Every interaction with Alexa begins with the wake word: usually “Alexa”, but sometimes “Amazon”, “Echo”, or “Computer” — or, now, “Hey Samuel”. Only after positively identifying the wake word does an Alexa-enabled device send your request to the cloud for further processing.
Six years after the announcement of the first Amazon Echo, the Alexa science team continues to innovate new approaches to wake word recognition, improving Alexa’s responsiveness and accuracy.
At this year’s Interspeech, for instance, Alexa researchers presented five different papers about new techniques for wake word recognition. One of these — “Building a robust word-level wakeword verification network” — describes models that run in the cloud to confirm on-device wake word detections.
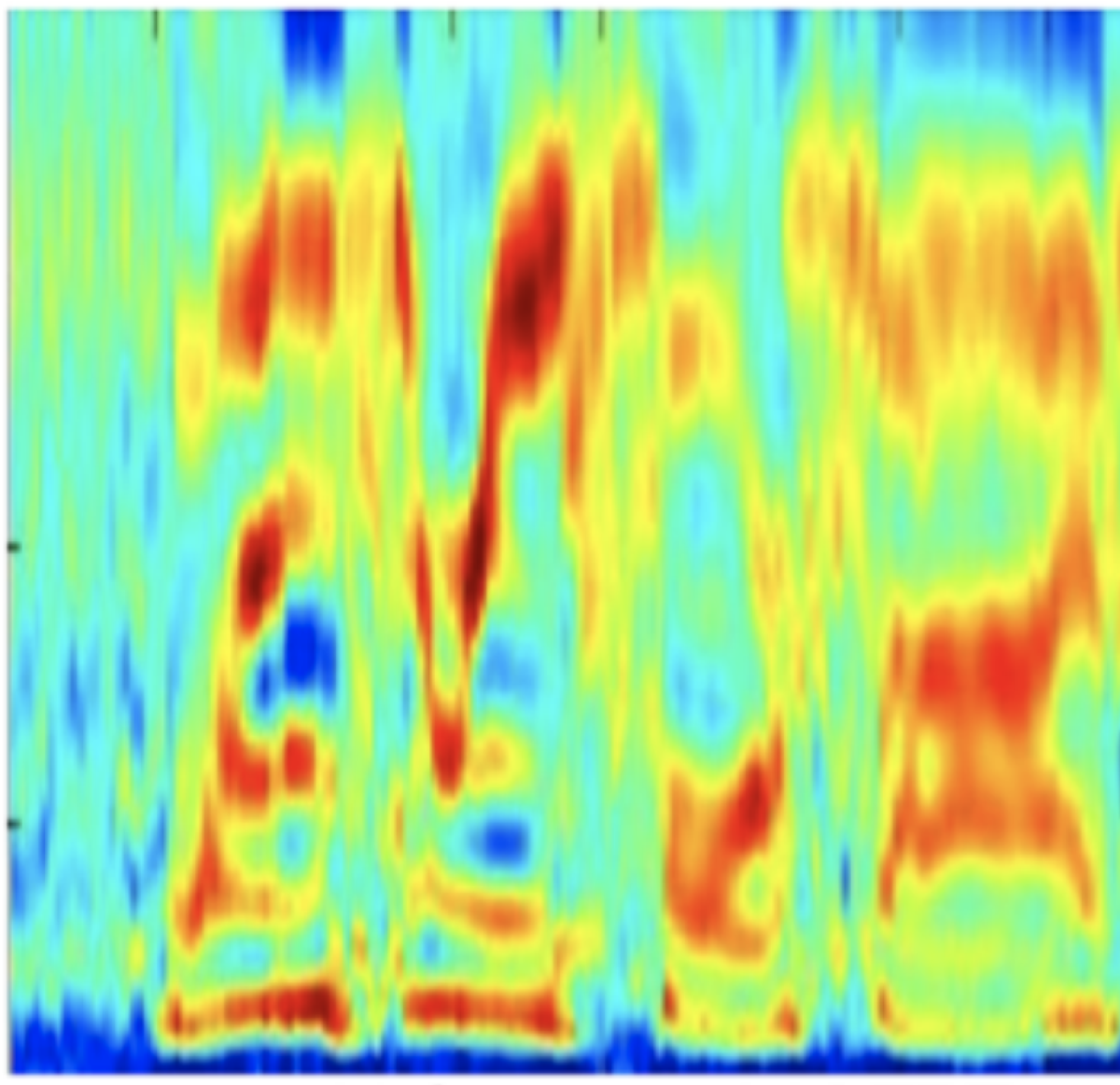
Another paper, “Metadata-aware end-to-end keyword spotting”, describes a new system that uses metadata about the state of the Alexa-enabled device — such as the type of device and whether it’s playing music or sounding an alarm — to improve the accuracy of the on-device wake word detector.
