Amazon is a gold sponsor of this year’s meeting of the Association for the Advancement of Artificial Intelligence (AAAI), and Amazon researchers are coauthors on eight papers accepted to the conference.
Of those papers, Dilek Hakkani-Tür, a senior principal scientist in the Alexa AI group whose research focuses on dialogue systems, is a coauthor on three.
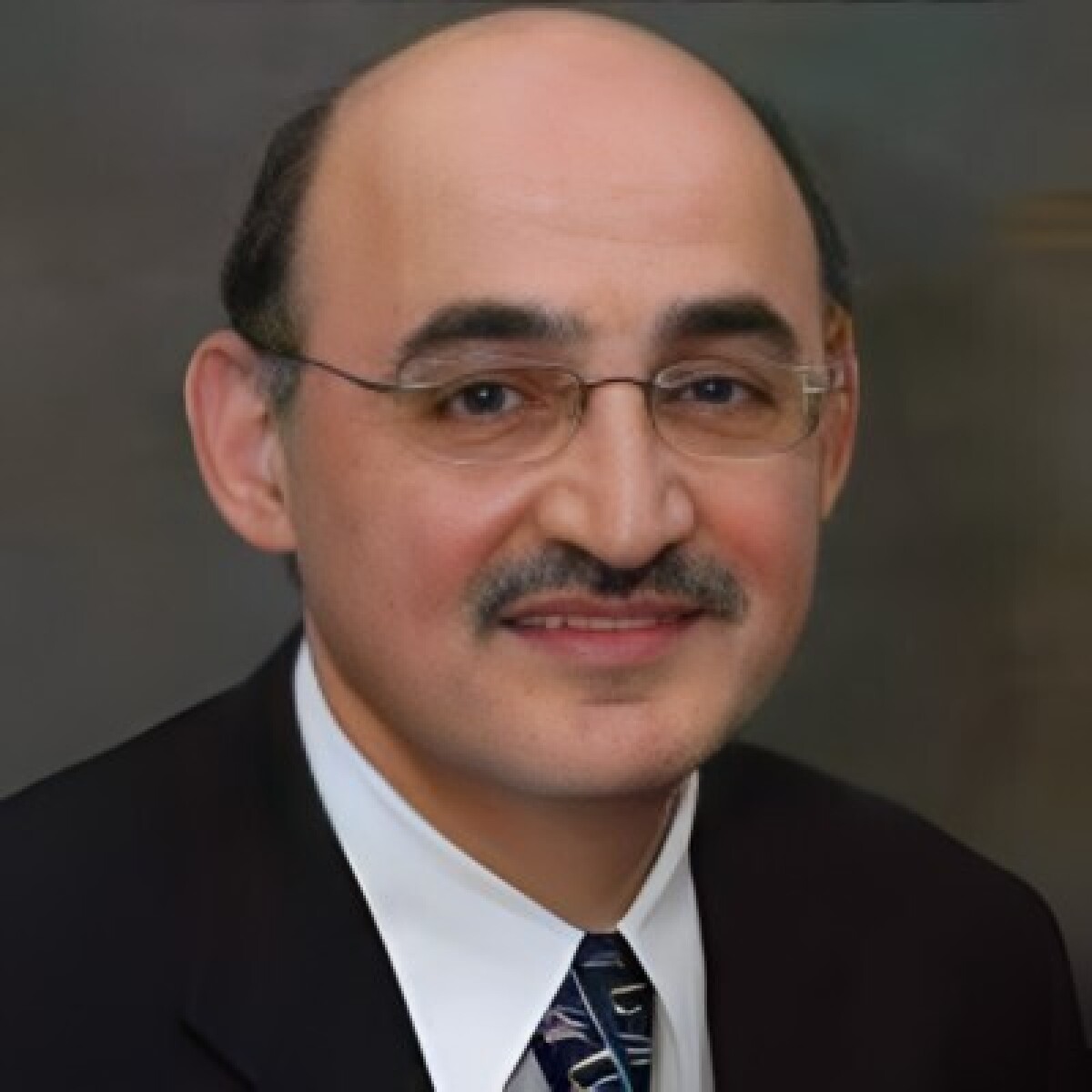
“AAAI’s scope is very broad,” Hakkani-Tür says. “Everything related to AI, you can find papers. We have papers on three quite different topics. Two of them are, yes, on conversational systems — dialogue state tracking, question answering — but the last one is on making human-robot interaction more conversational. And since AAAI covers broad areas of AI, it’s still a good fit.”
