Amazon’s papers at the International Conference on Machine Learning (ICML) lean — like the conference as a whole — toward the theoretical. Although some papers deal with applications important to Amazon, such as anomaly detection and automatic speech recognition, most concern more-general topics related to machine learning, such as responsible AI and transfer learning. Learning algorithms, reinforcement learning, and privacy emerge as areas of particular interest.
Active learning
Understanding the training speedup from sampling with approximate losses
Rudrajit Das, Xi Chen, Bertram Ieong, Parikshit Bansal, Sujay Sanghavi
Anomaly detection
Online adaptive anomaly thresholding with confidence sequences
Sophia Sun, Abishek Sankararaman, Balakrishnan (Murali) Narayanaswamy
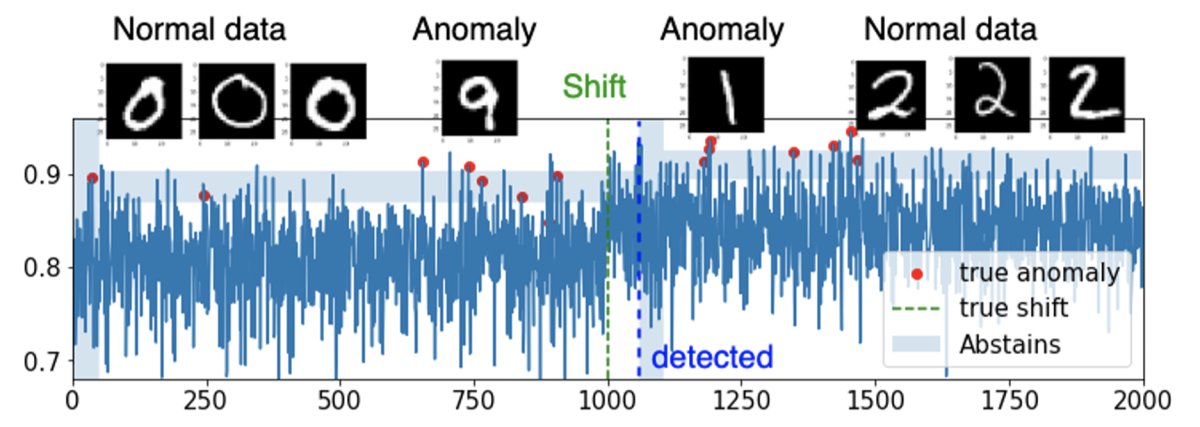
Automatic speech recognition
An efficient self-learning framework for interactive spoken dialog systems
Hitesh Tulsiani, David M. Chan, Shalini Ghosh, Garima Lalwani, Prabhat Pandey, Ankish Bansal, Sri Garimella, Ariya Rastrow, Björn Hoffmeister
Causal inference
Multiply-robust causal change attribution
Victor Quintas, Taha Bahadori, Eduardo Santiago, Jeff Mu, Dominik Janzing, David E. Heckerman
Code completion
REPOFORMER: Selective retrieval for repository-level code completion
Di Wu, Wasi Ahmad, Dejiao Zhang, Murali Krishna Ramanathan, Xiaofei Ma
Continual learning
MemoryLLM: Towards self-updatable large language models
Yu Wang, Yifan Gao, Xiusi Chen, Haoming Jiang, Shiyang Li, Jingfeng Yang, Qingyu Yin, Zheng Li, Xian Li, Bing Yin, Jingbo Shang, Julian McAuley