The wake word detectors reported in both papers rely, at least in part, on convolutional neural networks. Originally developed for image processing, convolutional neural nets, or CNNs, repeatedly apply the same “filter” to small chunks of input data. For object recognition, for instance, a CNN might step through an image file in eight-by-eight blocks of pixels, inspecting each block for patterns associated with particular objects.
Since audio signals can be represented as two-dimensional mappings of frequency against time, CNNs apply naturally to them as well. Each of the filters applied to a CNN’s inputs defines a channel through the first layer of the CNN, and usually, the number of channels increases with every layer.
Varying norms
“Metadata-aware end-to-end keyword spotting” is motivated by the observation that if a device is emitting sound — music, synthesized speech, or an alarm sound — it causes a marked shift in the input signal’s log filter bank energies, or LFBEs. The log filter banks are a set of differently sized frequency bands chosen to emphasize the frequencies in which human hearing is most acute.
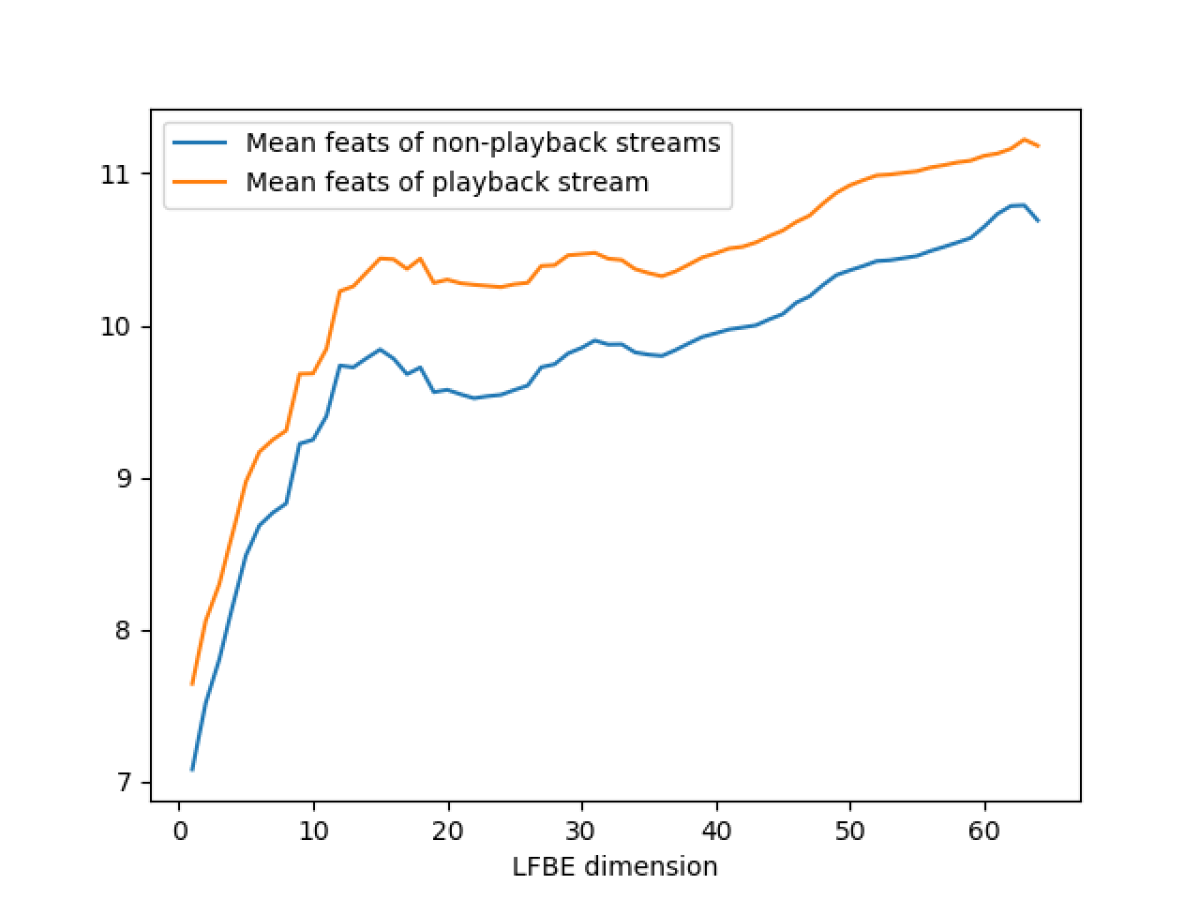
To address this problem, applied scientists Hongyi Liu and Apurva Abhyankar and their colleagues include device metadata as an input to their wake word model. The model embeds the metadata, or represents it as points in a multidimensional space, such that location in the space conveys information useful to the model. The model uses the embeddings in two different ways.
