In recent years, deep neural networks have been responsible for most top-performing AI systems. In particular, natural-language processing (NLP) applications are generally built atop Transformer-based language models such as BERT.
One exception to the deep-learning revolution has been applications that rely on data stored in tables, where machine learning approaches based on decision trees have tended to work better.
At Amazon Web Services, we have been working to extend Transformers from NLP to table data with TabTransformer, a novel, deep, tabular, data-modeling architecture for supervised and semi-supervised learning.
Starting today, TabTransformer is available through Amazon SageMaker JumpStart, where it can be used for both classification and regression tasks. TabTransformer can be accessed through the SageMaker JumpStart UI inside of SageMaker Studio or through Python code using SageMaker Python SDK. To get started with TabTransformer on SageMaker JumpStart, please refer to the program documentation.
We are also thrilled to see that TabTransformer has gained attention from people across industries: it has been incorporated into the official repository of Keras, a popular open-source software library for working with deep neural networks, and it has featured in posts on Towards Data Science and Medium. We also presented a paper on the work at the ICLR 2021 Workshop on Weakly Supervised Learning.
The TabTransformer solution
TabTransformer uses Transformers to generate robust data representations — embeddings — for categorical variables, or variables that take on a finite set of discrete values, such as months of the year. Continuous variables (such as numerical values) are processed in a parallel stream.
We exploit a successful methodology from NLP in which a model is pretrained on unlabeled data, to learn a general embedding scheme, then fine-tuned on labeled data, to learn a particular task. We find that this approach increases the accuracy of TabTransformer, too.
In experiments on 15 publicly available datasets, we show that TabTransformer outperforms the state-of-the-art deep-learning methods for tabular data by at least 1.0% on mean AUC, the area under the receiver-operating curve that plots false-positive rate against false-negative rate. We also show that it matches the performance of tree-based ensemble models.
In the semi-supervised setting, when labeled data is scarce, DNNs generally outperform decision-tree-based models, because they are better able to take advantage of unlabeled data. In our semi-supervised experiments, all of the DNNs outperformed decision trees, but with our novel unsupervised pre-training procedure, TabTransformer demonstrated an average 2.1% AUC lift over the strongest DNN benchmark.
Finally, we also demonstrate that the contextual embeddings learned from TabTransformer are highly robust against both missing and noisy data features and provide better interpretability.
Tabular data