Contrastive learning
EMC2: Efficient MCMC negative sampling for contrastive learning with global convergence
Chung Yiu Yau, Hoi-To Wai, Parameswaran Raman, Soumajyoti Sarkar, Mingyi Hong
Data preparation
Fewer truncations improve language modeling
Hantian Ding, Zijian Wang, Giovanni Paolini, Varun Kumar, Anoop Deoras, Dan Roth, Stefano Soatto
Explainable AI
Explaining probabilistic models with distributional values
Luca Franceschi, Michele Donini, Cédric Archambeau, Matthias Seeger
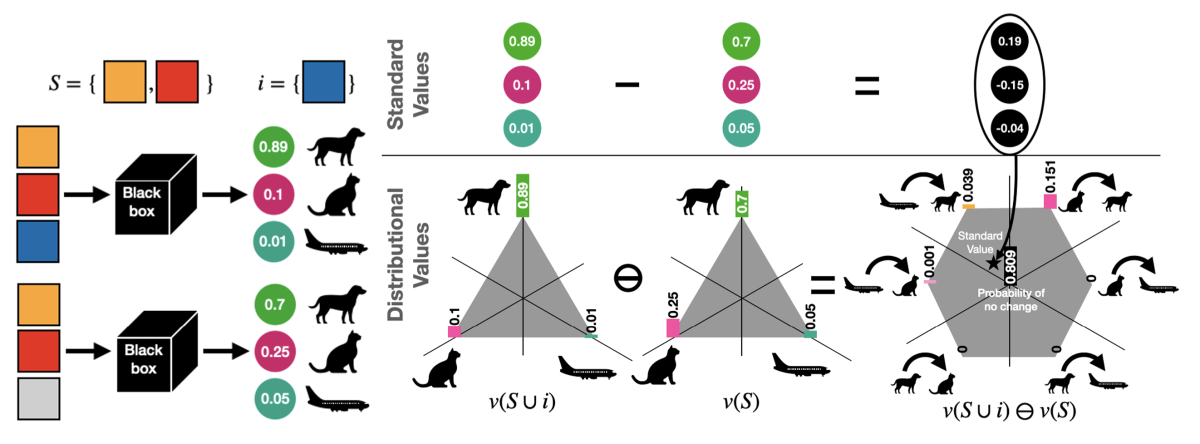
Hallucination mitigation
Multicalibration for confidence scoring in LLMs
Gianluca Detommaso, Martin Bertran Lopez, Riccardo Fogliato, Aaron Roth
Learning algorithms
MADA: Meta-adaptive optimizers through hyper-gradient descent
Kaan Ozkara, Can Karakus, Parameswaran Raman, Mingyi Hong, Shoham Sabach, Branislav Kveton, Volkan Cevher
Variance-reduced zeroth-order methods for fine-tuning language models
Tanmay Gautam, Youngsuk Park, Hao Zhou, Parameswaran Raman, Wooseok Ha
LLM decoding
Bifurcated attention for single-context large-batch sampling
Ben Athiwaratkun, Sujan Gonugondla, Sanjay Krishna Gouda, Hantian Ding, Qing Sun, Jun Wang, Jiacheng Guo, Liangfu Chen, Haifeng Qian, Parminder Bhatia, Ramesh Nallapati, Sudipta Sengupta, Bing Xiang
Model compression
COLLAGE: Light-weight low-precision strategy for LLM training
Tao Yu, Gaurav Gupta, Karthick Gopalswamy, Amith Mamidala, Hao Zhou, Jeffrey Huynh, Youngsuk Park, Ron Diamant, Anoop Deoras, Luke Huan
Privacy
Differentially private bias-term fine-tuning of foundation models
Zhiqi Bu, Yu-Xiang Wang, Sheng Zha, George Karypis