One is as an additional input to the last few layers of the network, which decide whether the acoustic input signal includes the wake word. The final outputs of the convolutional layers are flattened, or strung together into a single long vector. The metadata embedding vector is fed into a fully connected layer — a layer all of whose processing nodes pass their outputs to all of the nodes of the next layer — and the output is concatenated to the flattened audio feature vector.
This fused vector passes to a final fully connected layer, which issues a judgment about whether the network contains the wake word or not.
The other use of the metadata embedding is to modulate the outputs of the convolutional layers while they’re processing the input signal. The filters that a CNN applies to inputs are learned during training, and they can vary greatly in size. Consequently, the magnitude of the values passing through the network’s various channels can vary as well.
With CNNs, it’s common practice to normalize the channels’ outputs between layers, so that they’re all on a similar scale, and no one channel swamps the others. But Liu, Abhyankar, and their colleagues train their model to vary the normalization parameters depending on the metadata vector, which improves the network’s ability to generalize to heterogenous data sets.
The researchers believe that this model better captures the characteristics of the input audio signal when the Alexa-enabled device is emitting sound. In their paper, they report experiments showing that, on average, a model trained with metadata information achieves a 14.6% improvement in false-reject rate relative to a baseline CNN model.
Paying attention
The metadata-aware wake word detector runs on-device, but the next two papers describe models that run in the cloud. On-device models must have small memory footprints, which means that they sacrifice some processing power. If an on-device model thinks it has detected a wake word, it sends a short snippet of audio to the cloud for confirmation by a larger, more-powerful model.
The on-device model tries to identify the start of the wake word, but sometimes it misses slightly. To ensure that the cloud-based model receives the whole wake word, the snippet sent by the device includes the half-second of audio preceding the device’s estimate of the wake word’s start.
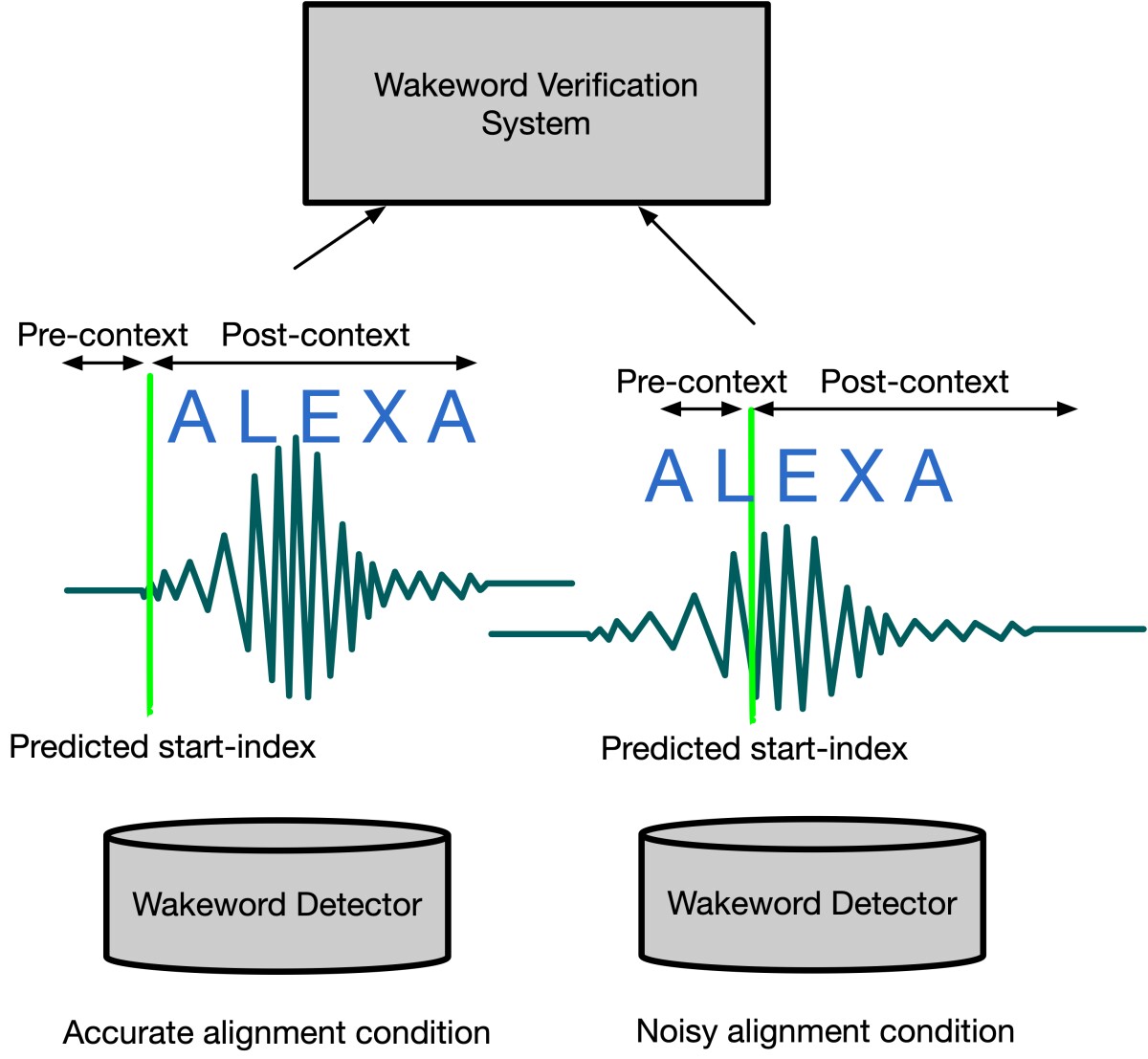
When CNNs are trained on well-aligned data, convolutional-layer outputs that focus on particular regions of the input can become biased toward finding wake word features in those regions. This can result in weaker performance when the alignment is noisy.
In “Building a robust word-level wakeword verification network”, applied scientist Rajath Kumar and his colleagues address this problem by adding recurrent layers to their network, to process the outputs of the convolutional layers. Recurrent layers can capture information as time sequences. Instead of learning where the wake word occurs in the input, the recurrent layers learn how the sequence changes temporally when the wake word is present.
