A quick guide to Amazon’s innovative work at the IEEE Spoken Language Technology Workshop (SLT), which begins next week:
Accelerator-aware training for transducer-based speech recognition
Suhaila Shakiah, Rupak Vignesh Swaminathan, Hieu Duy Nguyen, Raviteja Chinta, Tariq Afzal, Nathan Susanj, Athanasios Mouchtaris, Grant Strimel, Ariya Rastrow
Machine learning models trained at full precision can suffer performance falloffs when deployed on neural-network accelerator (NNA) chips, which leverage highly parallelized fixed-point arithmetic to improve efficiency. To avoid this problem, Amazon researchers propose a method for emulating NNA operations at training time.
An analysis of the effects of decoding algorithms on fairness in open-ended language generation
Jwala Dhamala, Varun Kumar, Rahul Gupta, Kai-Wei Chang, Aram Galstyan
The researchers systematically study the effects of different decoding algorithms on the fairness of large language models, showing that fairness varies significantly with changes in decoding algorithms’ hyperparameters. They also provide recommendations for reporting decoding details during fairness evaluations and optimizing decoding algorithms.
An experimental study on private aggregation of teacher ensemble learning for end-to-end speech recognition
Chao-Han Huck Yang, I-Fan Chen, Andreas Stolcke, Sabato Marco Siniscalchi, Chin-Hui Lee
For machine learning models, meeting differential-privacy (DP) constraints usually means adding noise to data, which can hurt performance. Amazon researchers apply private aggregation of teacher ensembles (PATE), which uses different noisy models to train a single student model, to automatic speech recognition, reducing word error rate by 26% to 28% while meeting DP constraints.
Exploration of language-specific self-attention parameters for multilingual end-to-end speech recognition
Brady Houston, Katrin Kirchhoff
Multilingual, end-to-end, automatic-speech-recognition models perform better when they’re trained using both language-specific and language-universal model parameters. Amazon researchers show that using language-specific parameters in the attention mechanisms of Conformer-based encoders can improve the performance of ASR models across six languages by up to 12% relative to multilingual baselines and 36% relative to monolingual baselines.
Guided contrastive self-supervised pre-training for automatic speech recognition
Aparna Khare, Minhua Wu, Saurabhchand Bhati, Jasha Droppo, Roland Maas
Contrastive predictive coding (CPC) is a representation-learning method that maximizes the mutual information between a model’s intermediate representations and its output. Amazon researchers present a modification of CPC that maximizes the mutual information between representations from a prior-knowledge model and the output of a model being pretrained, reducing the word error rate relative to CPC pretraining only.
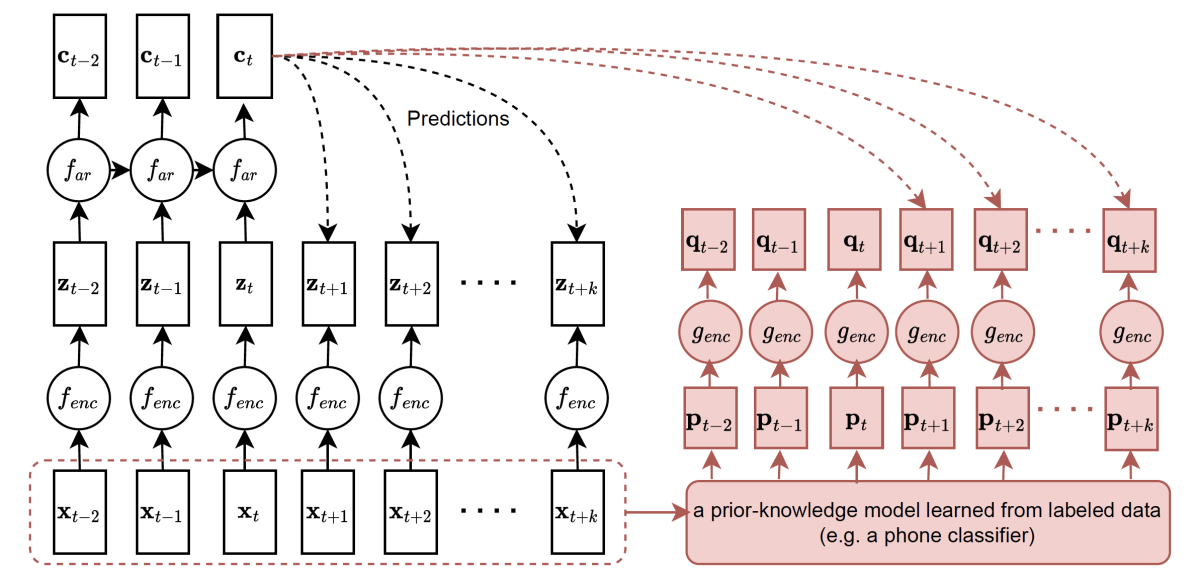
Implicit acoustic echo cancellation for keyword spotting and device-directed speech detection
Samuele Cornell, Thomas Balestri, Thibaud Sénéchal
In realistic human-machine interactions, customer speech can overlap with device playback. Amazon researchers propose a way to improve keyword spotting and device-directed-speech detection in these circumstances. They teach the model to ignore playback audio via an implicit acoustic echo cancellation mechanism. They show that, by conditioning on the reference signal as well as the signal captured at the microphone, they can improve recall by as much as 56%.