Seokhwan Kim, a senior applied scientist in Hakkani-Tür’s group, will also be attending the conference, both as a co-presenter of the paper on human-robot interaction and as the chair of a workshop built around the Eighth Dialog System Technology Challenge, a competition in which industry and academic teams build systems to address outstanding problems in the dialogue systems field. Now in its eighth year, the competition workshop has for the past three years been held at AAAI.
“That also improves AAAI’s attraction for people like me who work on dialogue,” Hakkani-Tür says. At the workshop, Hakkani-Tür says, Alexa researchers will be among the attendees proposing tasks for inclusion in next year’s challenge.
Also at AAAI, Krishnaram Kenthapadi, a principal scientist with Amazon Web Services (AWS), will co-lead a tutorial on explainable AI. Explainable AI, Kenthapadi says, is “an emerging discipline that aims at making the reasoning of artificial-intelligence systems intelligible to humans, thereby enhancing the transparency and trustworthiness of such systems.”
Amazon researchers’ AAAI papers include the following:
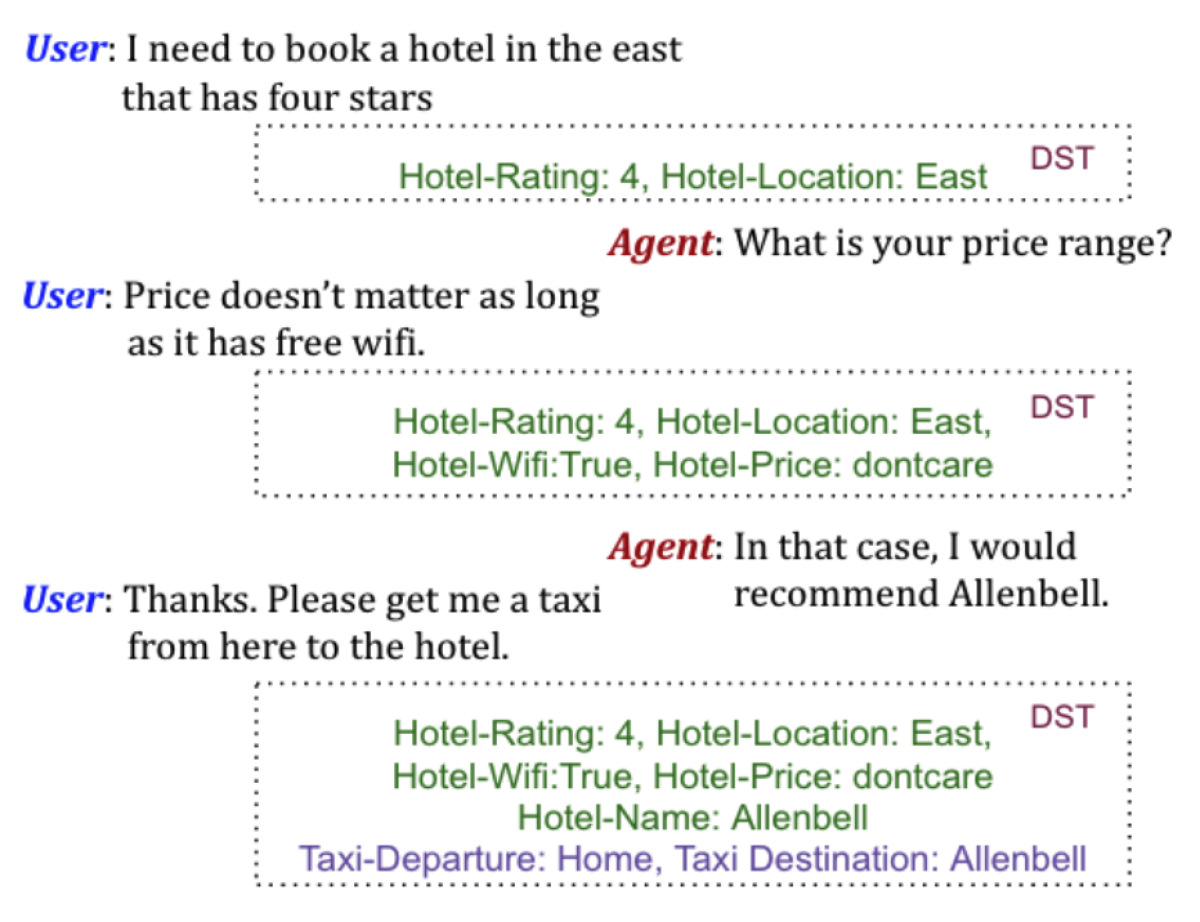
MA-DST: Multi-Attention-Based Scalable Dialog State Tracking
Adarsh Kumar, Peter Ku, Angeliki Metallinou, Anuj Goyal, Dilek Hakkani-Tür
Dialogue systems often need to remember contextual information from early in a conversation, and they can benefit from having a specific component that tracks the “state” of the conversation — the entities mentioned so far. When a customer asks, “Are there any Indian restaurants nearby?”, for instance, the state tracker updates the cuisine variable to “Indian” and remembers that “nearby” refers to the location of the movie theater mentioned two turns ago. Attention mechanisms are a powerful tool for tracking state because they help determine which words in the conversation history are relevant to interpreting the current turn. The researchers use attention at three different layers of granularity to improve the state-of-the-art state tracking accuracy by 5%.
MMM: Multi-stage Multi-task Learning for Multi-choice Reading Comprehension