This allows the researchers to train their network on well-aligned data without suffering much performance drop off on noisy data. To further improve performance, the researchers also use an attention layer to process and re-weight the sequential outputs of the recurrent layers, to emphasize the outputs required for wake word verification. The model is thus a convolutional-recurrent-attention (CRA) model.
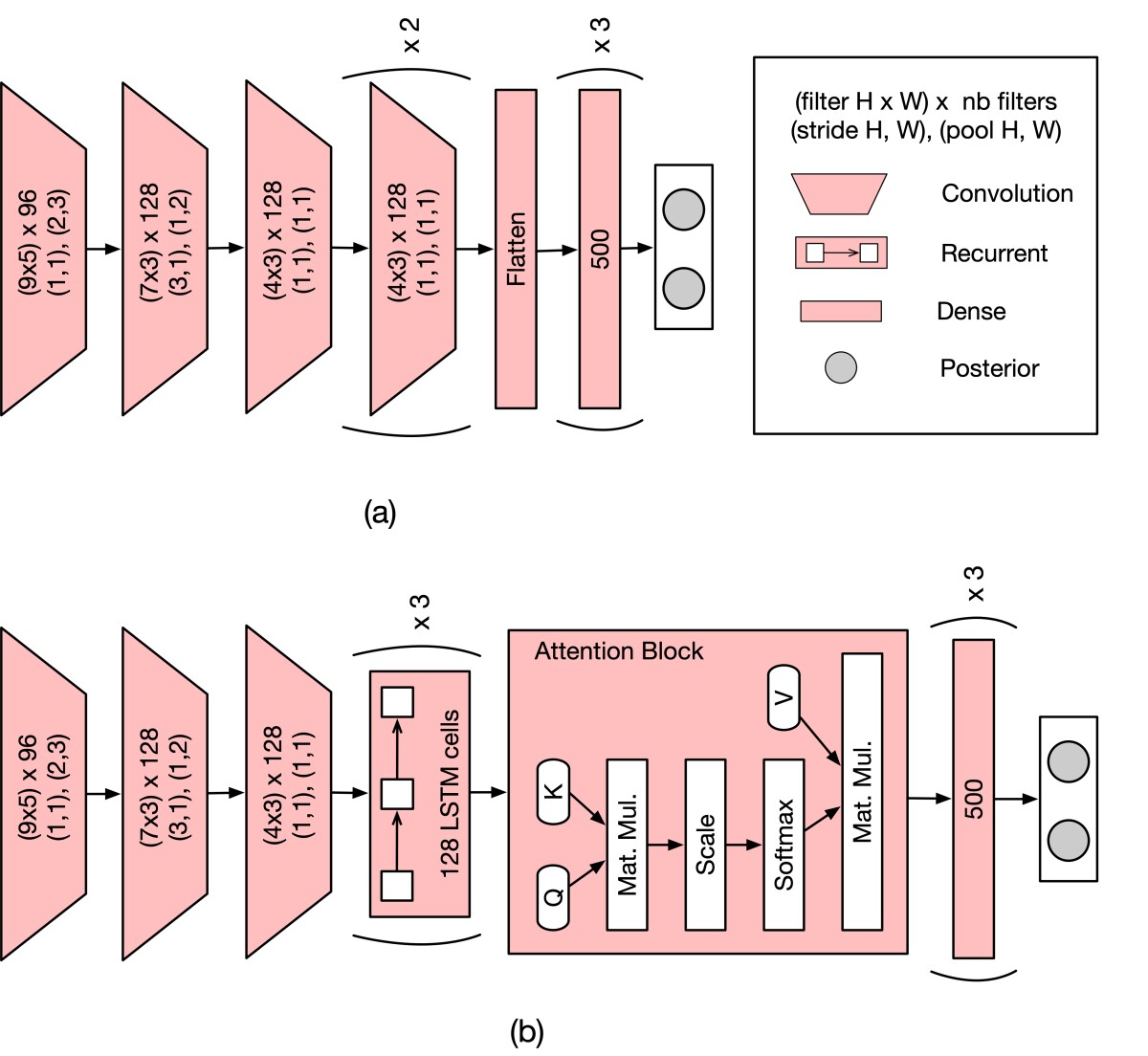
To evaluate their CRA model, the researchers compared its performance to that of several CNN-only models. Each example in the training data included 195 input frames, or sequential snapshots of the frequency spectrum. Within that 195-frame span, two of the CNN models looked at sliding windows of 76 frames or 100 frames. A third CNN model, and the CRA model, looked at all 195 frames. The models’ performance was assessed relative to a baseline wake word detector that combines a deep neural network with a hidden Markov model, an architecture was the industry standard for some time.
On accurately aligned inputs, the CRA model offers only a slight improvement over the 195-frame CNN model. Compared to the baseline, the CNN model reduced the false-acceptance rate by 53%, while the CRA reduced it by 55%. On the same task, the 100-frame CNN model achieved only a 35% reduction.
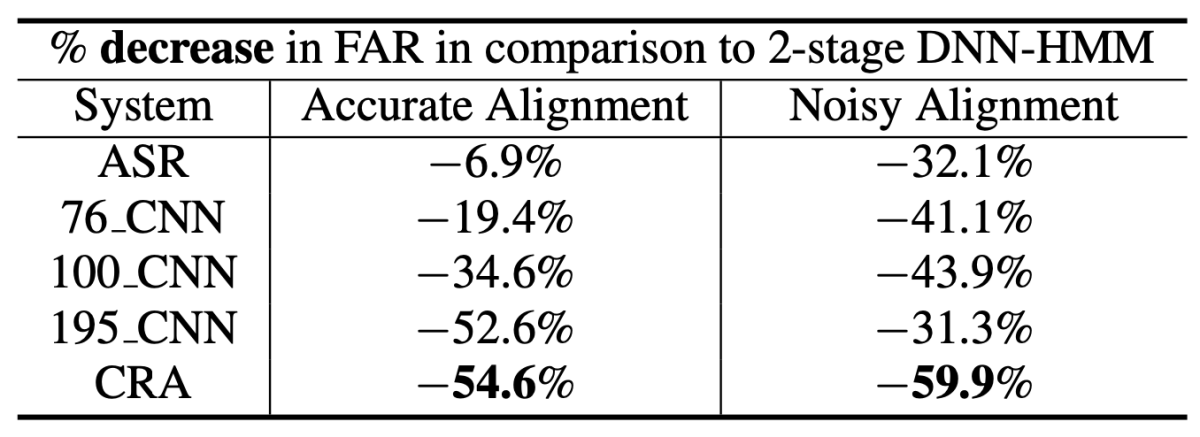
On noisily aligned inputs, the CRA model offered a much more dramatic improvement. Relative to baseline, it reduced the false-acceptance rate by 60%. The 195-frame CNN model managed only 31%, the 100-frame model 44%.