To get a sense of the problem our method addresses, consider a table where the rows represent different samples and the columns represent both sample features (predictor variables) and the sample label (the target variable). TabTransformer takes the features of each sample as input and generates an output to best approximate the corresponding label.
In a practical industry setting, where the labels are partially available (i.e., semi-supervised learning scenarios), TabTransformer can be pre-trained on all the samples without any labels and fine-tuned on the labeled samples.
Additionally, companies usually have one large table (e.g., describing customers/products) that contains multiple target variables, and they are interested in analyzing this data in multiple ways. TabTransformer can be pre-trained on the large number of unlabeled samples once and fine-tuned multiple times for multiple target variables.
The architecture of TabTransformer is shown below. In our experiments, we use standard feature-engineering techniques to transform data types such as text, zip codes, and IP addresses into either numeric or categorical features.
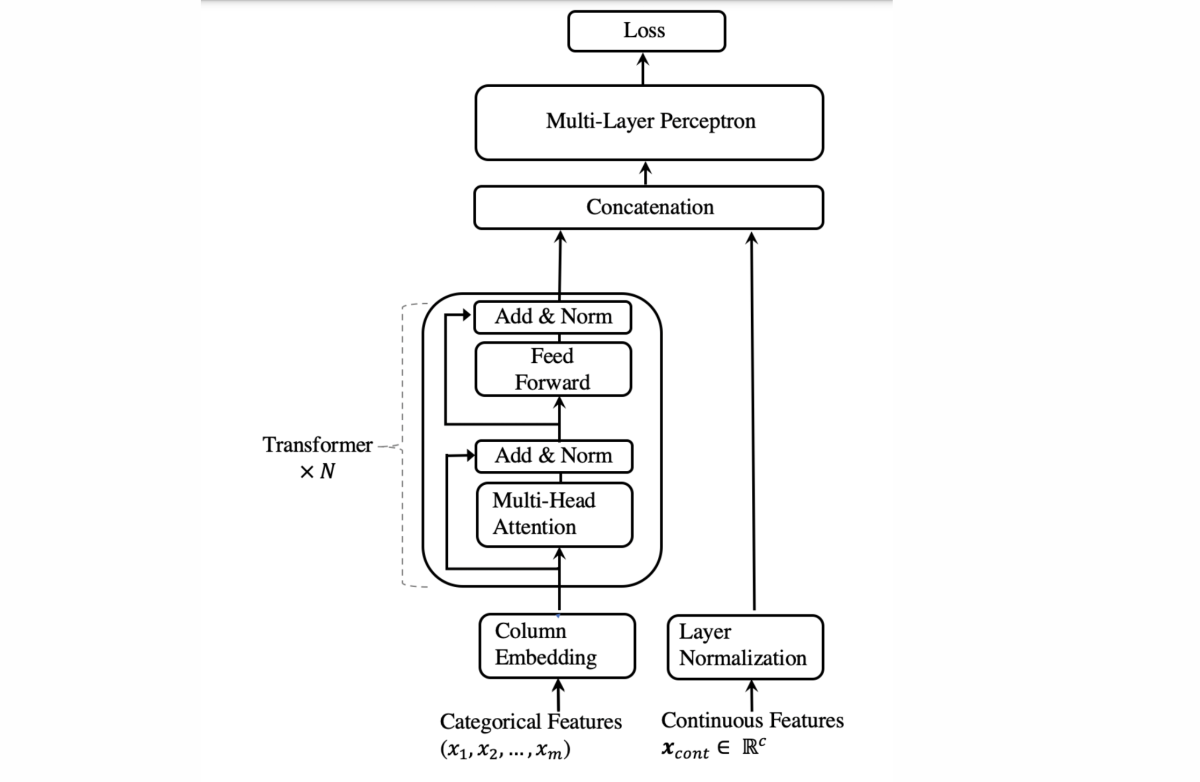
Pretraining procedures
We explore two different types of pre-training procedures: masked language modeling (MLM) and replaced-token detection (RTD). In MLM, for each sample, we randomly select a certain portion of features to be masked and use the embeddings of the other features to reconstruct the masked features. In RTD, for each sample, instead of masking features, we replace them with random values chosen from the same columns.
In addition to comparing TabTransformer to baseline models, we conducted a study to demonstrate the interpretability of the embeddings produced by our contextual-embedding component.
In that study, we took contextual embeddings from different layers of the Transformer and computed a t-distributed stochastic neighbor embedding (t-SNE) to visualize their similarity in function space. More precisely, after training TabTransformer, we pass the categorical features in the test data through our trained model and extract all contextual embeddings (across all columns) from a certain layer of the Transformer. The t-SNE algorithm is then used to reduce each embedding to a 2-D point in the t-SNE plot.
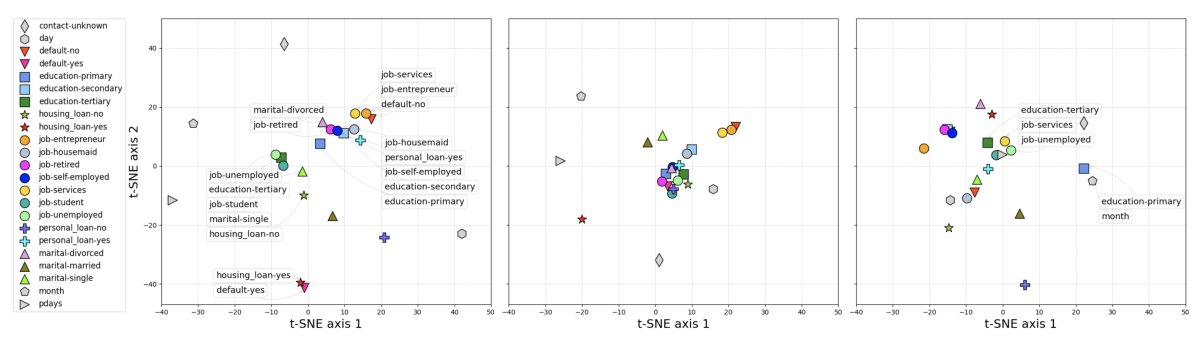
The figure above shows the 2-D visualization of embeddings from the last layer of the Transformer for the dataset bank marketing. We can see that semantically similar classes are close to each other and form clusters (annotated by a set of labels) in the embedding space.
For example, all of the client-based features (colored markers), such as job, education level, and marital status, stay close to the center, and non-client-based features (gray markers), such as month (last contact month of the year) and day (last contact day of the week), lie outside the central area. In the bottom cluster, the embedding of having a housing loan stays close to that of having defaulted, while the embeddings of being a student, single marital status, not having a housing loan, and tertiary education level are close to each other.
