the-shelf broad parsers are intended to detect coordination structures, but they are often trained on written text with correct punctuation. Automatic speech recognition (ASR) outputs, by contrast, often lack punctuation, and spoken language has different syntactic patterns than written language.
Our new parser is a deep neural network that we train on varied coordination structures in spoken-language data. The training examples are labeled according to the BIO scheme, which indicates groups of words — or “chunks” — that should be treated as ensembles. Most of the words in a sample utterance are labeled with either a B, to indicate the beginning of a chunk, an I, to indicate the inside of a chunk, or an O, to indicate a word that lies outside a chunk. Coordinating conjunctions such as “and” are labeled independently.
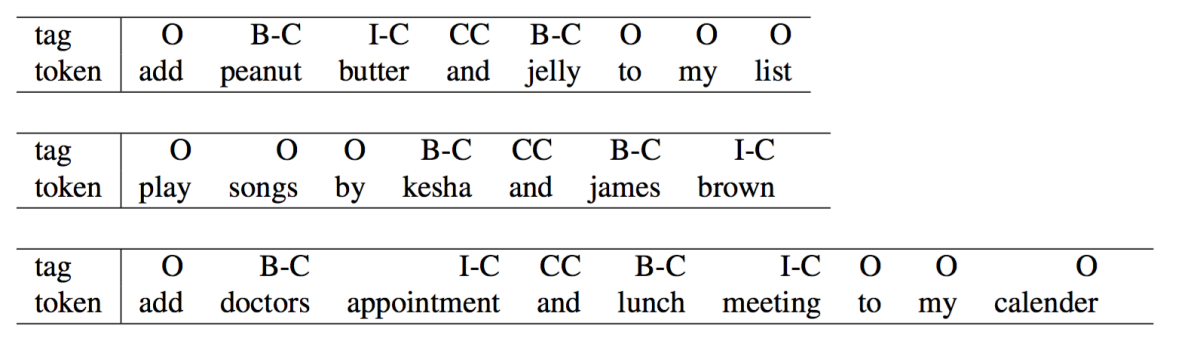
Inputs to the network undergo embedding, a process that represents words as points (vectors) in high-dimensional spaces. We experiment with two different types of embeddings: word embeddings, in which words with similar functions are clustered together, and character embeddings, in which words that contain the same subsequences of letters are clustered together. Character embeddings are useful for systems like ours that are intended to generalize across contexts, because they enable educated guesses about the meanings of unfamiliar words on the basis of their roots, prefixes, and suffixes.
The embeddings then pass through a bidirectional long short-term memory. Long short-term memories (LSTMs) are networks that process sequential data in order, and any given output factors in the outputs that precede it. Bidirectional LSTMs run through a given data sequence both forward and backward.
The output of the bi-LSTM is a contextual embedding of each word in the input sentence, which captures information about its position in the sentence. On top of the bi-LSTM layer, we have a dense layer to map each contextual word embedding to a distribution over the output labels, which are the B, I, and O of the BIO scheme.
In our experiments, we evaluated a system that classifies each word of the input according to its most probable output label. But we got better results by passing the label estimates through a conditional-random-field (CRF) layer. The CRF layer learns associations between output labels and chooses the most likely sequence of output labels from all possible sequences.
When we relied directly on the output of the label-prediction layer, it was possible to get incoherent outputs — such as an O tag followed by an I tag — since each label is independently predicted. The CRF layer, however, would learn that such a sequence of output labels is unlikely and assign it a low score.
Instead of building separate parsers for different slot types (such as ListItem, FoodItem, Appliance, etc.), we built one parser that can handle multiple slot types. For example, our parser can successfully identify ListItems in the utterance “add apples peanut butter and jelly to my list” and Appliances in the utterance “turn on the living room light and kitchen light”.
To enable our model to generalize, we used a technique called adversarial training. During training, the output of the bi-LSTM layer passes not only to the densely connected layer that learns to predict BIO labels but to another layer that learns to predict domains, or broad topic categories such as Weather, Music, and Groceries. The network is evaluated not only on how well it predicts BIO labels but on how badly it predicts domain labels. That way, we ensure that it learns chunking cues that are not domain-specific.
To generate data for our experiments, we take annotated single-entity data and replace the single entities with two or more entities of the same slot type with coordinating conjunctions between the penultimate entity and the final entity. For example, the utterance “set the timer for five minutes” might become “set the timer for five and ten minutes”.
We ran an extensive set of experiments with variations in how the word embeddings are initialized (randomly or with pretrained embeddings, such as FastText, that group words together by meaning), whether or not we use character embeddings, and whether or not we use the CRF layer at the top. We tested separately on two-entity, three-entity, and four-or-more-entity utterances and measured accuracy.
We noticed a few general trends: irrespective of the model configuration, accuracy degrades as we move from two- to three- to four-or-more-entity utterances; initialization with pretrained embeddings usually helps; and adding a CRF layer almost always improves accuracy. Our best results — the ones that improved most on the off-the-shelf parser — came from a model that used character embeddings, pretrained FastText embeddings, and a CRF layer.
Acknowledgments: Rahul Goel, Tagyoung Chung, Abhishek Sethi, Arindam Mandal, Spyros Matsoukas