Mixture of domain experts for language understanding: An analysis of modularity, task performance, and memory tradeoffs
Benjamin Kleiner, Jack FitzGerald, Haidar Khan, Gokhan Tur
Amazon researchers show that natural-language-understanding models that incorporate mixture-of-experts networks, in which each network layer corresponds to a different domain, are easier to update after deployment, with less effect on performance, than other types of models.
N-best hypotheses reranking for text-to-SQL systems
Lu Zeng, Sree Hari Krishnan Parthasarathi, Dilek Hakkani-Tür
Text-to-SQL models map natural-language requests to structured database queries, and today’s state-of-the-art systems rely on fine-tuning pretrained language models. Amazon researchers improve the coherence of such systems with a model that generates a query plan predicting whether a SQL query contains particular clauses; they improve the correctness of such systems with an algorithm that generates schemata that can be used to match prefixes and abbreviations for slot values (such as “left” and “L”).
On granularity of prosodic representations in expressive text-to-speech
Mikolaj Babianski, Kamil Pokora, Raahil Shah, Rafal Sienkiewicz, Daniel Korzekwa, Viacheslav Klimkov
In expressive-speech synthesis, the same input text can be mapped to different acoustic realizations. Prosodic embeddings at the utterance, word, or phoneme level can be used at training time to simplify that mapping. Amazon researchers study these approaches, showing that utterance-level embeddings have insufficient capacity and phoneme-level embeddings tend to introduce instabilities, while word-level representations strike a balance between capacity and predictability. The researchers use that finding to close the gap in naturalness between synthetic speech and recordings by 90%.
Personalization of CTC speech recognition models
Saket Dingliwal, Monica Sunkara, Srikanth Ronanki, Jeff Farris, Katrin Kirchhoff, Sravan Bodapati
Connectionist temporal classification (CTC) loss functions are an attractive option for automatic speech recognition because they yield simple models with low inference latency. But CTC models are hard to personalize because of their conditional-independence assumption. Amazon researchers propose a battery of techniques to bias a CTC model’s encoder and its beam search decoder, yielding a 60% improvement in F1 score on domain-specific rare words over a strong CTC baseline.
Remap, warp and attend: Non-parallel many-to-many accent conversion with normalizing flows
Abdelhamid Ezzerg, Tom Merritt, Kayoko Yanagisawa, Piotr Bilinski, Magdalena Proszewska, Kamil Pokora, Renard Korzeniowski, Roberto Barra-Chicote, Daniel Korzekwa
Regional accents affect not only how words are pronounced but prosodic aspects of speech such as speaking rate and intonation. Amazon researchers investigate an approach to accent conversion that uses normalizing flows. The approach has three steps: remapping the phonetic conditioning, to better match the target accent; warping the duration of the converted speech, to better suit the target phonemes; and applying an attention mechanism to implicitly align source and target speech sequences.
Residual adapters for targeted updates in RNN-transducer based speech recognition system
Sungjun Han, Deepak Baby, Valentin Mendelev
While it is possible to incrementally fine-tune an RNN-transducer (RNN-T) automatic-speech-recognition model to recognize multiple sets of new words, this creates a dependency between the updates, which is not ideal when we want each update to be applied independently. Amazon researchers propose training residual adapters on the RNN-T model and combining them on the fly through adapter fusion, enabling a recall on new words of more than 90%, with less than 1% relative word error rate degradation.
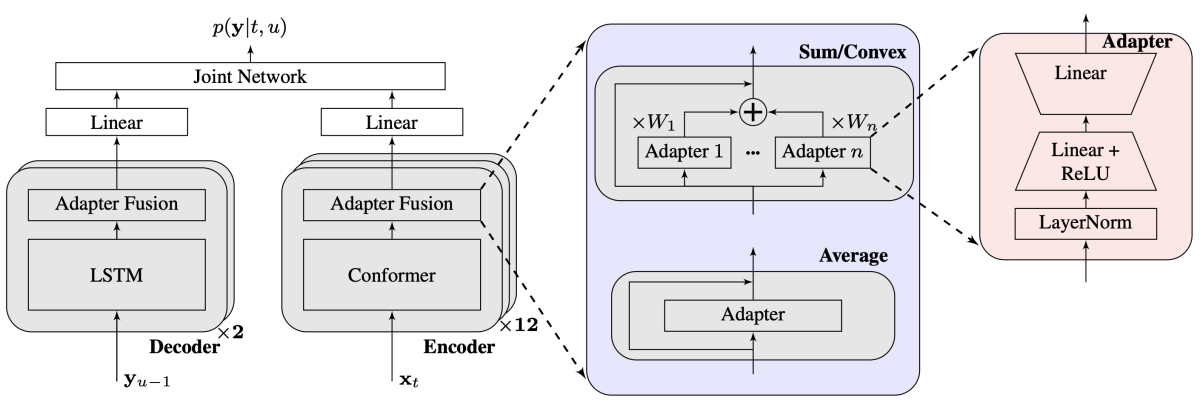
Sub-8-bit quantization for on-device speech recognition: a regularization-free approach
Kai Zhen, Martin Radfar, Hieu Nguyen, Grant Strimel, Nathan Susanj, Athanasios Mouchtaris
For on-device automatic speech recognition (ASR), quantization-aware training (QAT) can help manage the trade-off between performance and efficiency. Among existing QAT methods, one major drawback is that the quantization centroids have to be predetermined and fixed. Amazon researchers introduce a compression mechanism with self-adjustable centroids that results in a simpler yet more versatile quantization scheme that enables a 30.73% memory footprint savings and a 31.75% user-perceived latency reduction, compared to eight-bit QAT.