The center figure is the t-SNE plot of embeddings before being passed through the Transformer (i.e., from layer 0). The right figure is the t-SNE plot of the embeddings the model produces when the Transformer layers are removed, converting it into an ordinary multilayer perceptron (MLP). In those plots, we do not observe the types of patterns seen in the left plot.
Finally, we conduct extensive experiments on 15 publicly available datasets, using both supervised and semi-supervised learning. In the supervised-learning experiment, TabTransformer matched the performance of the state-of-the-art gradient-boosted decision-tree (GBDT) model and significantly outperformed the prior DNN models TabNet and Deep VIB.
Model name | Mean AUC (%) |
TabTransformer | 82.8 ± 0.4 |
MLP | 81.8 ± 0.4 |
Gradient-boosted decision trees | 82.9 ± 0.4 |
Sparse MLP | 81.4 ± 0.4 |
Logistic regression | 80.4 ± 0.4 |
TabNet | 77.1 ± 0.5 |
Deep VIB | 80.5 ± 0.4 |
Model performance with supervised learning. The evaluation metric is mean standard deviation of AUC score over the 15 datasets for each model. The larger the number, the better the result. The top two numbers are bold.
In the semi-supervised-learning experiment, we pretrain two TabTransformer models on the entire unlabeled set of training data, using the MLM and RTD methods respectively; then we fine-tune both models on labeled data.
As baselines, we use the semi-supervised learning methods pseudo labeling and entropy regularization to train both a TabTransformer network and an ordinary MLP. We also train a gradient-boosted-decision-tree model using pseudo-labeling and an MLP using a pretraining method called the swap-noise denoising autoencoder.
# Labeled data | 50 | 200 | 500 |
TabTransformer-RTD | 66.6 ± 0.6 | 70.9 ± 0.6 | 73.1 ± 0.6 |
TabTransformer-MLM | 66.8 ± 0.6 | 71.0 ± 0.6 | 72.9 ± 0.6 |
ER-MLP | 65.6 ± 0.6 | 69.0 ± 0.6 | 71.0 ± 0.6 |
PL-MLP | 65.4 ± 0.6 | 68.8 ± 0.6 | 71.0 ± 0.6 |
ER-TabTransformer | 62.7 ± 0.6 | 67.1 ± 0.6 | 69.3 ± 0.6 |
PL-TabTransformer | 63.6 ± 0.6 | 67.3 ± 0.7 | 69.3 ± 0.6 |
DAE | 65.2 ± 0.5 | 68.5 ± 0.6 | 71.0 ± 0.6 |
PL-GBDT | 56.5 ± 0.5 | 63.1 ± 0.6 | 66.5 ± 0.7 |
Semi-supervised-learning results on six datasets, each with more than 30,000 unlabeled data points, and different number of labeled data points. Evaluation metric is mean AUC in percentage.
# Labeled data | 50 | 200 | 500 |
TabTransformer-RTD | 78.6 ± 0.6 | 81.6 ± 0.5 | 83.4 ± 0.5 |
TabTransformer-MLM | 78.5 ± 0.6 | 81.0 ± 0.6 | 82.4 ± 0.5 |
ER-MLP | 79.4 ± 0.6 | 81.1 ± 0.6 | 82.3 ± 0.6 |
PL-MLP | 79.1 ± 0.6 | 81.1 ± 0.6 | 82.0 ± 0.6 |
ER-TabTransformer | 77.9 ± 0.6 | 81.2 ± 0.6 | 82.1 ± 0.6 |
PL-TabTransformer | 77.8 ± 0.6 | 81.0 ± 0.6 | 82.1 ± 0.6 |
DAE | 78.5 ± 0.7 | 80.7 ± 0.6 | 82.2 ± 0.6 |
PL-GBDT | 73.4 ± 0.7 | 78.8 ± 0.6 | 81.3 ± 0.6 |
Semi-supervised learning results on nine datasets, each with fewer than 30,000 data points, and different numbers of labeled data points. Evaluation metric is mean AUC in percentage.
To gauge relative performance with different amounts of unlabeled data, we split the set of 15 datasets into two subsets. The first set consists of the six datasets that containing more than 30,000 data points. The second set includes the remaining nine datasets.
When the amount of unlabeled data is large, TabTransformer-RTD and TabTransformer-MLM significantly outperform all the other competitors. Particularly, TabTransformer-RTD/MLM improvement are at least 1.2%, 2.0%, and 2.1% on mean AUC for the scenarios of 50, 200, and 500 labeled data points, respectively. When the number of unlabeled data becomes smaller, as shown in Table 3, TabTransformer-RTD still outperforms most of its competitors but with a marginal improvement.
Acknowledgments: Ashish Khetan, Milan Cvitkovic, Zohar Karnin