Di Jin, Shuyang Gao, Jiun-yu Kao, Tagyoung Chung, Dilek Hakkani-Tür
Teaching machine learning systems to answer multiple-choice questions has been difficult, mostly because of the lack of training data. The researchers show that powerful new neural language models, such as BERT, can be adapted to answer multiple-choice questions if the adaptation proceeds in two stages: first, a “coarse tuning” on large natural-language-inference data sets, which encode the logical relationships between pairs of sentences; then, fine-tuning on a domain-specific set of questions and answers. In tests, this method improved the state-of-the-art accuracy on several benchmark data sets by 16%.
Just Ask: An Interactive Learning Framework for Vision and Language Navigation
Ta-Chung Chi, Mihail Eric, Seokhwan Kim, Minmin Shen, Dilek Hakkani-Tür
The researchers show how they use reinforcement learning to train a robot to ask for help when it has difficulty interpreting spoken instructions. When uncertain how to proceed, the robot would send a help request to its operator, who could provide clarification through some combination of verbal and visual feedback. In simulations, the researchers found that a single help request during each navigation task increased the chances of successfully completing the task by 15%.
Feedback-Based Self-Learning in Large-Scale Conversational AI Agents
Pragaash Ponnusamy, Alireza Roshan-Ghias, Chenlei Guo, Ruhi Sarikaya
Alexa customers sometimes cut Alexa off and rephrase requests that don’t elicit the responses they want. The researchers describe how to use implicit signals like these to automatically improve Alexa’s natural-language-understanding models, without the need for human intervention. The key is to model sequences of requests as “absorbing Markov chains”, which describe probabilistic transitions from one request to another and can be used to calculate the likelihood that rewriting a request will result in a better outcome. In tests, the system demonstrated a win/loss ratio of 12, meaning that it learned 12 valid substitutions for every one invalid one. Blog post here.