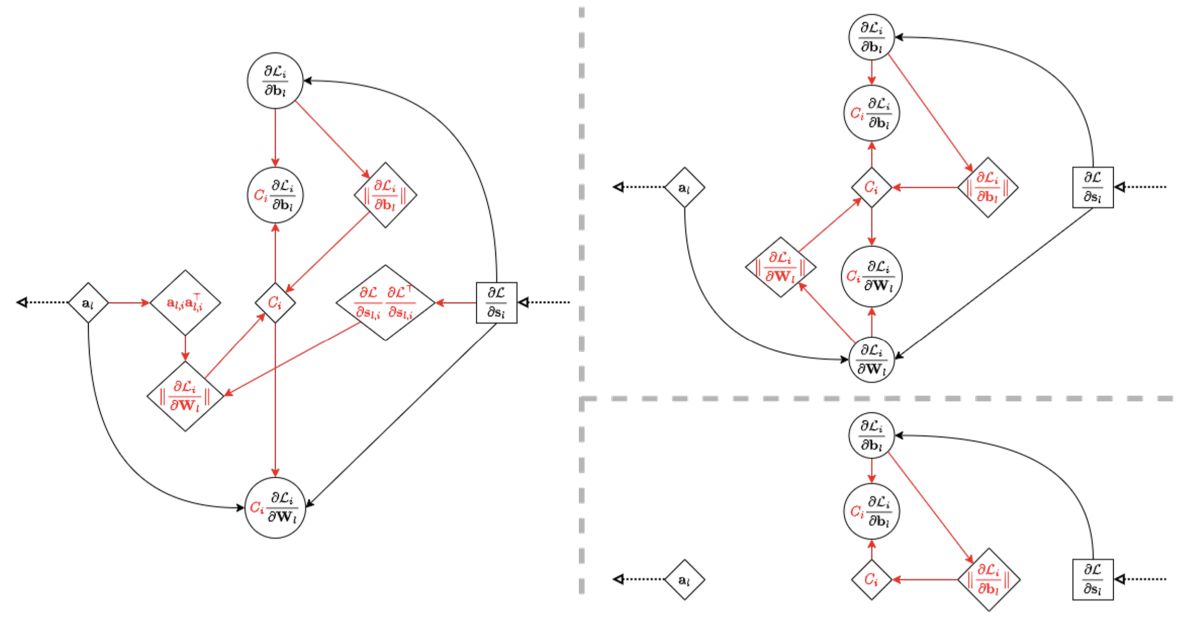
Membership inference attacks on diffusion models via quantile regression
Shuai Tang, Zhiwei Steven Wu, Sergul Aydore, Michael Kearns, Aaron Roth
Reinforcement learning
Finite-time convergence and sample complexity of actor-critic multi-objective reinforcement learning
Tianchen Zhou, Fnu Hairi, Haibo Yang, Jia (Kevin) Liu, Tian Tong, Fan Yang, Michinari Momma, Yan Gao
Learning the target network in function space
Kavosh Asadi, Yao Liu, Shoham Sabach, Ming Yin, Rasool Fakoor
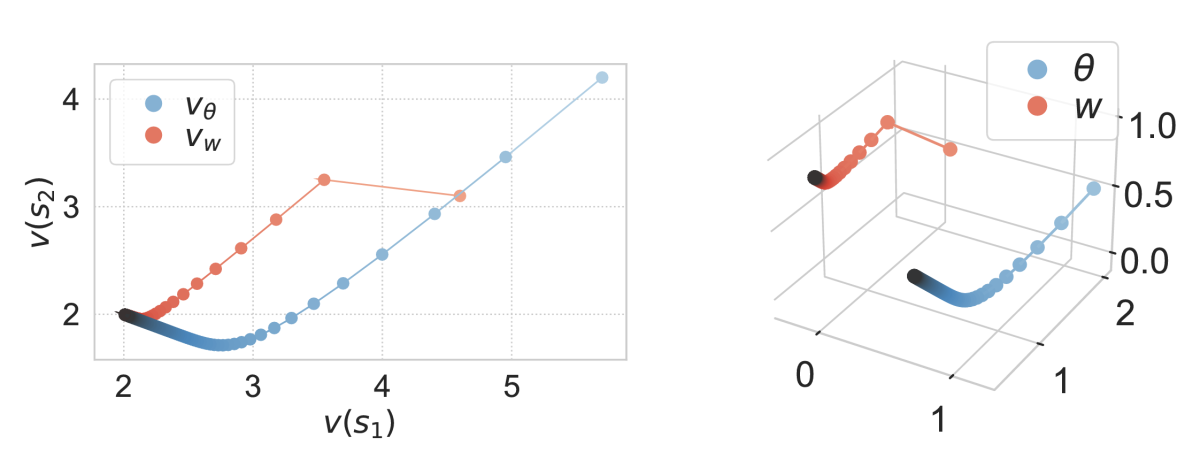
Near-optimal regret in linear MDPs with aggregate bandit feedback
Asaf Cassel, Haipeng Luo, Dmitry Sotnikov, Aviv Rosenberg
Responsible AI
Discovering bias in latent space: An unsupervised debiasing approach
Dyah Adila, Shuai Zhang, Boran Han, Bernie Wang
Retrieval-augmented generation
Automated evaluation of retrieval-augmented language models with task-specific exam generation
Gauthier Guinet, Behrooz Omidvar-Tehrani, Anoop Deoras, Laurent Callot
Robust learning
Robust multi-task learning with excess risks
Yifei He, Shiji Zhou, Guojun Zhang, Hyokun Yun, Yi Xu, Belinda Zeng, Trishul Chilimbi, Han Zhao
Scientific machine learning
Using uncertainty quantification to characterize and improve out-of-domain learning for PDEs
S. Chandra Mouli, Danielle Maddix Robinson, Shima Alizadeh, Gaurav Gupta, Andrew Stuart, Michael Mahoney, Bernie Wang
Transfer learning
Transferring knowledge from large foundation models to small downstream models
Shikai Qiu, Boran Han, Danielle Maddix Robinson, Shuai Zhang, Bernie Wang, Andrew Wilson