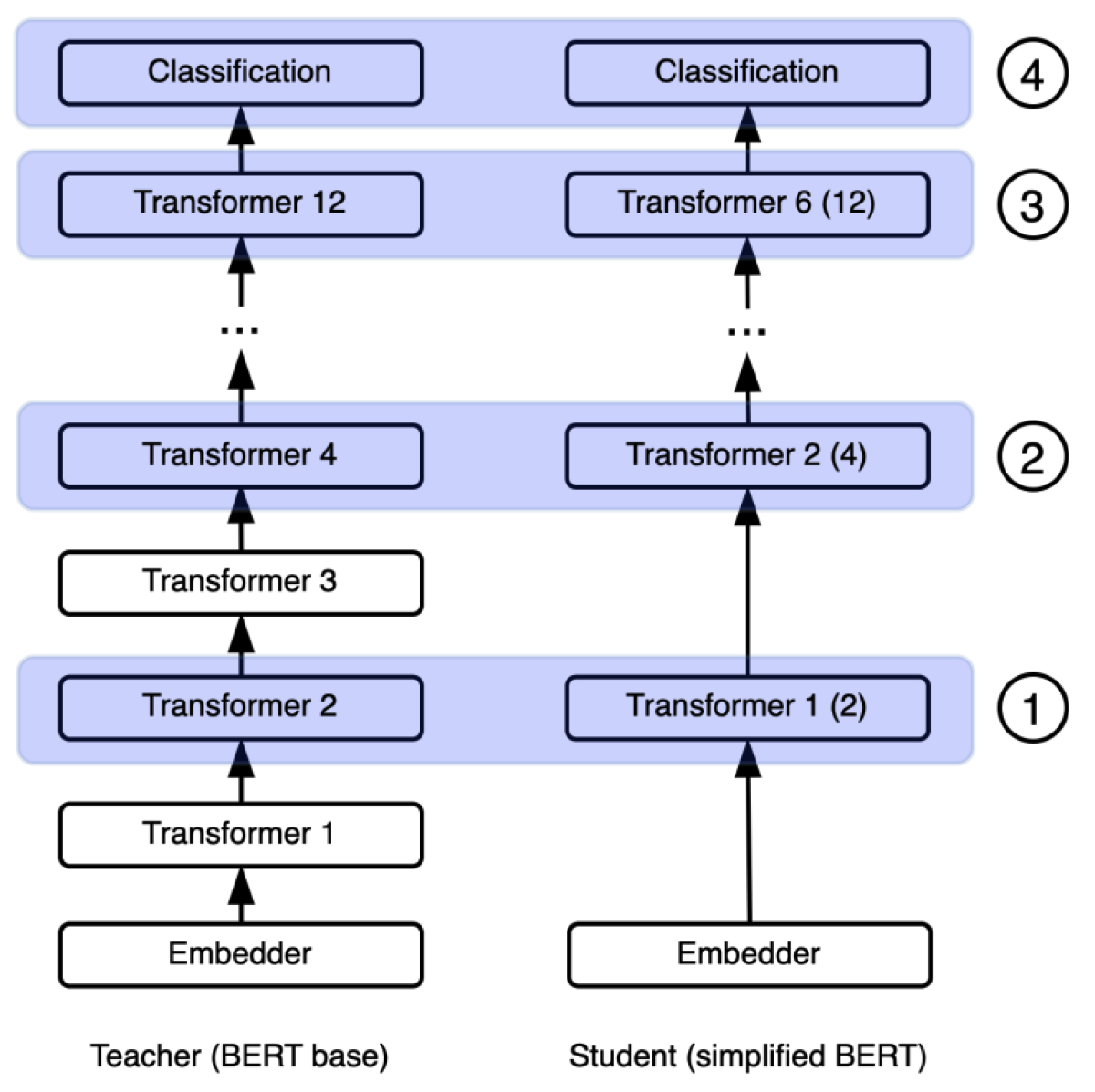
Knowledge Distillation from Internal Representations
Gustavo Aguilar, Yuan Ling, Yu Zhang, Benjamin Yao, Xing Fan, Chenlei Guo
Knowledge distillation is a technique in which a lean, efficient machine learning model is trained to reproduce the outputs of a larger, slower, but more powerful “teacher” model. The more complex the teacher, however, the more difficult its outputs are to reproduce faithfully. The researchers address this limitation by training the “student” model to mimic not only the teacher’s outputs but also the internal states it assumes in producing those outputs. In experiments involving a BERT model, the researchers’ method demonstrated a 5-10% performance improvement on benchmark data sets, including a large new Reddit data set that the authors assembled and have made available for research purposes.
TANDA: Transfer and Adapt Pre-Trained Transformer Models for Answer Sentence Selection
Siddhant Garg, Thuy Vu, Alessandro Moschitti
Answer sentence selection is the process of choosing one sentence among many candidates to serve as the answer to a query. The researchers describe a new technique for adapting powerful language models built atop the Transformer neural network to the problem of answer selection. They first fine-tune the language model on a large, general data set of question-answer pairs, then fine-tune it again on a much smaller, topic-specific data set. In tests on standard benchmarks in the field, they reduce the state-of-the-art error rate by 50%. Blog post here.
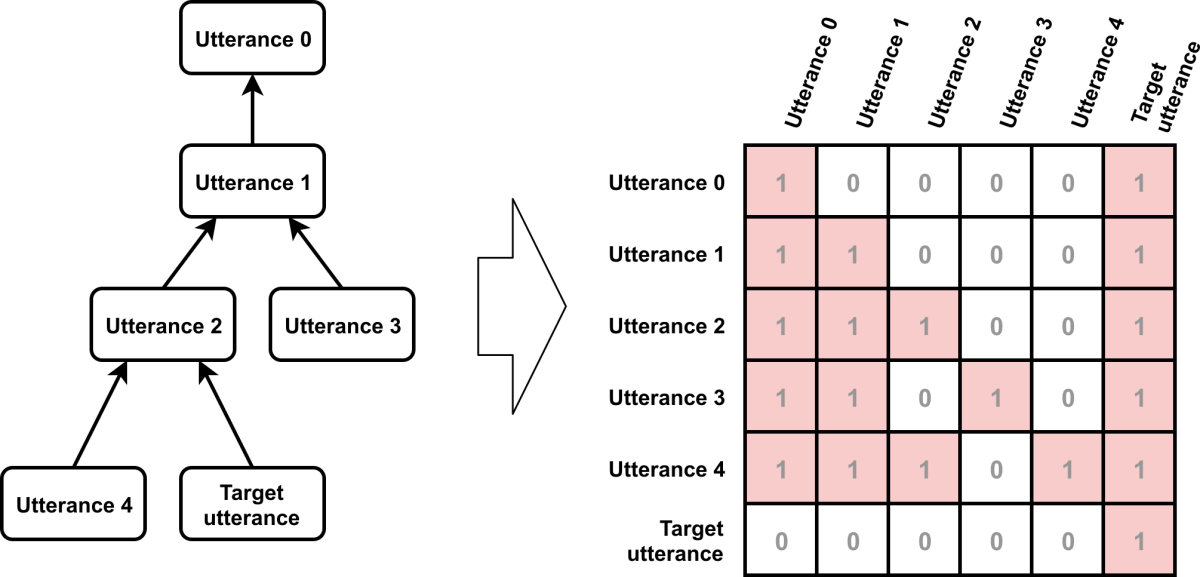
Who Did They Respond To? Conversation Structure Modeling Using Masked Hierarchical Transformer
Henghui Zhu, Feng Nan, Zhiguo Wang, Ramesh Nallapati, Bing Xiang
On online discussion boards or in meeting transcriptions, it can be hard to tell who is responding to which comment by whom. The researchers describe a new method for training machine learning models to determine the “reply-to” structure of such conversations. They use an attention mechanism, which determines how much weight to give prior comments when identifying the antecedent of the current comment. But they enforce a hierarchical conversational structure by “masking” — or setting to zero — the attention weights on comments that are not ancestors of a given candidate antecedent. In tests that involved inferring conversation structure, their method yielded significant accuracy improvements over the previous state-of-the-art system on multiple data sets.
At the conference’s Workshop on Interactive and Conversational Recommendation Systems, Alexa researchers will also present a paper titled “What Do You Mean I’m Funny? Personalizing the Joke Skill of a Voice-Controlled Virtual Assistant”. To automatically label the training data fed to a joke skill’s machine learning models, the researchers experimented with two implicit signals of joke satisfaction: customer requests for additional jokes either within 5 minutes or between 1 and 25 hours after the initial joke. Models trained on both types of implicit signals outperformed existing baseline models.